Building an Efficient and Cost-Effective Business Data Analytics System with Databend Cloud
With the rise of large AI models such as OpenAI's ChatGPT, DeepL, and Gemini, the traditional machine translation field is being disrupted. Unlike earlier tools that often produced rigid translations lacking contextual understanding, these new models can accurately capture linguistic nuances and context, adjusting wording in real-time to deliver more natural and fluent translations. As a result, more users are turning to these intelligent tools, making cross-language communication more efficient and human-like. Recently, a highly popular bilingual translation extension has gained widespread attention. This tool allows users to instantly translate foreign language web pages, PDF documents, ePub eBooks, and subtitles. It not only provides real-time bilingual display of both the original text and translation but also supports custom settings for dozens of translation platforms, including Google, OpenAI, DeepL, Gemini, and Claude. It has received overwhelmingly positive reviews online. As the user base continues to grow, the operations and product teams aim to leverage business data to support growth strategy decisions while ensuring user privacy is respected. Business Challenges Business event tracking metrics are one of the essential data sources in a data warehouse and among a company's most valuable assets. Typically, business data analytics rely on two major data sources: business analytics logs and upstream relational databases (such as MySQL). By leveraging these data sources, companies can conduct user growth analysis, business performance research, and even precisely troubleshoot user issues through business data analytics. The nature of business data analytics makes it challenging to build a scalable, flexible, and cost-effective analytics architecture. The key challenges include: High Traffic and Large Volume: Business data is generated in massive quantities, requiring robust storage and analytical capabilities. Diverse Analytical Needs: The system must support both static BI reporting and flexible ad-hoc queries. Varied Data Formats: Business data often includes both structured and semi-structured formats (e.g., JSON). Real-Time Requirements: Fast response times are essential to ensure timely feedback on business data. Due to these complexities, the tool’s technical team initially chose a general event tracking system for business data analytics. This system allows data to be automatically collected and uploaded by simply inserting JSON code into a website or embedding an SDK in an app, generating key metrics such as page views, session duration, and conversion funnels. However, while general event tracking systems are simple and easy to use, they also come with several limitations in practice: Lack of Detailed Data: These systems often do not provide detailed user visit logs and only allow querying predefined reports through the UI. Limited Custom Query Capabilities: Since general tracking systems do not offer a standard SQL query interface, data scientists struggle to perform complex ad-hoc queries due to the lack of SQL support. Rapidly Increasing Costs: These systems typically use a tiered pricing model, where costs double once a new usage tier is reached. As business traffic grows, querying a larger dataset can lead to significant cost increases. Additionally, the team follows the principle of minimal data collection, avoiding the collection of potentially identifiable data, specific user behavior details, and focusing only on necessary statistical data rather than personalized data, such as translation time, translation count, and errors or exceptions. Under these constraints, most third-party data collection services were discarded. Given that the tool serves a global user base, it is essential to respect data usage and storage rights across different regions and avoid cross-border data transfers. Considering these factors, the team must exercise fine-grained control over data collection and storage methods, making building an in-house business data system the only viable option. The Complexity of Building an In-House Business Data Analytics System To address the limitations of the generic tracking system, the translation tool decided to build its own business data analysis system after the business reached a certain stage of growth. After conducting research, the technical team found that traditional self-built architectures are mostly based on the Hadoop big data ecosystem. A typical implementation process is as follows: Embed SDK in the client (APP, website) to collect business data logs (activity logs); Use an Activity gateway for tracking metrics, collect the logs sent by the client, and transfer the logs to a Kafka message bus; Use Kafka to load the logs into computation engines like Hive or Spark; Use ETL tools to import the data into a data warehouse and generate business data analysis reports. Although this architecture can meet the funct
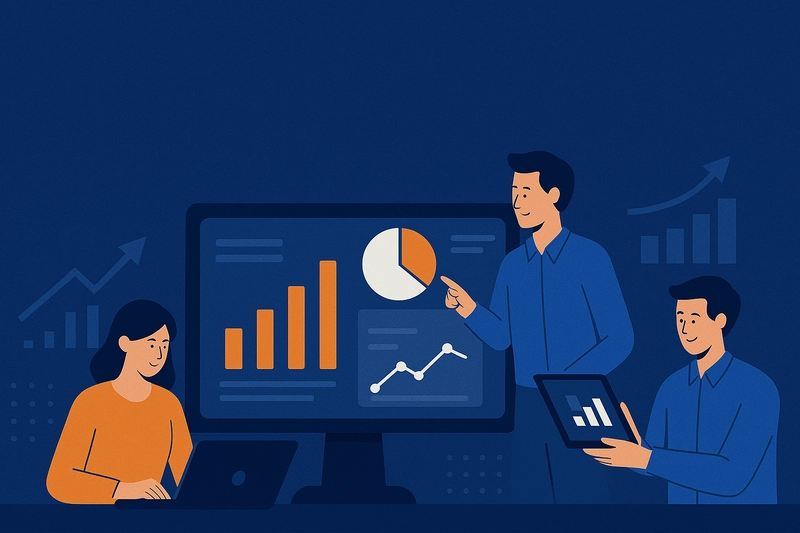
With the rise of large AI models such as OpenAI's ChatGPT, DeepL, and Gemini, the traditional machine translation field is being disrupted. Unlike earlier tools that often produced rigid translations lacking contextual understanding, these new models can accurately capture linguistic nuances and context, adjusting wording in real-time to deliver more natural and fluent translations. As a result, more users are turning to these intelligent tools, making cross-language communication more efficient and human-like.
