Manus AI: The Revolutionary Multi-Agent System Reshaping the Future of AI Assistants
In recent months, AI agents have transitioned from experimental curiosities to increasingly practical tools, with platforms like DeepSeek and OpenAI's Deep Research leading the charge. Now, a new contender has emerged: Manus, a general-purpose AI agent capturing global attention with its innovative approach. This article delves into Manus AI, exploring its unique architecture, impressive capabilities, and the ongoing debate surrounding its classification as an AI "wrapper." Discover how Manus is challenging conventional AI development and potentially redefining the future of AI assistants. This article is for technology enthusiasts, AI developers, startup founders, and anyone curious about the latest advancements in artificial intelligence. We aim to provide a comprehensive overview of Manus AI, its impact, and its place in the rapidly evolving AI landscape. Understanding Manus: Architecture and Innovation Manus distinguishes itself through its multi-agent system, a departure from the monolithic neural networks often employed in AI development. Instead of relying on a single, massive model, Manus operates more like an executive overseeing a team of specialized sub-agents. This architecture allows for a more nuanced and efficient approach to complex tasks. Multi-Agent System Overview Executive Oversight Model: Manus takes in your prompt and acts as a coordinator, figuring out what needs to be done. Coordination Across Shared Action Space: The system breaks down complex tasks into manageable subtasks, creating a master plan for execution. 29 Integrated Tools Ecosystem: Manus can call upon an extensive suite of 29 different integrated tools. Role of Sub-Agents and Specialization: These sub-agents are like in-house experts, each with a delineated domain, from knowledge and memory to execution. This multi-agent approach allows Manus to leverage specialized expertise for different aspects of a task, leading to more accurate and efficient results. Technical Infrastructure Manus's architecture is underpinned by several key technical components: Dynamic Task Decomposition Algorithm: This algorithm enables Manus to autonomously break down complex instructions into clear execution paths. Chain of Thought Injection Technique: To ensure stability, the Manus team developed an original technique called chain of thought injection, enabling agents to actively reflect and update plans. Integration with Claude 3.7 Sonnet: At its core, Manus makes use of Anthropic's Claude 3.7 Sonnet. Cross-Platform Execution Capabilities: Manus features robust cross-platform execution capabilities thanks to its seamless integration with open-source tools. Partnership with YC Company Browser: For advanced website interaction, Manus partners with YC company browser use. These technical elements combine to create a robust and adaptable AI system capable of handling a wide range of tasks. Performance and Capabilities Manus's capabilities extend beyond theoretical innovation, demonstrating impressive performance in real-world applications and benchmark testing. Benchmark Performance Gaia Benchmark Results (86.5% Score): Manus smashed the state-of-the-art on Gaia, scoring 86.5%. Comparison to Human Performance (92%): Just a few points shy of the average human. Contrast with OpenAI's Deep Research (74%): Humans typically score about 92%, whereas OpenAI's Deep Research, in comparison, scored about 74% at its best. These results highlight Manus's ability to reason, handle multimodal data, browse the web, and utilize tools effectively. Real-World Applications Manus excels in a variety of real-world scenarios: Travel Planning Capabilities: Creating detailed travel itineraries. Financial Analysis Features: Performing detailed financial analyses. Educational Content Creation: Assisting with educational content. Database Compilation: Assisting with structured database compilation. Insurance Policy Comparisons: Comparing insurance policies. Supplier Sourcing: Sourcing suppliers. Presentation Assistance: Assisting with high-quality presentations. These diverse applications demonstrate Manus's versatility and