How Glean Leverages Hybrid Search for Accurate and Efficient Enterprise AI
Glean is a work AI platform that seamlessly connects and understands all your company's data, enabling employees to find answers, generate content, and automate tasks with AI. Glean empowers organisations to maximise the full potential of their data securely and efficiently by integrating with various enterprise applications and ensuring robust data and AI governance. Its mission is to expand the human potential to do extraordinary work. This article explores how Glean uses a hybrid search approach to overcome the limitations of traditional enterprise search methods. It highlights why hybrid search search is important in enterprise AI and the core components of Glean’s hybrid search architecture. The article also covers the implementation process, while showcasing the performance benchmarks and real-world applications. Balancing Accuracy and Efficiency in Enterprise Search Employees need quick and accurate access to information. However, finding the right balance between accuracy and efficiency in enterprise search can be challenging. When search tools are too focused on accuracy, they may take longer to deliver results, causing frustration and lost productivity. On the other hand, if the focus is solely on speed, the results may lack relevance, leading to wasted time sifting through unnecessary information. To strike the right balance, organizations should invest in search solutions that combine intelligent algorithms with user-friendly features. This means incorporating natural language processing, machine learning, and contextual understanding to deliver accurate results without compromising speed. Why Hybrid Search is Important in Enterprise AI Traditional enterprise search systems face challenges due to the growing volume and diversity of data and the limitations inherent in both keyword-based and purely neural search methods. Here are some of the key challenges faced by these systems - Volume and Diversity of Enterprise Data Modern enterprises produce massive amounts of data, often stored across various systems, formats, and locations. Traditional search engines struggle to keep up with the sheer volume and diversity, leading to inefficient and incomplete search results. This challenge is amplified when data is unstructured, like emails, PDFs, or multimedia files, making it harder for conventional systems to index and search accurately. Limitations of Keyword-Based Search Keyword-based search methods rely on matching exact words or phrases. While this approach works for simple queries, it becomes less effective when the user does not know the exact terms used in the data. As a result, searches can return irrelevant or incomplete results. Additionally, keyword-based systems often fail to understand context or semantic relationships, leading to a lack of nuanced results. Shortcomings of Purely Neural Search Purely neural search methods, which leverage machine learning models, are designed to overcome some keyword-based limitations. However, they come with their own set of challenges. These models require substantial training data and computational resources. Furthermore, they may produce results that are not easily interpretable or verifiable, which can be problematic for enterprises requiring transparency and accuracy. Why Hybrid Search? Finding the right information quickly and accurately is crucial. With the ever-growing amount of data, traditional search methods sometimes fall short. This is where hybrid search comes into play. Hybrid search combines the strengths of two main search approaches, keyword-based search and semantic search. This means that even if users phrase their queries differently or use synonyms, the system can still deliver accurate results. It not only looks for keyword matches but also understands the context and meaning behind the words. By leveraging both methods, hybrid search provides more relevant and accurate results, regardless of how users phrase their queries. Core Components of Glean's Hybrid Search Glean's hybrid search system is designed to enhance how organizations retrieve and utilize information by combining several advanced technologies. The core components of this system include - Enterprise Knowledge Graph At the heart of Glean's search capabilities is the enterprise knowledge graph. This framework models an organization's data by understanding the relationships between content, people, and activities. By mapping these connections, Glean provides search results that are contextually relevant and tailored to the user's needs. This approach moves beyond simple keyword matching to comprehend the nuances of organizational information. Integration of Vector and Lexical Search Glean uses a hybrid approach by integrating both vector (semantic) search and lexical (keyword-based) search methods. Vector search interprets the meaning behind queries, enabling the system to find relevant information even when exact
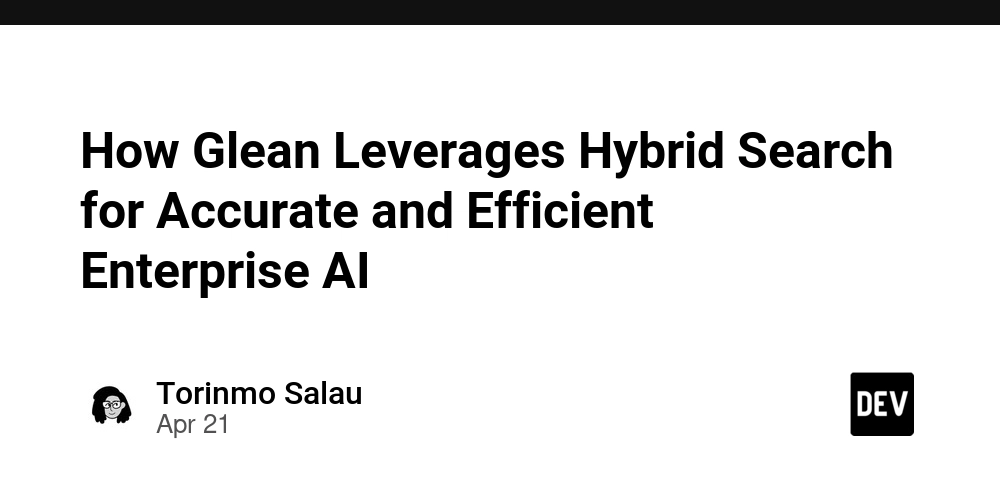
Glean is a work AI platform that seamlessly connects and understands all your company's data, enabling employees to find answers, generate content, and automate tasks with AI. Glean empowers organisations to maximise the full potential of their data securely and efficiently by integrating with various enterprise applications and ensuring robust data and AI governance. Its mission is to expand the human potential to do extraordinary work.