Recently, a highly popular bilingual translation extension has gained widespread attention. This tool allows users to instantly translate foreign language web pages, PDF documents, ePub eBooks, and subtitles. It not only provides real-time bilingual display of both the original text and translation but also supports custom settings for dozens of translation platforms, including Google, OpenAI, DeepL, Gemini, and Claude. It has received overwhelmingly positive reviews online.
As the user base continues to grow, the operations and product teams aim to leverage business data to support growth strategy decisions while ensuring user privacy is respected.
Business Challenges
Business event tracking metrics are one of the essential data sources in a data warehouse and among a company's most valuable assets. Typically, business data analytics rely on two major data sources: business analytics logs and upstream relational databases (such as MySQL). By leveraging these data sources, companies can conduct user growth analysis, business performance research, and even precisely troubleshoot user issues through business data analytics.
The nature of business data analytics makes it challenging to build a scalable, flexible, and cost-effective analytics architecture. The key challenges include:
- High Traffic and Large Volume: Business data is generated in massive quantities, requiring robust storage and analytical capabilities.
- Diverse Analytical Needs: The system must support both static BI reporting and flexible ad-hoc queries.
- Varied Data Formats: Business data often includes both structured and semi-structured formats (e.g., JSON).
- Real-Time Requirements: Fast response times are essential to ensure timely feedback on business data.
Due to these complexities, the tool’s technical team initially chose a general event tracking system for business data analytics. This system allows data to be automatically collected and uploaded by simply inserting JSON code into a website or embedding an SDK in an app, generating key metrics such as page views, session duration, and conversion funnels.
However, while general event tracking systems are simple and easy to use, they also come with several limitations in practice:
- Lack of Detailed Data: These systems often do not provide detailed user visit logs and only allow querying predefined reports through the UI.
- Limited Custom Query Capabilities: Since general tracking systems do not offer a standard SQL query interface, data scientists struggle to perform complex ad-hoc queries due to the lack of SQL support.
- Rapidly Increasing Costs: These systems typically use a tiered pricing model, where costs double once a new usage tier is reached. As business traffic grows, querying a larger dataset can lead to significant cost increases.
Additionally, the team follows the principle of minimal data collection, avoiding the collection of potentially identifiable data, specific user behavior details, and focusing only on necessary statistical data rather than personalized data, such as translation time, translation count, and errors or exceptions. Under these constraints, most third-party data collection services were discarded. Given that the tool serves a global user base, it is essential to respect data usage and storage rights across different regions and avoid cross-border data transfers. Considering these factors, the team must exercise fine-grained control over data collection and storage methods, making building an in-house business data system the only viable option.
The Complexity of Building an In-House Business Data Analytics System
To address the limitations of the generic tracking system, the translation tool decided to build its own business data analysis system after the business reached a certain stage of growth. After conducting research, the technical team found that traditional self-built architectures are mostly based on the Hadoop big data ecosystem. A typical implementation process is as follows:
- Embed SDK in the client (APP, website) to collect business data logs (activity logs);
- Use an Activity gateway for tracking metrics, collect the logs sent by the client, and transfer the logs to a Kafka message bus;
- Use Kafka to load the logs into computation engines like Hive or Spark;
- Use ETL tools to import the data into a data warehouse and generate business data analysis reports.
Although this architecture can meet the functional requirements, its complexity and maintenance costs are extremely high:
- Kafka relies on Zookeeper and requires SSD drives to ensure performance.
- Kafka to Data Warehouse requires kafka-connect.
- Spark needs to run on YARN, and ETL processes need to be managed by Airflow.
- When Hive storage reaches its limit, it may be necessary to replace MySQL with distributed databases like TiDB.
This architecture not only requires a large investment of technical team resources but also significantly increases the operational maintenance burden. In the current context where businesses are constantly striving for cost reduction and efficiency improvement, this architecture is no longer suitable for business scenarios that require simplicity and high efficiency.
