Teaching AI in 2025: Why We Must Include Its Financial Reality
Just wrapped up a thought-provoking session with leaders from academia and the cloud industry — diving deep into AI, R&D, and the economics behind it all. One of the most important and sobering takeaways? Despite all the hype, most AI companies aren't actually making money — and the only real winners so far are those who power the infrastructure, not those building the models. We discussed how companies like OpenAI are currently on track to spend $9 billion in 2024 while making just $4 billion, with the bulk of their revenue going straight into compute costs. Even paying customers reportedly cost the company more than they bring in. Similarly, Anthropic, backed by giants like Amazon and Google, lost over $5.6 billion last year while generating under a billion in revenue. Stability AI, once a rising star, has faced funding challenges and leadership turbulence. And even newer entrants like Perplexity, despite high valuations, only brought in $56 million last year and remain unprofitable. In stark contrast, Nvidia, a hardware company rather than an AI startup, is thriving. In Q4 of FY24 alone, it reported $22.1 billion in revenue and $12.3 billion in profit, mostly driven by surging demand for its AI-optimized GPUs. Companies like OpenAI, Anthropic, and major cloud providers (AWS, Azure, Google Cloud) are essentially building their entire infrastructures on Nvidia’s chips — making it the backbone of this AI boom. What’s even more concerning is the broader industry picture. Microsoft and Google are pouring tens of billions into AI infrastructure — yet their generative AI products like Copilot and Gemini have relatively small user bases compared to their traditional offerings. Many of these tools are being pushed into enterprise suites more out of pressure to “look futuristic” than real customer demand. Meanwhile, a staggering portion of AI revenue is tied up in subscriptions and cloud credits, not sustainable business models. So what does all of this mean for us — as educators, researchers, and cloud service providers? It’s clear we need to start preparing students and professionals not just to build and use AI, but to deeply understand the economics behind it, the hardware-software balance, and what it truly means to create scalable, sustainable, and valuable technology. The hype is loud — but the numbers are louder. This conversation isn’t over. In fact, it’s just beginning.
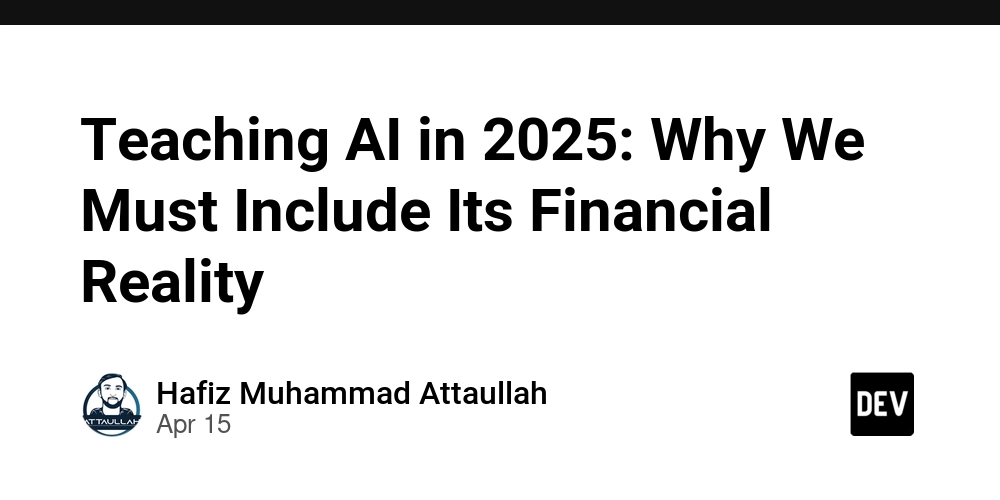
Just wrapped up a thought-provoking session with leaders from academia and the cloud industry — diving deep into AI, R&D, and the economics behind it all. One of the most important and sobering takeaways? Despite all the hype, most AI companies aren't actually making money — and the only real winners so far are those who power the infrastructure, not those building the models.
We discussed how companies like OpenAI are currently on track to spend $9 billion in 2024 while making just $4 billion, with the bulk of their revenue going straight into compute costs. Even paying customers reportedly cost the company more than they bring in. Similarly, Anthropic, backed by giants like Amazon and Google, lost over $5.6 billion last year while generating under a billion in revenue. Stability AI, once a rising star, has faced funding challenges and leadership turbulence. And even newer entrants like Perplexity, despite high valuations, only brought in $56 million last year and remain unprofitable.
In stark contrast, Nvidia, a hardware company rather than an AI startup, is thriving. In Q4 of FY24 alone, it reported $22.1 billion in revenue and $12.3 billion in profit, mostly driven by surging demand for its AI-optimized GPUs. Companies like OpenAI, Anthropic, and major cloud providers (AWS, Azure, Google Cloud) are essentially building their entire infrastructures on Nvidia’s chips — making it the backbone of this AI boom.
What’s even more concerning is the broader industry picture. Microsoft and Google are pouring tens of billions into AI infrastructure — yet their generative AI products like Copilot and Gemini have relatively small user bases compared to their traditional offerings. Many of these tools are being pushed into enterprise suites more out of pressure to “look futuristic” than real customer demand. Meanwhile, a staggering portion of AI revenue is tied up in subscriptions and cloud credits, not sustainable business models.
So what does all of this mean for us — as educators, researchers, and cloud service providers? It’s clear we need to start preparing students and professionals not just to build and use AI, but to deeply understand the economics behind it, the hardware-software balance, and what it truly means to create scalable, sustainable, and valuable technology. The hype is loud — but the numbers are louder.
This conversation isn’t over. In fact, it’s just beginning.