Guide to Real-Time Data Stream APIs
Real-time data streams deliver the sub-second responsiveness that modern applications demand. While batch processing handles data in chunks, real-time processing transforms information instantly, creating experiences that feel truly alive. This isn't just technical preference—it's business critical. From financial fraud detection to manufacturing optimization, social media feeds to multiplayer gaming, real-time data streams have revolutionized how businesses operate. These systems require specialized API approaches to overcome unique challenges: crushing latency demands, managing massive throughput, and seamless scaling under fluctuating loads. Let's take a look at how to build and document APIs that make real-time data streams both powerful and accessible for developers who need immediate responsiveness in their applications. Mastering the Fundamentals: Core Concepts That Power Real-Time APIs Crafting Developer-Friendly Experiences: Designing Your Real-Time API Building Reliable Foundations: Setting Up the Server Side Delivering Data to Clients: Client-Side Implementation Bringing It All Together Mastering the Fundamentals: Core Concepts That Power Real-Time APIs Before diving into implementation details, understanding the foundational concepts behind real-time data streaming will ensure your API architecture stands on solid ground. When designing an API for real-time data streams, you're creating a high-performance data highway that handles incredible speeds without compromising reliability. The architecture patterns, data formats, and connection protocols you choose form the backbone of your entire system. Core Streaming Architecture Patterns These battle-tested approaches define how data flows through your system: Publish-Subscribe (Pub/Sub): Publishers send events to topics without caring who's listening, while subscribers only receive the data they've requested. This pattern excels for dashboards requiring fresh data or notification systems that must capture every event. Event Sourcing: Rather than just recording current state, event sourcing saves every change as an immutable sequence. This creates a complete historical record perfect for audit trails and time-travel debugging capabilities. Command Query Responsibility Segregation (CQRS): By splitting read and write paths, CQRS optimizes each for its specific purpose. Write operations focus on consistency while read operations prioritize speed—crucial for real-time data delivery. Data Formats Optimized for Streaming Your format choice significantly impacts performance: Avro: This binary format includes schema definitions with the data, handling evolving schemas elegantly. Avro pairs exceptionally well with Kafka for efficient, compact streaming. Protocol Buffers (Protobuf): Google's binary format delivers unmatched speed and minimal size. When latency is your primary concern, Protobuf offers the smallest payload and fastest serialization available. JSON: While less efficient than binary formats, JSON's human-readability and universal support make it valuable for debugging and web client integration. Just be prepared for the performance trade-off. Connection Protocols for Real-Time Data Streams These protocols determine how clients stay connected to your data stream: WebSockets: Creating persistent, full-duplex channels over a single TCP connection, WebSockets excel for applications requiring two-way communication like chat or collaborative tools. Server-Sent Events (SSE): Perfect for one-way server-to-client updates, SSE offers simplicity and broad browser support for news feeds, stock tickers, and similar applications. WebRTC: Enabling direct client-to-client communication, WebRTC eliminates the server middleman for peer-to-peer data streaming applications. Utilizing a hosted API gateway can simplify the management of these protocols, providing benefits such as scalability, security, and ease of deployment. Stateful vs. Stateless Processing This fundamental choice affects how your system handles data context: Stateless Processing: Processing each data piece in isolation allows horizontal scaling and simple failure recovery but limits analytical capabilities. Stateful Processing: Maintaining context across multiple events enables windowed aggregations, cross-stream joins, and pattern detection, though it adds complexity to scaling and recovery. Event Time vs. Processing Time Time concepts create critical distinctions in real-time systems: Event Time: When events actually occurred at the source—essential for accurate analytics but challenging with out-of-order arrivals and delayed data. Processing Time: When your system processes the event—simpler to implement but potentially misleading when events arrive with varying delays. With these foundational concepts clarified, you're equipped to make architecture choices that balance performance, reliabili
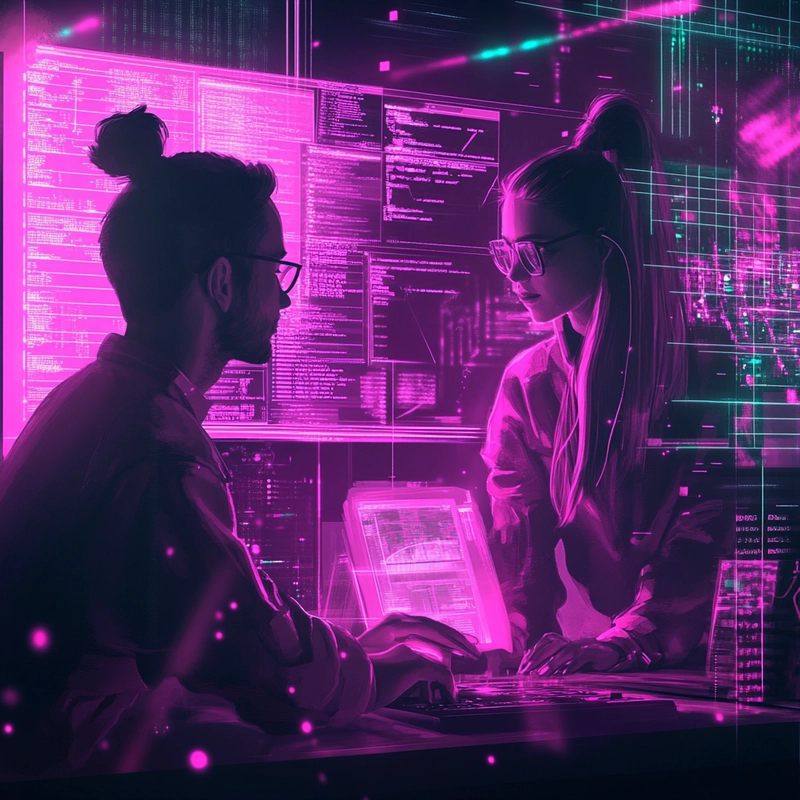
Real-time data streams deliver the sub-second responsiveness that modern applications demand. While batch processing handles data in chunks, real-time processing transforms information instantly, creating experiences that feel truly alive. This isn't just technical preference—it's business critical.