Why Databend Cloud?
The technical team chose Databend Cloud for building the business data analysis system due to its simple architecture and flexibility, offering an efficient and low-cost solution:
- 100% object storage-based, with full separation of storage and computation, significantly reducing storage costs.
- The query engine, written in Rust, offers high performance at a low cost. It automatically hibernates when computational resources are idle, preventing unnecessary expenses.
- Fully supports 100% ANSI SQL and allows for semi-structured data analysis (JSON and custom UDFs). When users have complex JSON data, they can leverage the built-in JSON analysis capabilities or custom UDFs to analyze semi-structured data.
- Built-in task scheduling drives ETL, fully stateless, with automatic elastic scaling.
After adopting Databend Cloud, they abandoned Kafka and instead used Databend Cloud to create stages, importing business logs into S3 and then using tasks to bring them into Databend Cloud for data processing.
- Log collection and storage: Kafka is no longer required. The tracking logs are directly stored in S3 in NDJSON format via vector.
- Data ingestion and processing: A copy task is created within Databend Cloud to automatically pull the logs from S3. In many cases, S3 can act as a stage in Databend Cloud. Data within this stage can be automatically ingested by Databend Cloud, processed there, and then exported back from S3.
- Query and report analysis: BI reports and ad-hoc queries are run via a warehouse that automatically enters sleep mode, ensuring no costs are incurred while idle.
Databend, as an international company with an engineering-driven culture, has earned the trust of the technical team through its contributions to the open-source community and its reputation for respecting and protecting customer data. Databend's services are available globally, and if the team has future needs for global data analysis, the architecture is easy to migrate and scale.
Through the approach outlined above, Databend Cloud enables enterprises to meet their needs for efficient business data analysis in the simplest possible way.
Solution
The preparation required to build such a business data analysis architecture is very simple. First, prepare two Warehouses: one for Task-based data ingestion and the other for BI report queries. The ingestion Warehouse can be of a smaller specification, while the query Warehouse should be of a higher specification, as queries typically don't run continuously. This helps save more costs.
Then, click Connect to obtain a connection string, which can be used in BI reports for querying. Databend provides drivers for various programming languages.
The next preparation steps are simple and can be completed in three steps:
- Create a table with fields that match the NDJSON format of the logs.
- Create a stage, linking the S3 directory where the business data logs are stored.
- Create a task that runs every minute or every ten seconds. It will automatically import the files from the stage and then clean them up.
Vector configuration:
[sources.input_logs]
type = "file"
include = ["/path/to/your/logs/*.log"]
read_from = "beginning"
[transforms.parse_ndjson]
type = "remap"
inputs = ["input_logs"]
source = '''
. = parse_json!(string!(.message))
'''
[sinks.s3_output]
type = "aws_s3"
inputs = ["parse_ndjson"]
bucket = "${YOUR_BUCKET_NAME}"
region = "%{YOUR_BUCKET_REGION}"
encoding.codec = "json"
key_prefix = "logs/%Y/%m/%d"
compression = "none"
batch.max_bytes = 10485760 # 10MB
batch.timeout_secs = 300 # 5 minutes
aws_access_key_id = "${AWS_ACCESS_KEY_ID}"
aws_secret_access_key = "${AWS_SECRET_ACCESS_KEY}"
Once the preparation work is complete, you can continuously import business data logs into Databend Cloud for analysis.
Architecture Comparisons & Benefits
By comparing the generic tracking system, traditional Hadoop architecture, and Databend Cloud, Databend Cloud has significant advantages:
- Architectural Simplicity: It eliminates the need for complex big data ecosystems, without requiring components like Kafka, Airflow, etc.
- Cost Optimization: Utilizes object storage and elastic computing to achieve low-cost storage and analysis.
- Flexibility and Performance: Supports high-performance SQL queries to meet diverse business scenarios.
In addition, Databend Cloud provides a snapshot mechanism that supports time travel, allowing for point-in-time data recovery, which helps ensure data security and recoverability for "immersive translation."
Ultimately, the technical team of the translation tool completed the entire POC test in just one afternoon, switching from the complex Hadoop architecture to Databend Cloud, greatly simplifying operational and maintenance costs.
When building a business data tracking system, in addition to storage and computing costs, maintenance costs are also an important factor in architecture selection. Through its innovation of separating object storage and computing, Databend has completely transformed the complexity of traditional business data analysis systems. Enterprises can easily build a high-performance, low-cost business data analysis architecture, achieving full-process optimization from data collection to analysis. This not only reduces costs and improves efficiency but also unlocks the maximum value of data.