Agentic AI vs Traditional AI: Why Autonomy is the Next Frontier
Artificial intelligence has developed from a set of rapid rules-based programs, which are able to learn, adopt and generate new content. Today, a new form of AI is emerging which further further borders - agent AI. Unlike traditional AI, which often follows pre-defined instructions and depends a lot on human input, agent AI works with high level autonomy, decision making and target-oriented. AI as businesses, teachers and tech developers detect the next stage of development, it becomes important to understand the change in the agentic system from the traditional model. What is agent AI? Agent AI refers to systems that can initiate action, take independent decisions, and manage complex goals over time. These systems are designed to be active rather than reactive. They are not just equipment that users respond to command - they are more like assistants with their own understanding of objectives. For example, an agent AI can be tasked with improving customer service in a retail company. Instead of waiting for input, it can analyze the customer questions, update its own training data, adapt its communication style, and even recommend new products or services based on the future insight. Traditional AI, by contrast, works well for specific functions, but this adaptive, target-oriented flexibility. This requires frequent updates, training, and often cannot be effectively operated outside its predetermined scope. Affection technology behind autonomy Agent AI systems manufacture on many advanced technologies: Language understanding of language and large language models like GPT-4 and Gemini for generations. Learning reinforcement for decision making and reward optimization. Cognitive architecture that mimics human-like logic and memory structures. Multi-agent systems, where many intelligent agents collaborate or compete to complete complex tasks. These technologies allow agent AI to set up the sub-goal, learn from results and refine strategies-similar to a human decision-maker. Real world application In 2025, we are already looking at the initial implementation of agent AI in areas: Healthcare: Autonomous agents can manage patient data, perform follow -up schedule, and suggest initial diagnosis strategies based on the patient's history. Finance: Agents have started helping the system portfolio management, detection of fraud and even following economic trends. Education: Smart learning agents can personalize students, identify gaps, and recommend the paths of learning without direct human supervision. Customer Experience: AI agents in call center are not only answering questions, but also starting negotiations on the basis of future analytics. Why does autonomy now matters The demand for real -time decision making in the rapidly growing digital environment is increasing. Traditional AI bus cannot keep data in areas such as cyber security, logistics and financial trade with the quantity and speed of data. Agent AI fills this difference by operating with low dependence on human intervention. It does not only follow the instructions - it understands the intentions behind them and adopts strategies accordingly. This increased autonomy also brings scalability. Businesses can deploy hundreds of agent systems to handle various operations - from customer engagement to backand logistics - from every learning and adopting independently. Risk and moral challenges More responsibility comes with more power. Autonomous AI introduces complex moral challenges: Accountability: If an AI agent acts independently and causes damage, who is responsible? Prejudice and fairness: Like all AI systems, agentic models can inherit or increase prejudices if they are not trained on various, representative data. Security: Highly autonomous agents can become aiming for adverse attacks, or worse, if the obstacles are not well defined, act unexpectedly. Ensuring confidence in agent AI means developing transparent models, continuous monitoring systems and clear moral guidelines. Latest developments In Openai's recent 2025 report, the team introduced a new "autogept" prototype, capable of operating complex research functions on the Internet, which manages several stages of logic without the need for continuous signs. Google Deepmind also unveiled a multi-agent system earlier this year, showing autonomous cooperation in a simulated environment-Given how agent systems can manage disaster relief logistics or space exploration mission someday. These developments confirm that agent AI is not a distant future concept - this is an emerging current. What about human roles? An important fear is job displacement around the agent AI. But experts suggest more nuances. Agentic systems are equipment for growth, not replacement. They handle repetition, data-heavy tasks while humans focus on creativity, sympathy and strategic thinking. Organizations that embrace this collaborative model will gain competitive lead in the coming years. Concl
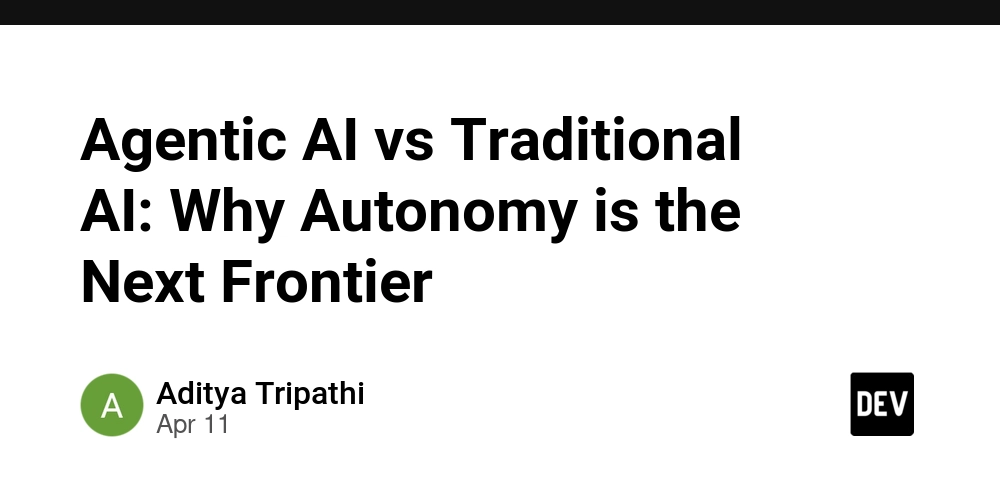
Artificial intelligence has developed from a set of rapid rules-based programs, which are able to learn, adopt and generate new content. Today, a new form of AI is emerging which further further borders - agent AI. Unlike traditional AI, which often follows pre-defined instructions and depends a lot on human input, agent AI works with high level autonomy, decision making and target-oriented.
