Revolutionizing Beekeeping with AI-Powered Computer Vision
Beekeeping is an ancient practice that has long relied on honeybees for honey production and pollination. According to recent research by the Environment America Research & Policy Center, bees contribute to pollinating 73 of the 103 crops essential to global agriculture. Traditionally, beekeepers have monitored hive health and productivity through manual inspections, a process that is time-consuming and reliant on human observation. However, advancements in artificial intelligence (AI) and computer vision in beekeeping have paved the way for automated hive monitoring. Modern AI-driven technology can analyze hive health, detect diseases, classify species and pollen in real-time, and provide beekeepers with crucial insights. By leveraging AI-based monitoring, beekeepers can enhance colony health and improve pollination efficiency in agricultural ecosystems. This article explores how integrating computer vision into beekeeping can transform colony productivity and shape the future of hive management. Challenges in Modern Beekeeping Beekeepers face numerous challenges in maintaining colony health and productivity. Traditional monitoring techniques are inefficient, making it difficult to sustain thriving hives. 1. Detection of Hive Diseases Pests and bacterial infections can spread rapidly within a beehive, threatening the entire colony. Early detection of diseases such as the Varroa destructor infestation is crucial to maintaining a healthy hive. 2. Foraging Behavior Surveillance Tracking bee activity and identifying the flowers they visit helps optimize pollination. However, monitoring this behavior across vast areas without automation is challenging. 3. Tracking Bee Populations Accurate records of bees entering and exiting the hive provide insights into colony strength. Manual counting, however, is impractical. 4. Addressing Environmental Threats Climate change, habitat loss, and extreme temperature fluctuations pose significant risks to bee colonies. Monitoring these environmental conditions in real-time is essential to colony survival. To address these challenges, AI-powered real-time monitoring provides automated solutions that reduce the human workload while offering data-driven insights. Practical Applications of Computer Vision in Beekeeping AI-powered vision systems enable beekeepers to enhance hive monitoring and pollination tracking in various ways: 1. Early Disease Detection The Varroa destructor is a common parasite that weakens bee colonies and contributes to colony collapse disorder. AI-powered object detection systems can identify mites early, preventing widespread infestations. 2. Flower Detection & Classification By identifying high-value nectar sources, beekeepers can make informed decisions to support honey yield and colony health. AI models trained to classify flower species analyze images captured by drones, helping beekeepers adjust hive placement and maintain access to diverse floral resources. 3. Activity Monitoring Monitoring colony activity is crucial for detecting signs of stress, food insufficiency, or disease. AI-driven computer vision systems analyze real-time footage from hive entrance cameras, counting bees and identifying fluctuations in activity. These systems can also track bees carrying pollen, offering insights into pollination success. 4. Automated Hive Management Computer vision technology assists in counting bees, analyzing frame images, and automating beekeeping techniques. Smart farming solutions powered by AI-driven monitoring systems enable beekeepers to make data-driven decisions that maintain hive health. 5. Remote Monitoring Real-time surveillance systems equipped with AI-powered cameras allow beekeepers to monitor hive activity remotely. These systems can detect changes in bee behavior and classify flower species, ensuring optimal hive placement and management. 6. Pollen Type Detection Analyzing the pollen collected by bees provides valuable insights into colony nutrition. AI-enabled vision models trained on microscopic pollen images can differentiate between pollen types based on their unique structures. This information helps beekeepers make informed decisions about hive placement, honey processing, and forage availability. How Computer Vision Enhances Hive Monitoring Computer vision processes visual data from cameras and sensors to analyze hive activity. The integration of AI into beekeeping follows these steps: 1. Data Gathering Collecting images and videos from hive entrances, floral environments, and bee activity is the foundation of AI-based monitoring. 2. Data Annotation Labeling images to identify pests, flowers, bees, and pollen types ensures accurate AI training. 3. Model Training AI models are trained using datasets to recognize normal and abnormal bee activity, disease symptoms, and preferred foraging sites. 4. Validation & Testing The accuracy of AI models is tested in differen
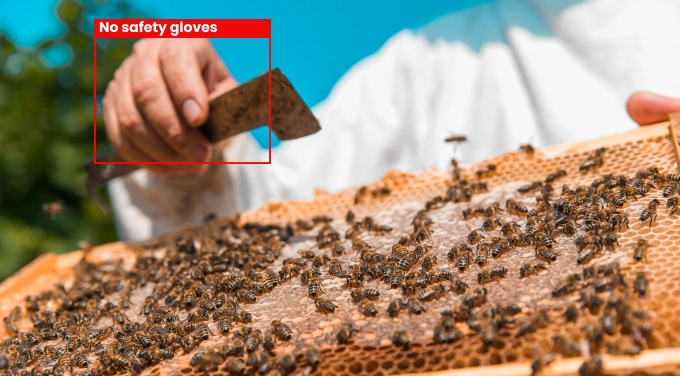
Beekeeping is an ancient practice that has long relied on honeybees for honey production and pollination. According to recent research by the Environment America Research & Policy Center, bees contribute to pollinating 73 of the 103 crops essential to global agriculture. Traditionally, beekeepers have monitored hive health and productivity through manual inspections, a process that is time-consuming and reliant on human observation. However, advancements in artificial intelligence (AI) and computer vision in beekeeping have paved the way for automated hive monitoring.