This article explores how Glean uses a hybrid search approach to overcome the limitations of traditional enterprise search methods. It highlights why hybrid search search is important in enterprise AI and the core components of Glean’s hybrid search architecture. The article also covers the implementation process, while showcasing the performance benchmarks and real-world applications.
Balancing Accuracy and Efficiency in Enterprise Search
Employees need quick and accurate access to information. However, finding the right balance between accuracy and efficiency in enterprise search can be challenging. When search tools are too focused on accuracy, they may take longer to deliver results, causing frustration and lost productivity.
On the other hand, if the focus is solely on speed, the results may lack relevance, leading to wasted time sifting through unnecessary information. To strike the right balance, organizations should invest in search solutions that combine intelligent algorithms with user-friendly features. This means incorporating natural language processing, machine learning, and contextual understanding to deliver accurate results without compromising speed.
Why Hybrid Search is Important in Enterprise AI
Traditional enterprise search systems face challenges due to the growing volume and diversity of data and the limitations inherent in both keyword-based and purely neural search methods. Here are some of the key challenges faced by these systems -
- Volume and Diversity of Enterprise Data Modern enterprises produce massive amounts of data, often stored across various systems, formats, and locations. Traditional search engines struggle to keep up with the sheer volume and diversity, leading to inefficient and incomplete search results. This challenge is amplified when data is unstructured, like emails, PDFs, or multimedia files, making it harder for conventional systems to index and search accurately.
- Limitations of Keyword-Based Search Keyword-based search methods rely on matching exact words or phrases. While this approach works for simple queries, it becomes less effective when the user does not know the exact terms used in the data. As a result, searches can return irrelevant or incomplete results. Additionally, keyword-based systems often fail to understand context or semantic relationships, leading to a lack of nuanced results.
- Shortcomings of Purely Neural Search Purely neural search methods, which leverage machine learning models, are designed to overcome some keyword-based limitations. However, they come with their own set of challenges. These models require substantial training data and computational resources. Furthermore, they may produce results that are not easily interpretable or verifiable, which can be problematic for enterprises requiring transparency and accuracy.
Why Hybrid Search?
Finding the right information quickly and accurately is crucial. With the ever-growing amount of data, traditional search methods sometimes fall short. This is where hybrid search comes into play.
Hybrid search combines the strengths of two main search approaches, keyword-based search and semantic search. This means that even if users phrase their queries differently or use synonyms, the system can still deliver accurate results. It not only looks for keyword matches but also understands the context and meaning behind the words. By leveraging both methods, hybrid search provides more relevant and accurate results, regardless of how users phrase their queries.
Core Components of Glean's Hybrid Search
Glean's hybrid search system is designed to enhance how organizations retrieve and utilize information by combining several advanced technologies. The core components of this system include -
- Enterprise Knowledge Graph At the heart of Glean's search capabilities is the enterprise knowledge graph. This framework models an organization's data by understanding the relationships between content, people, and activities. By mapping these connections, Glean provides search results that are contextually relevant and tailored to the user's needs. This approach moves beyond simple keyword matching to comprehend the nuances of organizational information.
