Scikit-learn Essentials for Data science
Introduction Scikit-learn is one of the most popular machine learning libraries for python. It's built on top of NumPy, SciPy, and Matplotlib, making it an efficient and user-friendly toolkit for data analysis, predictive modeling and AI-driven applications. Key Features of Scikit-learn: Simple and efficient tools for data mining and analysis. Built-in algorithms for classification, regression, clustering and more. Support for preprocessing tasks like feature selection, normalization and dimensionality reduction. Extensive documentation and active community to help developers and data scientists. Code import numpy as np import pandas as pd from sklearn.model_selection import train_test_split from sklearn.preprocessing import StandardScaler from sklearn.linear_model import LogisticRegression from sklearn.metrics import accuracy_score # Step 1: Generate Sample Data np.random.seed(42) X = np.random.rand(100, 2) # 100 samples, 2 features y = (X[:, 0] + X[:, 1] > 1).astype(int) # Labels based on sum of features # Step 2: Split the Data into Training and Testing Sets X_train, X_test, y_train, y_test = train_test_split(X, y, test_size=0.2, random_state=42) # Step 3: Standardize the Features scaler = StandardScaler() X_train_scaled = scaler.fit_transform(X_train) X_test_scaled = scaler.transform(X_test) # Step 4: Train the Logistic Regression Model model = LogisticRegression() model.fit(X_train_scaled, y_train) # Step 5: Make Predictions y_pred = model.predict(X_test_scaled) # Step 6: Evaluate the Model accuracy = accuracy_score(y_test, y_pred) print(f"Model Accuracy: {accuracy:.2f}") Explanation: Data Generation: We create random data points and define labels based on a simple rule. Splitting the Dataset: The dataset is divided into training(80%) and testing(20%) parts. Feature Scaling: Standardizing features helps improve the performance of many models. Model Training: We use logistic Regression, a popular algorithm for binary classification. Prediction: After training, the model predicts labels for the test data. Evaluation: We measure how well the model performs using accuracy score.
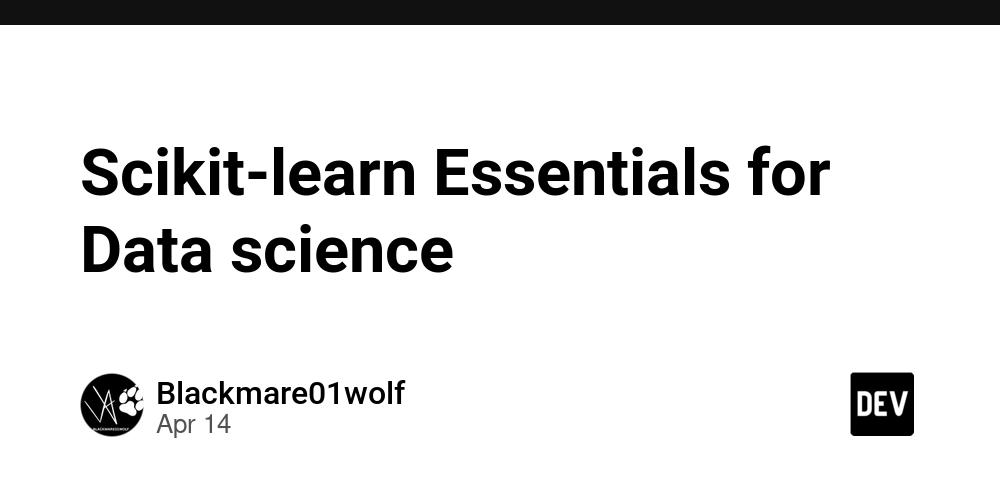
Introduction
Scikit-learn is one of the most popular machine learning libraries for python. It's built on top of NumPy, SciPy, and Matplotlib, making it an efficient and user-friendly toolkit for data analysis, predictive modeling and AI-driven applications.
Key Features of Scikit-learn:
- Simple and efficient tools for data mining and analysis.
- Built-in algorithms for classification, regression, clustering and more.
- Support for preprocessing tasks like feature selection, normalization and dimensionality reduction.
- Extensive documentation and active community to help developers and data scientists.
Code
import numpy as np
import pandas as pd
from sklearn.model_selection import train_test_split
from sklearn.preprocessing import StandardScaler
from sklearn.linear_model import LogisticRegression
from sklearn.metrics import accuracy_score
# Step 1: Generate Sample Data
np.random.seed(42)
X = np.random.rand(100, 2) # 100 samples, 2 features
y = (X[:, 0] + X[:, 1] > 1).astype(int) # Labels based on sum of features
# Step 2: Split the Data into Training and Testing Sets
X_train, X_test, y_train, y_test = train_test_split(X, y, test_size=0.2, random_state=42)
# Step 3: Standardize the Features
scaler = StandardScaler()
X_train_scaled = scaler.fit_transform(X_train)
X_test_scaled = scaler.transform(X_test)
# Step 4: Train the Logistic Regression Model
model = LogisticRegression()
model.fit(X_train_scaled, y_train)
# Step 5: Make Predictions
y_pred = model.predict(X_test_scaled)
# Step 6: Evaluate the Model
accuracy = accuracy_score(y_test, y_pred)
print(f"Model Accuracy: {accuracy:.2f}")
Explanation:
- Data Generation: We create random data points and define labels based on a simple rule.
- Splitting the Dataset: The dataset is divided into training(80%) and testing(20%) parts.
- Feature Scaling: Standardizing features helps improve the performance of many models.
- Model Training: We use logistic Regression, a popular algorithm for binary classification.
- Prediction: After training, the model predicts labels for the test data.
- Evaluation: We measure how well the model performs using accuracy score.