Pathology AI Breakthrough: Train SOTA Models With 1000x Less Data
This is a Plain English Papers summary of a research paper called Pathology AI Breakthrough: Train SOTA Models With 1000x Less Data. If you like these kinds of analysis, you should join AImodels.fyi or follow us on Twitter. Overview Researchers trained pathology foundation models using only 70,000 patches Achieved performance comparable to models trained on 80 million patches Used contrastive learning and medical imaging transformers Results suggest smaller, more diverse datasets are more efficient Method enables high-quality models with much less computational resources Plain English Explanation Medical AI has a data problem. To build good models for analyzing medical images like tissue slides, researchers typically need enormous datasets - often tens of millions of image patches. This creates significant barriers: gathering and storing that much medical data is diffic... Click here to read the full summary of this paper
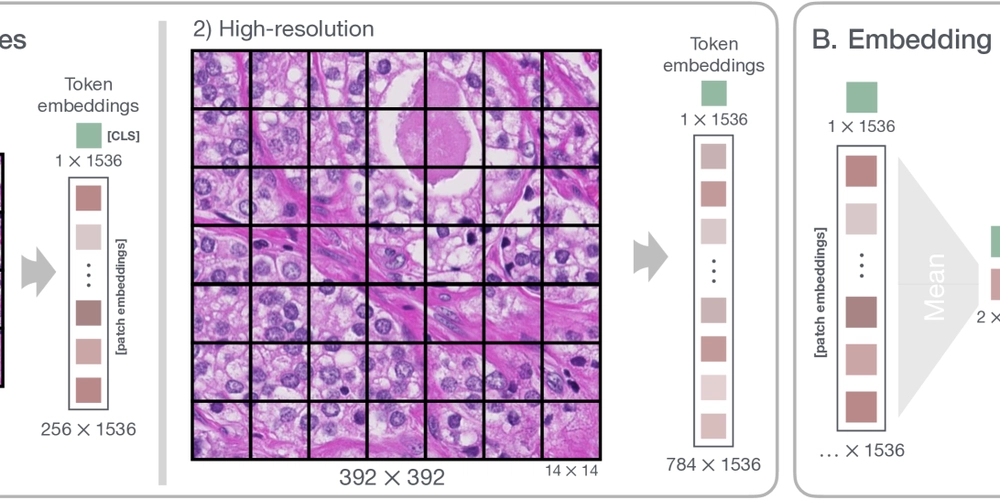
This is a Plain English Papers summary of a research paper called Pathology AI Breakthrough: Train SOTA Models With 1000x Less Data. If you like these kinds of analysis, you should join AImodels.fyi or follow us on Twitter.
Overview
- Researchers trained pathology foundation models using only 70,000 patches
- Achieved performance comparable to models trained on 80 million patches
- Used contrastive learning and medical imaging transformers
- Results suggest smaller, more diverse datasets are more efficient
- Method enables high-quality models with much less computational resources
Plain English Explanation
Medical AI has a data problem. To build good models for analyzing medical images like tissue slides, researchers typically need enormous datasets - often tens of millions of image patches. This creates significant barriers: gathering and storing that much medical data is diffic...