potential to streamline various tasks across different industries. The "Wrapper" Debate The emergence of Manus has reignited a debate about the nature of AI startups at the application layer, often referred to as "wrappers." Understanding the Criticism Definition of AI "Wrappers": Some have dismissed Manus as merely a wrapper since it stitches together existing foundational models and various tool calls. Common Misconceptions: But this dismissal overlooks an important reality. Industry Examples: Cursor and Windsurf, for example, integrate existing LLMs alongside external APIs and developer-focused tooling such as real-time code analysis and debugging utilities. The criticism often stems from the perception tha
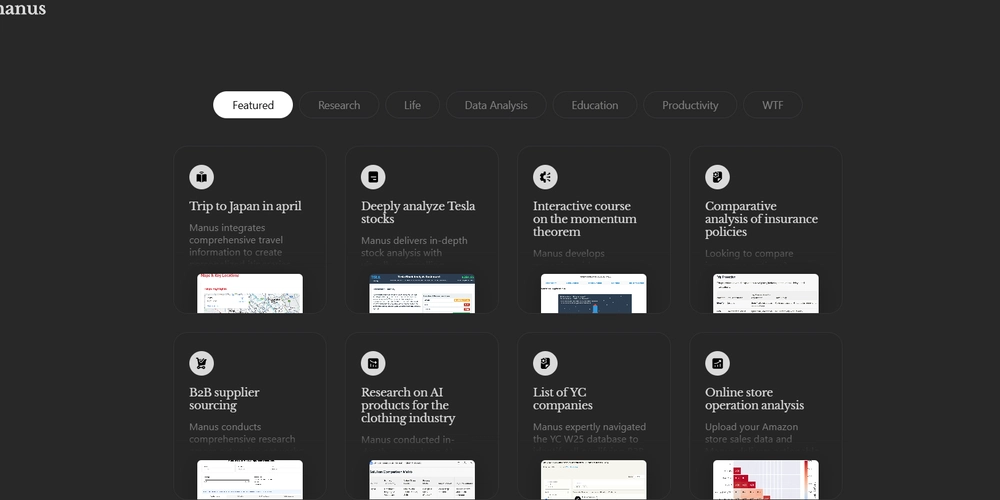
In recent months, AI agents have transitioned from experimental curiosities to increasingly practical tools, with platforms like DeepSeek and OpenAI's Deep Research leading the charge. Now, a new contender has emerged: Manus, a general-purpose AI agent capturing global attention with its innovative approach.
This article delves into Manus AI, exploring its unique architecture, impressive capabilities, and the ongoing debate surrounding its classification as an AI "wrapper." Discover how Manus is challenging conventional AI development and potentially redefining the future of AI assistants.
This article is for technology enthusiasts, AI developers, startup founders, and anyone curious about the latest advancements in artificial intelligence. We aim to provide a comprehensive overview of Manus AI, its impact, and its place in the rapidly evolving AI landscape.
Understanding Manus: Architecture and Innovation
Manus distinguishes itself through its multi-agent system, a departure from the monolithic neural networks often employed in AI development. Instead of relying on a single, massive model, Manus operates more like an executive overseeing a team of specialized sub-agents. This architecture allows for a more nuanced and efficient approach to complex tasks.
Multi-Agent System Overview
- Executive Oversight Model: Manus takes in your prompt and acts as a coordinator, figuring out what needs to be done.
- Coordination Across Shared Action Space: The system breaks down complex tasks into manageable subtasks, creating a master plan for execution.
- 29 Integrated Tools Ecosystem: Manus can call upon an extensive suite of 29 different integrated tools.
- Role of Sub-Agents and Specialization: These sub-agents are like in-house experts, each with a delineated domain, from knowledge and memory to execution.
This multi-agent approach allows Manus to leverage specialized expertise for different aspects of a task, leading to more accurate and efficient results.
Technical Infrastructure
Manus's architecture is underpinned by several key technical components:
- Dynamic Task Decomposition Algorithm: This algorithm enables Manus to autonomously break down complex instructions into clear execution paths.
- Chain of Thought Injection Technique: To ensure stability, the Manus team developed an original technique called chain of thought injection, enabling agents to actively reflect and update plans.
- Integration with Claude 3.7 Sonnet: At its core, Manus makes use of Anthropic's Claude 3.7 Sonnet.
- Cross-Platform Execution Capabilities: Manus features robust cross-platform execution capabilities thanks to its seamless integration with open-source tools.
- Partnership with YC Company Browser: For advanced website interaction, Manus partners with YC company browser use.
These technical elements combine to create a robust and adaptable AI system capable of handling a wide range of tasks.
Performance and Capabilities
Manus's capabilities extend beyond theoretical innovation, demonstrating impressive performance in real-world applications and benchmark testing.
Benchmark Performance
- Gaia Benchmark Results (86.5% Score): Manus smashed the state-of-the-art on Gaia, scoring 86.5%.
- Comparison to Human Performance (92%): Just a few points shy of the average human.
- Contrast with OpenAI's Deep Research (74%): Humans typically score about 92%, whereas OpenAI's Deep Research, in comparison, scored about 74% at its best.
