How Tech Giants Leverage AI Acquisitions: Building a Strong Foundation in 2025
Below, we’ll look into why these AI acquisitions matter, how they reflect a broader shift in the tech landscape, and what you can do to future-proof your own venture (without waiting for a giant to show up at your door). 1. Why Big Companies Buy AI Startups Tech giants have historically expanded through acquisitions, but 2025 has taken it up a notch. From enterprise software providers integrating AI-driven workflow automation, to social media conglomerates using advanced NLP or computer-vision startups, the message is the same: AI isn’t a buzzword—it’s a main differentiator. Some real-world examples reinforce this point: ServiceNow acquiring Moveworks for automating tasks within enterprises. CoreWeave buying Weights & Biases to enhance tracking and monitoring insights. UI Path buys Peak.ai to help with business process efficiency. Even if a corporation built its brand on something else—like consumer apps, business processes, or data analytics—it sees AI as the missing puzzle piece to stay competitive. Often, these larger companies need specialized engineering and research teams fast, and a well-chosen acquisition is the quickest path there. 2. What This Means for Smaller and Mid-Sized Startups If you’re a small or medium enterprise trying to “catch up” on AI, these acquisitions might feel both exciting and intimidating. On one hand, it signals an industry shift that underscores the value of specialized AI and data capabilities. On the other hand, not everyone has the resources to buy an entire startup when they need advanced AI. What you can do instead is invest systematically in AI fundamentals: Data Architecture: Make sure your data flows, pipelines, and storage are well-organized—something we emphasize heavily at AI Flow. Trying to layer advanced algorithms on messy data is a recipe for minimal impact and high frustration. Skilled Team: Even if you use existing off-the-shelf models, you need a team or consultant who understands how to adapt and scale them. You don’t need an army of PhDs, but you do need people who truly get how ML systems work under the hood. Responsible Experimentation: Aim for well-scoped AI pilots that solve concrete issues, like automating part of your customer support or refining your product recommendation engine. From there, scale incrementally. 3. Building a Future-Proof Strategy Major acquisitions happen because AI is much more than a marketing line: it optimizes processes, uncovers new revenue, and solves real pain points. Startups that figure out how to integrate AI responsibly from day one often get noticed (and in some cases acquired). But even if acquisition isn’t your end goal, applying these principles can help you stand out: Clarity on Use Cases: Don’t jump into AI just because it’s trendy. Identify a specific business process or user experience that genuinely benefits from advanced algorithms. For instance, one of our clients had a high data volume platform that needed better data ingestion methods. We built data pipelines, with information extraction and summarization ML components. Nothing flashy, just targeted components, used at scale, to ingest millions of data records daily, from unstructured documents. Modularity: Big companies lean on AI “building blocks”—like natural language models, image classifiers, or specialized recommendation engines. You can do the same by choosing tools (or an internal framework) that let you swap out components as technology evolves. That way, if the latest open-source model is better than your current one, it’s easier to integrate without rewriting your entire application. Data Ownership: We see it consistently—whoever controls the best data and knows how to harness it gains a competitive edge. Make sure you track the data you’re collecting, how it’s cleaned, and who has access. This is crucial not just for building robust AI but for compliance, especially under new or upcoming regulations. 4. Lessons from Past Client Projects At AI Flow, we’ve worked with companies that realized they needed advanced AI capabilities only after hitting scaling issues: Financing platform We rebuilt an outdated student-financing platform in a few months, launching an MVP that seamlessly scaled to thousands of users from day one. Once we had enough data on usage, we used AI to cluster contracts and generate insights from them. ExoMatter By introducing an AutoML pipeline (via Google’s Vertex AI) for predicting material properties, we cut model-development time from months to hours—transforming the way this materials-science startup manages data and experimentation. In both instances, the push for AI came from real business strain. If you approach AI from a place of immediate operational need, you’re more likely to invest in methods or technologies that stick—and that’s exactly how acquisitions get justified at the enterprise level. 5. Looking Ahead: The
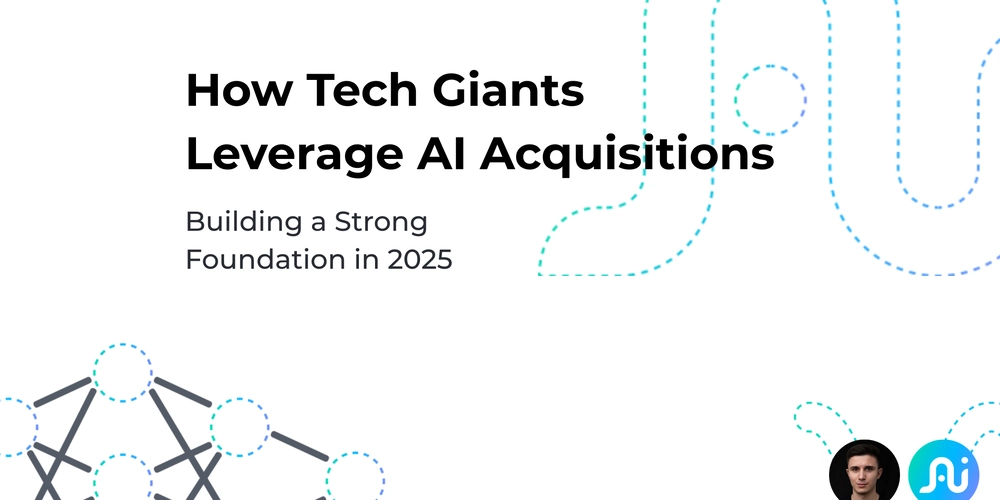
Below, we’ll look into why these AI acquisitions matter, how they reflect a broader shift in the tech landscape, and what you can do to future-proof your own venture (without waiting for a giant to show up at your door).