From financial fraud detection to manufacturing optimization, social media feeds to multiplayer gaming, real-time data streams have revolutionized how businesses operate. These systems require specialized API approaches to overcome unique challenges: crushing latency demands, managing massive throughput, and seamless scaling under fluctuating loads.
Let's take a look at how to build and document APIs that make real-time data streams both powerful and accessible for developers who need immediate responsiveness in their applications.
- Mastering the Fundamentals: Core Concepts That Power Real-Time APIs
- Crafting Developer-Friendly Experiences: Designing Your Real-Time API
- Building Reliable Foundations: Setting Up the Server Side
- Delivering Data to Clients: Client-Side Implementation
- Bringing It All Together
Mastering the Fundamentals: Core Concepts That Power Real-Time APIs
Before diving into implementation details, understanding the foundational concepts behind real-time data streaming will ensure your API architecture stands on solid ground.
When designing an API for real-time data streams, you're creating a high-performance data highway that handles incredible speeds without compromising reliability. The architecture patterns, data formats, and connection protocols you choose form the backbone of your entire system.
Core Streaming Architecture Patterns
These battle-tested approaches define how data flows through your system:
- Publish-Subscribe (Pub/Sub): Publishers send events to topics without caring who's listening, while subscribers only receive the data they've requested. This pattern excels for dashboards requiring fresh data or notification systems that must capture every event.
- Event Sourcing: Rather than just recording current state, event sourcing saves every change as an immutable sequence. This creates a complete historical record perfect for audit trails and time-travel debugging capabilities.
- Command Query Responsibility Segregation (CQRS): By splitting read and write paths, CQRS optimizes each for its specific purpose. Write operations focus on consistency while read operations prioritize speed—crucial for real-time data delivery.
Data Formats Optimized for Streaming
Your format choice significantly impacts performance:
- Avro: This binary format includes schema definitions with the data, handling evolving schemas elegantly. Avro pairs exceptionally well with Kafka for efficient, compact streaming.
- Protocol Buffers (Protobuf): Google's binary format delivers unmatched speed and minimal size. When latency is your primary concern, Protobuf offers the smallest payload and fastest serialization available.
- JSON: While less efficient than binary formats, JSON's human-readability and universal support make it valuable for debugging and web client integration. Just be prepared for the performance trade-off.
Connection Protocols for Real-Time Data Streams
These protocols determine how clients stay connected to your data stream:
WebSockets: Creating persistent, full-duplex channels over a single TCP connection, WebSockets excel for applications requiring two-way communication like chat or collaborative tools.
Server-Sent Events (SSE): Perfect for one-way server-to-client updates, SSE offers simplicity and broad browser support for news feeds, stock tickers, and similar applications.
WebRTC: Enabling direct client-to-client communication, WebRTC eliminates the server middleman for peer-to-peer data streaming applications.
Utilizing a hosted API gateway can simplify the management of these protocols, providing benefits such as scalability, security, and ease of deployment.
Stateful vs. Stateless Processing
This fundamental choice affects how your system handles data context:
- Stateless Processing: Processing each data piece in isolation allows horizontal scaling and simple failure recovery but limits analytical capabilities.
- Stateful Processing: Maintaining context across multiple events enables windowed aggregations, cross-stream joins, and pattern detection, though it adds complexity to scaling and recovery.
Event Time vs. Processing Time
Time concepts create critical distinctions in real-time systems:
- Event Time: When events actually occurred at the source—essential for accurate analytics but challenging with out-of-order arrivals and delayed data.
- Processing Time: When your system processes the event—simpler to implement but potentially misleading when events arrive with varying delays.
With these foundational concepts clarified, you're equipped to make architecture choices that balance performance, reliability, and developer experience in your real-time data stream APIs.
Crafting Developer-Friendly Experiences: Designing Your Real-Time API
Creating an exceptional API for real-time data isn't just about moving bits quickly—it's about crafting interfaces that developers genuinely want to use while maintaining ironclad security and predictable performance.
Security, rate management, and clear documentation form the tripod supporting successful real-time APIs. Let's examine how to implement these critical elements effectively.
