AI in Diagnosis & Detection
Introduction An artificial Intelligence program has recently achieved an area under the curve (AUC) of 0.94 and markedly decreased the amount of false positives and false negatives, surpassing six radiologists in lung cancer detection by CT scans [1] [2] [3]. This advanced precision constitutes technological innovations—and it’s the same drive that fuels the creation of communities like NeuraVia, which unites passionate researchers in the fields of neuroscience and AI to collaborate in creating early diagnostic tools for neurological disorders—and a valuable instrument for addressing one of healthcare’s most enduring issues: misdiagnosis. Every year, about 12 million US adults are misdiagnosed, leading to disabilities and deaths in 800,000 people [4]. The prevalence of diagnostic inaccuracy continues to affect patient outcomes and trust, AI presents a promising way to improve speed, accuracy, and accessibility in healthcare diagnostics. This article delves into the transformative future AI poses for disease detection and diagnosis, whether good or bad. How AI Works in Diagnosis When looking at AI usages for medical diagnosis, it consists of technological systems that imitate the knowledge of human systems to assist clinicians for more accurate diagnosis by evaluating intricate health data [5]. Fundamental to AI in machine learning (ML), a subset in which algorithms are trained to forecast outcomes by analyzing enormous amounts of medical information independently (without external human programming) [6] [8]. Much like humans, when exposed to many examples or data, these systems keep improving in accuracy, simulating a form of computational “intuition,” as it feels intuitive from deep pattern recognition [7]. Three primary tool sets enable AI diagnosis: Image recognition: To identify disease patterns, abnormalities, and early changes that cannot be seen with the human eye, AI proficiently analyzes radiological scans (X–rays, CT scans, and MRIs), dermatological photographs, and pathology slides [6] [8]. Natural language processing (NLP): With the use of this technology, AI can effectively extract clinical insights by comprehending unstructured data from patient records, clinical notes, and electronic health records (EHRs) [6] [8]. Predictive analytics: Using EHRs, AI is able to determine risk scores, detect disease onset and progression, and help identify individuals with higher risks earlier [6] [8]. Forming these bases involves exposure to thorough and greater training diversities with multiple datasets, allowing for reliable pattern identification among diverse groups [6][7]. This allows the models to “learn” through captioned data—like labeled images with confirmed diagnosis and patient records with known outcomes—helping it improve its precision and find significant correlations [6]. Real-World Applications Radiology In radiology, AI has expanded significantly as they are now consistently analyzing data from mammograms, chest X-rays, and MRIs, helping detect diseases such as breast cancer, lung cancer, and brain disorders more quickly and accurately [9]. For example, Google Health's breast cancer AI has reduced the amount of recorded false positives, the workload, and has outdone human radiologists in detected cancers [9]. MRI systems with AI enhancements are also well-versed in identifying brain abnormalities, which enable faster diagnosis of neurological disorders compared to conventional techniques. Dermatology Similarly, AI allows for faster detection of skin cancer. Clinical validation and accuracy rates have now exceeded 90% by allowing users to take a picture of their skin lesions and get generated risk evaluations from apps such as SkinVision and platforms such as Skin Image Search™ [10] [11]. This prompts people to develop successful routines and have helped dermatologists by providing rapid patient evaluation. Pathology AI in pathology has surpassed traditional microscopic examinations in terms of speed and accuracy from analyzing whole biopsy slides [12]. With 99% accuracy rates, AI has successfully detected metastatic breast cancer in lymph nodes and prostate, bone marrow, and colorectal cancer diagnosis [12] [13]. AI has also supported generating treatment plans by helping pathologists detect both cancerous and precancerous changes earlier. Ophthalmology In ophthalmology, AI is commonly used to screen diabetic retinopathy (DR), especially within underserved and rural areas [14]. Also with the use of mobiles, retinal fundus images can be generated when scanned which are helpful when specialists are absent [14]. The use of AI has significantly improved early detection, expanded screening coverage, closed the accessibility gap, and are more affordable than standard methods [14]. NeuraVia NeuraVia is an expanding project dedicated to bringing enthusiastic academics, students, and researchers to excel at working on a nexus on AI and neuroscience. It aims to speed up early detec