1. Why Big Companies Buy AI Startups
Tech giants have historically expanded through acquisitions, but 2025 has taken it up a notch. From enterprise software providers integrating AI-driven workflow automation, to social media conglomerates using advanced NLP or computer-vision startups, the message is the same: AI isn’t a buzzword—it’s a main differentiator.
Some real-world examples reinforce this point:
- ServiceNow acquiring Moveworks for automating tasks within enterprises.
- CoreWeave buying Weights & Biases to enhance tracking and monitoring insights.
- UI Path buys Peak.ai to help with business process efficiency.
Even if a corporation built its brand on something else—like consumer apps, business processes, or data analytics—it sees AI as the missing puzzle piece to stay competitive. Often, these larger companies need specialized engineering and research teams fast, and a well-chosen acquisition is the quickest path there.
2. What This Means for Smaller and Mid-Sized Startups
If you’re a small or medium enterprise trying to “catch up” on AI, these acquisitions might feel both exciting and intimidating. On one hand, it signals an industry shift that underscores the value of specialized AI and data capabilities. On the other hand, not everyone has the resources to buy an entire startup when they need advanced AI.
What you can do instead is invest systematically in AI fundamentals:
- Data Architecture: Make sure your data flows, pipelines, and storage are well-organized—something we emphasize heavily at AI Flow. Trying to layer advanced algorithms on messy data is a recipe for minimal impact and high frustration.
- Skilled Team: Even if you use existing off-the-shelf models, you need a team or consultant who understands how to adapt and scale them. You don’t need an army of PhDs, but you do need people who truly get how ML systems work under the hood.
- Responsible Experimentation: Aim for well-scoped AI pilots that solve concrete issues, like automating part of your customer support or refining your product recommendation engine. From there, scale incrementally.
3. Building a Future-Proof Strategy
Major acquisitions happen because AI is much more than a marketing line: it optimizes processes, uncovers new revenue, and solves real pain points. Startups that figure out how to integrate AI responsibly from day one often get noticed (and in some cases acquired). But even if acquisition isn’t your end goal, applying these principles can help you stand out:
- Clarity on Use Cases: Don’t jump into AI just because it’s trendy. Identify a specific business process or user experience that genuinely benefits from advanced algorithms. For instance, one of our clients had a high data volume platform that needed better data ingestion methods. We built data pipelines, with information extraction and summarization ML components. Nothing flashy, just targeted components, used at scale, to ingest millions of data records daily, from unstructured documents.
- Modularity: Big companies lean on AI “building blocks”—like natural language models, image classifiers, or specialized recommendation engines. You can do the same by choosing tools (or an internal framework) that let you swap out components as technology evolves. That way, if the latest open-source model is better than your current one, it’s easier to integrate without rewriting your entire application.
- Data Ownership: We see it consistently—whoever controls the best data and knows how to harness it gains a competitive edge. Make sure you track the data you’re collecting, how it’s cleaned, and who has access. This is crucial not just for building robust AI but for compliance, especially under new or upcoming regulations.
4. Lessons from Past Client Projects
At AI Flow, we’ve worked with companies that realized they needed advanced AI capabilities only after hitting scaling issues:
-
Financing platform
We rebuilt an outdated student-financing platform in a few months, launching an MVP that seamlessly scaled to thousands of users from day one. Once we had enough data on usage, we used AI to cluster contracts and generate insights from them.
-
ExoMatter
By introducing an AutoML pipeline (via Google’s Vertex AI) for predicting material properties, we cut model-development time from months to hours—transforming the way this materials-science startup manages data and experimentation.
In both instances, the push for AI came from real business strain. If you approach AI from a place of immediate operational need, you’re more likely to invest in methods or technologies that stick—and that’s exactly how acquisitions get justified at the enterprise level.
5. Looking Ahead: The 2025 AI Landscape
With major acquisitions ramping up, the AI market feels busier than ever. A few trends are especially relevant:
- Industry-Specific AI: Bigger companies often acquire startups with domain expertise—like healthcare or shipping—because generalized solutions only go so far. For smaller players, targeting a niche where you can show real results might be your best bet.
- Regulatory Environment: As more data gets absorbed into large-scale AI, regulations will tighten. Being transparent, ethical, and well-documented with your data processes can help you stand out—or avoid major headaches later.
- Ecosystem Partnerships: Not every business can do end-to-end AI alone. Partnerships with specialized agencies or consultants often accelerate the path to meaningful ROI, without requiring the overhead of in-house hires for every skill set.
Ultimately, if you want to remain competitive, you have two paths: build strong AI capabilities internally or rely on an external partner that knows how to integrate cutting-edge algorithms with solid engineering practices. The wave of acquisitions underscores just how important it is to pick one of these paths sooner rather than later.
Final Thoughts
Just because you’re not a multibillion-dollar conglomerate doesn’t mean you can’t leverage AI effectively—whether your goal is to get acquired down the line or simply scale to new markets. By focusing on robust data practices, specialized talent, and carefully chosen pilot projects, you position your company to thrive in an environment where AI is increasingly the engine of innovation.
And as the big names continue to acquire specialized AI startups, remember that these deals aren’t just about technology; they’re about strategy. Your advantage as a smaller or mid-sized firm is agility—if you craft a compelling AI-enabled solution, you’re not only future-proofing your operations, but placing yourself in a strategic spot that bigger players can’t ignore.