Authentication and Authorization Strategies
Securing continuous connections requires approaches that balance protection with performance.
- Token-Based Authentication: JSON Web Tokens (JWT) shine for real-time authentication. They validate without database lookups and carry necessary user information within the token itself. Always implement appropriate expiration times to prevent security vulnerabilities. to monitor and manage permissions effectively.
- Multi-Factor Authentication (MFA): For streams carrying sensitive financial or healthcare information, implementing MFA verifies user identity through multiple channels before establishing continuous connections.
- OAuth 2.0 with Refresh Tokens: Ideal for long-running sessions, this approach allows applications to refresh access tokens without forcing users to repeatedly authenticate—maintaining seamless experiences.
For WebSocket connections, authenticate during the initial handshake and maintain that authentication state throughout the session, eliminating the need to validate every message.
Rate Limiting and Throttling
Without traffic controls, your real-time API can quickly become overwhelmed:
- Token Bucket Algorithm: This approach allows for natural traffic bursts while maintaining overall limits over time—matching real-world usage patterns that rarely follow perfectly consistent intervals.
- Dynamic Throttling: Adjust rate restrictions based on server load, reducing throughput for non-critical clients during peak times while maintaining service levels for priority connections.
- Client Identification: Track usage by API key, IP address, or user ID to ensure fair resource allocation and prevent individual clients from monopolizing system capacity.
- Graceful Degradation: When clients exceed thresholds, reduce update frequency rather than terminating connections completely. This provides a smoother user experience while still protecting system resources.
Implementing these strategies alongside API monitoring tools can help you maintain optimal performance and quickly respond to issues.
API Versioning in Streaming Contexts
Long-lived connections require special versioning considerations:
-
URL Path Versioning: Include the version directly in your connection URL
(e.g.,
/v1/stream/market-data
) for explicit, unambiguous version identification. - Header-Based Versioning: For WebSocket connections, pass version information in connection headers to maintain clean URLs while preserving explicit version control.
- Gradual Deprecation: Allow older API versions to continue functioning with reduced features while encouraging migration to newer versions. Abrupt changes lead to frustrated developers and broken applications.
- Version Negotiation: Implement handshake protocols where clients and servers agree on protocol versions during connection establishment, preventing compatibility surprises.
Async API - The Standards for Real-Time APIs
AsyncAPI is quickly emerging as the defacto standard for describing all non-REST APIs (with OpenAPI being the standard for REST APIs). If you're already familiar with OpenAPI, here's a quick overview of AsyncAPI and analogous properties:
| Concept / Property | AsyncAPI 3.0 | OpenAPI 3.1+ | | -------------------------------- | ------------------------------------------------------------------- | ------------------------------------------------------------- | | Spec Purpose | Event-driven APIs (WebSockets, MQTT, Kafka, SSE, etc.) | Request-response APIs over HTTP/HTTPS | | Top-Level Version | asyncapi: "3.0.0"
| openapi: "3.1.0"
| | Info Object | info
(title, version, description, etc.) | Same | | Servers | servers
(with protocol-specific fields like host, protocol, path) | Same, though focused on HTTP URL and variables | | Operations | operations
block with send
/ receive
actions | Defined inline under paths
with get
, post
, etc. | | Channels / Paths | channels
= logical topics or stream endpoints (e.g. /chat
) | paths
= HTTP paths (e.g. /users/{id}
) | | Messages vs Requests | messages
: standalone message definitions (for publish/subscribe) | requestBody
and responses
for HTTP requests/responses | | Payload Schema | payload
(JSON Schema, Avro, etc.) | schema
(JSON Schema-based for requests/responses) | | Actions | send
, receive
, and reply
(new in v3) | HTTP methods (get
, post
, etc.) define intent | | Protocols Supported | WebSockets, MQTT, Kafka, AMQP, SSE, Redis Streams, NATS, etc. | HTTP/HTTPS | | Bindings (Protocol Metadata) | Yes (bindings
object for channels, operations, messages) | Not applicable — protocol is standardized as HTTP | | Reusable Components | components
: messages, schemas, securitySchemes, etc. | components
: schemas, parameters, responses, securitySchemes | | Security Schemes | Yes (e.g. API key, OAuth2, etc.) | Same | | Links / Relationships | Under development (planned in v3.1+) | links
for describing response relationships | | Extensions | x-
prefix extensions supported | Same | | Codegen & Tooling Support | Growing: CLI, Studio, Generator, Parsers | Mature: Zudoku, Swagger UI, Stoplight, etc. | | Visual Documentation | AsyncAPI Studio, HTML docs generator | Zudoku, Swagger UI, Rapidoc | | Request-Reply Pattern | Explicit in v3 using reply
action | Modeled using multiple endpoints manually | | Workflow Modeling | Better for pub/sub or streaming pipelines | Better for RESTful workflows with verbs |
Fundamentally AsyncAPI is channel-first - it defines how data flows via topics, events, or message brokers. This is in contrast to OpenAPI which is resource-first. Now, let's get into some examples to see AsyncAPI 3.0 in action.