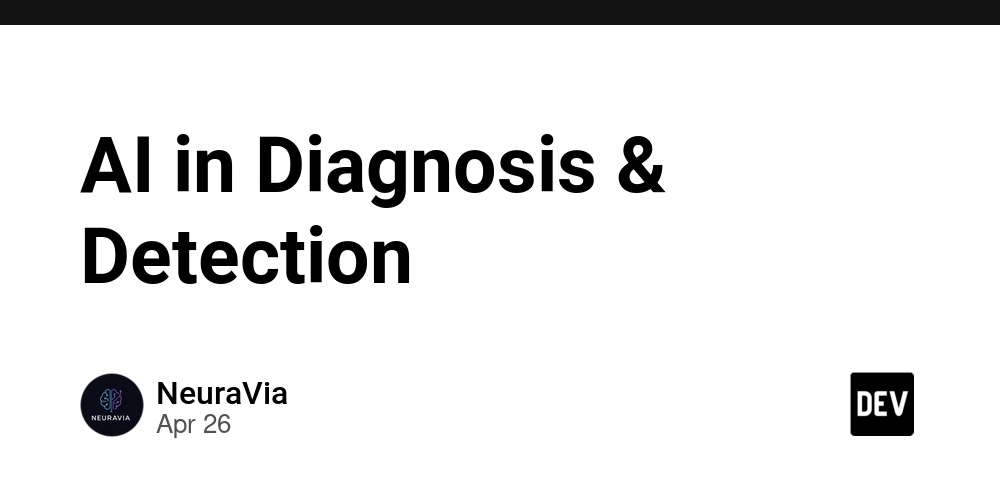
Introduction
An artificial Intelligence program has recently achieved an area under the curve (AUC) of 0.94 and markedly decreased the amount of false positives and false negatives, surpassing six radiologists in lung cancer detection by CT scans [1] [2] [3]. This advanced precision constitutes technological innovations—and it’s the same drive that fuels the creation of communities like NeuraVia, which unites passionate researchers in the fields of neuroscience and AI to collaborate in creating early diagnostic tools for neurological disorders—and a valuable instrument for addressing one of healthcare’s most enduring issues: misdiagnosis. Every year, about 12 million US adults are misdiagnosed, leading to disabilities and deaths in 800,000 people [4]. The prevalence of diagnostic inaccuracy continues to affect patient outcomes and trust, AI presents a promising way to improve speed, accuracy, and accessibility in healthcare diagnostics. This article delves into the transformative future AI poses for disease detection and diagnosis, whether good or bad.
How AI Works in Diagnosis
When looking at AI usages for medical diagnosis, it consists of technological systems that imitate the knowledge of human systems to assist clinicians for more accurate diagnosis by evaluating intricate health data [5]. Fundamental to AI in machine learning (ML), a subset in which algorithms are trained to forecast outcomes by analyzing enormous amounts of medical information independently (without external human programming) [6] [8]. Much like humans, when exposed to many examples or data, these systems keep improving in accuracy, simulating a form of computational “intuition,” as it feels intuitive from deep pattern recognition [7].
Three primary tool sets enable AI diagnosis:
Image recognition: To identify disease patterns, abnormalities, and early changes that cannot be seen with the human eye, AI proficiently analyzes radiological scans (X–rays, CT scans, and MRIs), dermatological photographs, and pathology slides [6] [8].
Natural language processing (NLP): With the use of this technology, AI can effectively extract clinical insights by comprehending unstructured data from patient records, clinical notes, and electronic health records (EHRs) [6] [8].
Predictive analytics: Using EHRs, AI is able to determine risk scores, detect disease onset and progression, and help identify individuals with higher risks earlier [6] [8].
Forming these bases involves exposure to thorough and greater training diversities with multiple datasets, allowing for reliable pattern identification among diverse groups [6][7]. This allows the models to “learn” through captioned data—like labeled images with confirmed diagnosis and patient records with known outcomes—helping it improve its precision and find significant correlations [6].
Real-World Applications
Radiology
In radiology, AI has expanded significantly as they are now consistently analyzing data from mammograms, chest X-rays, and MRIs, helping detect diseases such as breast cancer, lung cancer, and brain disorders more quickly and accurately [9]. For example, Google Health's breast cancer AI has reduced the amount of recorded false positives, the workload, and has outdone human radiologists in detected cancers [9]. MRI systems with AI enhancements are also well-versed in identifying brain abnormalities, which enable faster diagnosis of neurological disorders compared to conventional techniques.
Dermatology
Similarly, AI allows for faster detection of skin cancer. Clinical validation and accuracy rates have now exceeded 90% by allowing users to take a picture of their skin lesions and get generated risk evaluations from apps such as SkinVision and platforms such as Skin Image Search™ [10] [11]. This prompts people to develop successful routines and have helped dermatologists by providing rapid patient evaluation.
Pathology
AI in pathology has surpassed traditional microscopic examinations in terms of speed and accuracy from analyzing whole biopsy slides [12]. With 99% accuracy rates, AI has successfully detected metastatic breast cancer in lymph nodes and prostate, bone marrow, and colorectal cancer diagnosis [12] [13]. AI has also supported generating treatment plans by helping pathologists detect both cancerous and precancerous changes earlier.
Ophthalmology
In ophthalmology, AI is commonly used to screen diabetic retinopathy (DR), especially within underserved and rural areas [14]. Also with the use of mobiles, retinal fundus images can be generated when scanned which are helpful when specialists are absent [14]. The use of AI has significantly improved early detection, expanded screening coverage, closed the accessibility gap, and are more affordable than standard methods [14].