Modern AI-driven technology can analyze hive health, detect diseases, classify species and pollen in real-time, and provide beekeepers with crucial insights. By leveraging AI-based monitoring, beekeepers can enhance colony health and improve pollination efficiency in agricultural ecosystems. This article explores how integrating computer vision into beekeeping can transform colony productivity and shape the future of hive management.
Challenges in Modern Beekeeping
Beekeepers face numerous challenges in maintaining colony health and productivity. Traditional monitoring techniques are inefficient, making it difficult to sustain thriving hives.
1. Detection of Hive Diseases
Pests and bacterial infections can spread rapidly within a beehive, threatening the entire colony. Early detection of diseases such as the Varroa destructor infestation is crucial to maintaining a healthy hive.
2. Foraging Behavior Surveillance
Tracking bee activity and identifying the flowers they visit helps optimize pollination. However, monitoring this behavior across vast areas without automation is challenging.
3. Tracking Bee Populations
Accurate records of bees entering and exiting the hive provide insights into colony strength. Manual counting, however, is impractical.
4. Addressing Environmental Threats
Climate change, habitat loss, and extreme temperature fluctuations pose significant risks to bee colonies. Monitoring these environmental conditions in real-time is essential to colony survival.
To address these challenges, AI-powered real-time monitoring provides automated solutions that reduce the human workload while offering data-driven insights.
Practical Applications of Computer Vision in Beekeeping
AI-powered vision systems enable beekeepers to enhance hive monitoring and pollination tracking in various ways:
1. Early Disease Detection
The Varroa destructor is a common parasite that weakens bee colonies and contributes to colony collapse disorder. AI-powered object detection systems can identify mites early, preventing widespread infestations.
2. Flower Detection & Classification
By identifying high-value nectar sources, beekeepers can make informed decisions to support honey yield and colony health. AI models trained to classify flower species analyze images captured by drones, helping beekeepers adjust hive placement and maintain access to diverse floral resources.
3. Activity Monitoring
Monitoring colony activity is crucial for detecting signs of stress, food insufficiency, or disease. AI-driven computer vision systems analyze real-time footage from hive entrance cameras, counting bees and identifying fluctuations in activity. These systems can also track bees carrying pollen, offering insights into pollination success.
4. Automated Hive Management
Computer vision technology assists in counting bees, analyzing frame images, and automating beekeeping techniques. Smart farming solutions powered by AI-driven monitoring systems enable beekeepers to make data-driven decisions that maintain hive health.
5. Remote Monitoring
Real-time surveillance systems equipped with AI-powered cameras allow beekeepers to monitor hive activity remotely. These systems can detect changes in bee behavior and classify flower species, ensuring optimal hive placement and management.
6. Pollen Type Detection
Analyzing the pollen collected by bees provides valuable insights into colony nutrition. AI-enabled vision models trained on microscopic pollen images can differentiate between pollen types based on their unique structures. This information helps beekeepers make informed decisions about hive placement, honey processing, and forage availability.
How Computer Vision Enhances Hive Monitoring
Computer vision processes visual data from cameras and sensors to analyze hive activity. The integration of AI into beekeeping follows these steps:
1. Data Gathering
Collecting images and videos from hive entrances, floral environments, and bee activity is the foundation of AI-based monitoring.
2. Data Annotation
Labeling images to identify pests, flowers, bees, and pollen types ensures accurate AI training.
3. Model Training
AI models are trained using datasets to recognize normal and abnormal bee activity, disease symptoms, and preferred foraging sites.
4. Validation & Testing
The accuracy of AI models is tested in different hive environments and lighting conditions to ensure reliability.
5. Deploying Monitoring Systems
AI-powered computer vision systems are integrated into hive monitoring, providing real-time analytics for improved colony management.
By following these steps, beekeepers can customize computer vision models to detect and respond to hive conditions efficiently. AI-driven solutions help optimize management strategies and support sustainable beekeeping practices.
Conclusion
As beekeeping faces increasing challenges, AI-powered computer vision offers innovative solutions for disease detection, hive monitoring, and pollination optimization. From bee counting and disease identification to flower classification and pollen analysis, AI-driven technologies help maintain healthy colonies and improve honey production.
As a leading computer vision development company, Nextbrain provides AI video analytics software tailored for beekeeping and other industries. Our advanced AI models can distinguish between bees carrying pollen and those returning empty-handed, enabling beekeepers to make data-driven decisions.
Interested in learning more about AI-driven beekeeping solutions? Get in touch with our professionals today to explore the future of hive monitoring with AI video analytics.