AI as businesses, teachers and tech developers detect the next stage of development, it becomes important to understand the change in the agentic system from the traditional model.
What is agent AI?
Agent AI refers to systems that can initiate action, take independent decisions, and manage complex goals over time. These systems are designed to be active rather than reactive. They are not just equipment that users respond to command - they are more like assistants with their own understanding of objectives.
For example, an agent AI can be tasked with improving customer service in a retail company. Instead of waiting for input, it can analyze the customer questions, update its own training data, adapt its communication style, and even recommend new products or services based on the future insight.
Traditional AI, by contrast, works well for specific functions, but this adaptive, target-oriented flexibility. This requires frequent updates, training, and often cannot be effectively operated outside its predetermined scope.
Affection technology behind autonomy
Agent AI systems manufacture on many advanced technologies:
Language understanding of language and large language models like GPT-4 and Gemini for generations.
Learning reinforcement for decision making and reward optimization.
Cognitive architecture that mimics human-like logic and memory structures.
Multi-agent systems, where many intelligent agents collaborate or compete to complete complex tasks.
These technologies allow agent AI to set up the sub-goal, learn from results and refine strategies-similar to a human decision-maker.
Real world application
In 2025, we are already looking at the initial implementation of agent AI in areas:
Healthcare: Autonomous agents can manage patient data, perform follow -up schedule, and suggest initial diagnosis strategies based on the patient's history.
Finance: Agents have started helping the system portfolio management, detection of fraud and even following economic trends.
Education: Smart learning agents can personalize students, identify gaps, and recommend the paths of learning without direct human supervision.
Customer Experience: AI agents in call center are not only answering questions, but also starting negotiations on the basis of future analytics.
Why does autonomy now matters
The demand for real -time decision making in the rapidly growing digital environment is increasing. Traditional AI bus cannot keep data in areas such as cyber security, logistics and financial trade with the quantity and speed of data.
Agent AI fills this difference by operating with low dependence on human intervention. It does not only follow the instructions - it understands the intentions behind them and adopts strategies accordingly.
This increased autonomy also brings scalability. Businesses can deploy hundreds of agent systems to handle various operations - from customer engagement to backand logistics - from every learning and adopting independently.
Risk and moral challenges
More responsibility comes with more power. Autonomous AI introduces complex moral challenges:
Accountability: If an AI agent acts independently and causes damage, who is responsible?
Prejudice and fairness: Like all AI systems, agentic models can inherit or increase prejudices if they are not trained on various, representative data.
Security: Highly autonomous agents can become aiming for adverse attacks, or worse, if the obstacles are not well defined, act unexpectedly.
Ensuring confidence in agent AI means developing transparent models, continuous monitoring systems and clear moral guidelines.
Latest developments
In Openai's recent 2025 report, the team introduced a new "autogept" prototype, capable of operating complex research functions on the Internet, which manages several stages of logic without the need for continuous signs.
Google Deepmind also unveiled a multi-agent system earlier this year, showing autonomous cooperation in a simulated environment-Given how agent systems can manage disaster relief logistics or space exploration mission someday.
These developments confirm that agent AI is not a distant future concept - this is an emerging current.
What about human roles?
An important fear is job displacement around the agent AI. But experts suggest more nuances. Agentic systems are equipment for growth, not replacement. They handle repetition, data-heavy tasks while humans focus on creativity, sympathy and strategic thinking.
Organizations that embrace this collaborative model will gain competitive lead in the coming years.
Conclusions: Shift has already started
The rise of agent AI how to manufacture and interact with machines has a big innings. From performing defined tasks to further independent objectives, these systems represent a leap towards intelligent autonomy.
As this frontier spreads, it also creates interest in special teaching programs across the country. For example, the demand for Agentic Ai Course is increasing, which shows the growing attention of the region on innovation and future ready skills. Similarly, the rise of Creative AI Tools has increased interest in programs such as generative AI courses in Thane, which aims to equip students with hand capabilities in the next-gene AI system.
By understanding the agent AI and adopting responsibly, we step into a world where machines are not alone