NeuraVia
NeuraVia is an expanding project dedicated to bringing enthusiastic academics, students, and researchers to excel at working on a nexus on AI and neuroscience. It aims to speed up early detection, treatment, and provide education for neurological disorders, beginning with Alzheimer’s. It welcomes both creativity and cooperation to give the youth a chance to revolutionize the future by creating significant AI tools. This effort holds promise for a larger movement to use AI tools to advance in already advancing intelligence, compassion, and healthcare.
Benefits of AI Diagnosis
Increased Accuracy
Implying AI in regular practices ensures increased consistency through reducing human error, and even through outperforming clinicians on accurate diagnosis. As seen, in radiology for breast cancer, AI has reduced the amount of false positives and false negatives, enhancing skill it has towards earlier cancer detection [15]. In pathology and dermatology, AI can occasionally perform at the same level or even better than specialists in that field [12].
Faster Results and Quicker Treatment
Just by a click of a button, AI can generate the correct protocols to follow after careful and fast analysis, speeding up the diagnostic process. This rapid diagnosis of prompt treatment decisions is incredibly important in the setting of emergencies or critical care, where speed can potentially save a life.
Scalability and Accessibility
Because of its scalability, AI has revolutionized its technical diagnostics for availability to multiple regions, such as those that are underserved or remote. In areas with few health-care workers or with limited amounts of technology, AI-augmented telemedicine and mobile clinics can expand their professional diagnostic skills to close this differentiation [16].
24/7 Availability and Cost-Effectiveness
Once implemented, AI tools run consistently, available to be used 24/7 without the need for round-the-clock assistance and limitations. By streamlining continuous operations and automating workflows, expenses are lowered helping with cost savings and helping increase affordability and sustainability to maintain high-quality treatment [17].
Challenges and Limitations
Data Bias and Fairness
How well AI performs for giving fair and correct responses, depends on the data that they were trained on. If the data lacks specific groups, it can become biased with underdiagnosis and health inequalities increasing in those populations. To guarantee fair results, the dataset must have a strong validation, curation, and continual bias monitoring which may be tedious [18].
The Essential Role of Human Oversight
Even though AI can be seen as a shortcut, overly depending on these algorithms poses risks like loss of medical instinct, reduction in critical thinking, and risky acceptance of these forecasts because of overreliance. Human specialists obtain the ability to provide empathic care, awareness of context, and being able to identify uncommon or abnormal features [19].
Privacy and Ethical Concerns
In order to properly train the algorithms and correctly diagnose patients, AI systems require a considerable amount of private information which jeopardize the patient’s privacy, raising ethical concerns on consent and cybersecurity. If this information gets misused, after effects are to be expected. Other ethical issues are related to accountability, responsibility, and prejudice towards certain individuals [20].
Regulatory Hurdles
Before being presented into medical applications, regulations must be passed by agencies such as the FDA and EMA demanding strict oversight and validation for adhering to strict safety and effectiveness protocols. Monitoring of the product after it has been released requires constant review, and approval procedures are changing to handle the difficulties that adaptive AI presents [21].
The Future of AI in Diagnosis
Hybrid Models: Doctors + AI
The collaboration between AI’s computational power and human’s strengths in empathy, ethics and relevance paves the path for the most promising future of AI in diagnosis [22]. In actuality, this collaboration allows physicians to use AI as their “second option” or a confirmation tool, which will correct or enhance their clinical judgement [22]. When practiced together, this entails that patients receive an accurate and confident diagnosis, while still receiving trustful human advice at the core of the treatment [22].
When practiced as a “second option,” AI develops the “super assistant” role that extends beyond the need for just diagnosing, but to identify possible blinc spots or mistakes in complex situations that may have been missed, aiding with clinical decision-making, and synthesizing enormous medical databases [22]. These techniques improve the effectiveness and safety of clinicians without having to replace their accountability and knowledge.
Personalized Diagnosis with Genomics and AI
Thanks to the combination of AI and genetics, medicine is getting closer to creating effective personalized treatments, making highly personalized diagnostic and therapeutic approaches possible. By AI’s ability to analyze genomic, proteomic, and clinical data, personalized healthcare includes finding risk factors, disease subtypes, and treatment responses [23].
Building Trust and Transparency
In order for this collaboration to be utilized widely in the future, we need to maintain confidence and trust. These include explainable AI—careful explanation of algorithm operations—physician’s supervision, and the emphasis on ethical values. For maintaining transparency and strengthening physician trust and promoting acceptance, offering feature-highlighter or example-based explanations will be necessary [24].
Conclusion
Fortunately, AI is not employed to replace doctors, but to refine accuracy, provide them with more profound understanding, and smoother detection with diagnosing diseases. With the collaboration of both doctors with reassurance and empathy and AI algorithms with continuingly improving precision, the road to the most successful diagnosis has been unlocked. Our challenge is to ensure that with developing AI technology, this collaboration will provide care that is both intelligent and essentially more human. The future of diagnosis—where clinicians are now supported by AI algorithms—has already begun. Communities like NeuraVia stand at the forefront, empowered by curiosity and collaboration of related expertise that make AI diagnostics more intelligent—and more human.