- Integration of Vector and Lexical Search Glean uses a hybrid approach by integrating both vector (semantic) search and lexical (keyword-based) search methods. Vector search interprets the meaning behind queries, enabling the system to find relevant information even when exact keywords aren't present. Lexical search, on the other hand, ensures precision by matching specific terms. Combining these methods allows Glean to deliver comprehensive and accurate search results, catering to various user intents and query types.
- Advanced Crawling and Data Integration To ensure a thorough search experience, Glean features a robust and scalable crawling mechanism. This component connects to all enterprise data sources, adhering to existing permission structures and governance rules. By integrating seamlessly with various applications and data repositories, Glean ensures that users have access to the most current and relevant information available within their organization.
- AI-Powered Personalization and Learning Glean's system continuously learns from user interactions to refine and personalise search results. Using machine learning algorithms, it adapts to individual user behaviours, preferences, and roles within the organisation. This self-tuning capability ensures that over time, the search experience becomes more aligned with each user's specific needs, enhancing productivity and satisfaction.
Implementation Techniques of Glean's Hybrid Search
Glean's hybrid search combines traditional keyword-based methods with advanced AI techniques to enhance search accuracy and relevance. Here's a breakdown of how it works:
Traditional Keyword Search (Sparse Vectors)
Initially, Glean uses a method similar to traditional keyword searches, like the BM25 algorithm. This approach matches the exact words in your query to documents containing those words, ranking them based on factors like word frequency and document length. While effective for direct matches, it may miss documents that are relevant but don't contain the exact search terms.
AI-Powered Semantic Search (Dense Vectors)
To address this, Glean employs AI models that understand the meaning behind words. These models convert text into dense vector representations, capturing the semantic essence of the content. This allows Glean to identify documents that are contextually related to your query, even if they don't share the exact wording.
Combining Both Methods (Hybrid Search)
Glean integrates both approaches to leverage their strengths. It retrieves initial results using the keyword method and then re-ranks them based on semantic relevance. This combination ensures that search results are both precise and contextually pertinent. To merge the results from both methods, Glean uses techniques like Reciprocal Rank Fusion (RRF). RRF assigns scores to documents from each method and combines them, balancing the influence of exact keyword matches and semantic relevance. This ensures a comprehensive and accurate ranking of search results.
Performance Evaluation of Glean's Hybrid Search
To assess the performance of Glean's hybrid search, we use the following metrics:
Precision and Recall: These metrics measure the accuracy of search results. Precision calculates the proportion of relevant results out of all retrieved documents, while recall measures the proportion of relevant documents successfully retrieved. Glean's search consistently shows higher precision, reducing irrelevant results by up to 30% compared to traditional methods.
Response Time: This efficiency metric evaluates the speed at which results are returned. Response times are comparable, with Glean optimizing for quick retrieval through pre-indexing techniques.
User Satisfaction: This is collected through feedback and usability studies. This metric gauges how well the search results meet user expectations. User satisfaction scores indicate that Glean's results are perceived as more accurate and relevant, particularly for complex queries.
Query Success Rate: Measures the percentage of queries that yield the expected results, indicating the reliability of the search system.
Improved Search Outcomes in an Enterprise Environment
A global enterprise integrated Glean's hybrid search into its internal knowledge management system. Before implementation, employees reported difficulties in finding documents due to inconsistent naming conventions and outdated indexing.
After deploying Glean, the following improvements were observed:
Precision increased by 25% and recall improved by 18%, retrieving documents previously overlooked by the old system.
Average response time decreased by 20%, despite the increased data volume.
Employee satisfaction rose by 40%, attributed to the system’s ability to interpret natural language queries more accurately.
Another example is Databricks, a fast-growing company with employees generating vast amounts of content across multiple tools, including Google Workspace, Atlassian, Salesforce, and Slack. Glean gave Databricks a unified search experience that delivers the right information instantly. Now employees can spend less time searching and more time working productively.
Final Thoughts
Glean is transforming the way enterprises access and use their pools of data. At the heart of Glean’s approach is hybrid search, a technique that combines the best aspects of traditional keyword-based search and modern AI-driven semantic search. This fusion results in more accurate and efficient ways to find information within enterprise systems.
Glean’s system intelligently switches between keyword and semantic searches based on the query type and the data being searched. For straightforward, term-specific searches, it prioritizes keyword matching. For more complex, nuanced questions, it leans on semantic algorithms. This dynamic approach ensures that employees quickly find the most relevant data, no matter how they phrase their queries.
Glean reduces the time spent searching for information. Employees spend less time digging through documents and more time being productive. This boost in efficiency directly translates to better decision-making and streamlined workflows.