These results highlight Manus's ability to reason, handle multimodal data, browse the web, and utilize tools effectively.
Real-World Applications
Manus excels in a variety of real-world scenarios:
- Travel Planning Capabilities: Creating detailed travel itineraries.
- Financial Analysis Features: Performing detailed financial analyses.
- Educational Content Creation: Assisting with educational content.
- Database Compilation: Assisting with structured database compilation.
- Insurance Policy Comparisons: Comparing insurance policies.
- Supplier Sourcing: Sourcing suppliers.
- Presentation Assistance: Assisting with high-quality presentations.
These diverse applications demonstrate Manus's versatility and potential to streamline various tasks across different industries.
The "Wrapper" Debate
The emergence of Manus has reignited a debate about the nature of AI startups at the application layer, often referred to as "wrappers."
Understanding the Criticism
- Definition of AI "Wrappers": Some have dismissed Manus as merely a wrapper since it stitches together existing foundational models and various tool calls.
- Common Misconceptions: But this dismissal overlooks an important reality.
- Industry Examples: Cursor and Windsurf, for example, integrate existing LLMs alongside external APIs and developer-focused tooling such as real-time code analysis and debugging utilities.
The criticism often stems from the perception that these applications are simply repackaging existing AI models without adding significant value.
Value Proposition
However, this perspective overlooks the unique value proposition of well-designed AI wrappers:
- Cost Efficiency: Manus's multi-agent orchestration helps deliver significantly lower per-task costs, around $2 a task compared to integrated competitors like OpenAI's Deep Research.
- Transparency and User Control: Manus also offers greater transparency and user control, letting users directly inspect, customize, or replace individual sub-agents and tool integrations.
- Customization Capabilities: A degree of flexibility centralized platforms rarely match.
- File System Visibility: One of the coolest things Manus figured out was exposing the file system so you could see exactly what the agents were doing.
These advantages highlight the potential for AI wrappers to provide cost-effective, transparent, and customizable AI solutions.
Strengths and Limitations
Like any technology, Manus has its strengths and limitations.
Advantages
- Lower Operational Costs: Manus's multi-agent architecture contributes to lower per-task costs.
- Enhanced User Control: Users have the ability to inspect, customize, or replace individual sub-agents and tool integrations.
- Transparent Operations: The file system visibility provides insight into the agent's actions.
- Flexible Architecture: The modular design allows for adaptability and customization.
Challenges
- Coordination Complexity at Scale: Coordination across specialized agents becomes increasingly difficult as tasks scale or complexity grows.
- Vulnerability to Competition: Its current advantages—UX refinements, targeted fine-tuning, thoughtful integrations—are vulnerable to competitors just coming along and doing that as well.
- API Pricing Dependencies: API pricing changes or provider policy shifts can quickly erase any of the cost benefits.
Addressing these limitations will be crucial for Manus's long-term success.
Future Implications and Industry Impact
Manus's emergence has significant implications for developers, startups, and the broader AI industry.
For Developers and Startups
- Strategic Differentiation Opportunities: For founders, this might mean investing early in proprietary evaluations that are expensive or time-consuming to replicate.
- Investment Priorities: Embedding your workflows deeply into specific user routines to increase switching costs.
- Integration Strategies: Identifying integrations with platforms or datasets competitors can't easily access.
- Market Positioning: In the end, success in AI doesn't hinge on reinventing the wheel but rather on who can stitch together existing models into a product users genuinely love.
Future Development Paths
- Potential Improvements: Continued refinement of the multi-agent architecture.
- Industry Evolution: The rise of specialized AI agents and the evolution of AI wrappers.
- Competitive Landscape Changes: The emergence of new players and the consolidation of existing ones.
Conclusion
Manus AI represents a significant step forward in the evolution of AI assistants. Its innovative multi-agent architecture, impressive benchmark performance, and potential for cost-effective solutions position it as a key player in the AI landscape. While the debate surrounding AI wrappers continues, Manus demonstrates the value of thoughtfully designed applications that leverage existing AI models to solve real-world problems.
For developers and users alike, Manus offers a glimpse into the future of AI, where specialized agents work together to streamline tasks and enhance productivity. As the AI industry continues to evolve, Manus's approach may well become a blueprint for the next generation of AI assistants.