KPMG’s AI Report Exposes Critical AI Challenges
85% of enterprise leaders view data quality as their #1 AI challenge, according to KPMG. Yet most continue using traditional databases that obscure critical relationships in data. Still wondering why only 12% of enterprises actually launch AI agents despite heavy pilots? We’ve talked to dozens of CTOs and VPs of Engineering about scaling AI projects, and here’s what’s clear: 1.Data Quality: Explicit graph representations surface hidden relationships, solving foundational accuracy problems missed by tabular schemas. 2.Security & Privacy: Tenant isolation within multi-tenant architectures directly secures sensitive data at the node level—no external governance layers needed. 3.Trust & Explainability: Graph-based retrieval provides explicit audit trails, ensuring AI outputs are transparent and defensible. *Key takeaway: If you’re still relying on incremental database tweaks, you’re addressing symptoms, not root issues. *
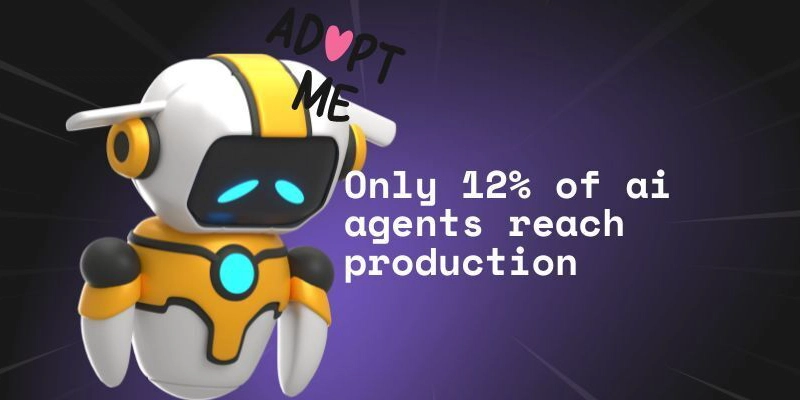
85% of enterprise leaders view data quality as their #1 AI challenge, according to KPMG.
Yet most continue using traditional databases that obscure critical relationships in data.
Still wondering why only 12% of enterprises actually launch AI agents despite heavy pilots?
We’ve talked to dozens of CTOs and VPs of Engineering about scaling AI projects, and here’s what’s clear:
1.Data Quality:
Explicit graph representations surface hidden relationships, solving foundational accuracy problems missed by tabular schemas.
2.Security & Privacy:
Tenant isolation within multi-tenant architectures directly secures sensitive data at the node level—no external governance layers needed.
3.Trust & Explainability:
Graph-based retrieval provides explicit audit trails, ensuring AI outputs are transparent and defensible.
*Key takeaway: If you’re still relying on incremental database tweaks, you’re addressing symptoms, not root issues.
*