From Generative AI Proof of Concept to Full-Scale Innovation
Completing a generative ai proof of concept can yield exciting insights and quick wins, but it’s only the first chapter in harnessing AI’s creative power. Turning that pilot into an enterprise-wide or customer-facing solution requires robust planning, data optimization, and scaled deployment strategies. This blog explores how to transition from a successful pilot to broad-based innovation, ensuring the technology and cultural pillars line up for longevity and transformative impact. 1. Reflecting on Pilot Outcomes 1.1 Achieved Metrics Did the pilot surpass your set success metrics—like generating content in half the usual time or achieving consistent brand voice alignment? Celebrate these achievements and gather them as data points for a broader rollout. 1.2 Identified Gaps Analyze any shortfalls—like suboptimal model accuracy or user confusion. Address these proactively via better data, more training, or refined prompts to ensure the final release meets higher standards. 2. Strengthening Data and Infrastructure 2.1 Data Pipeline Enhancements Scaling to an enterprise solution typically involves bigger datasets and possibly real-time feeds. Expand your pipeline to handle continuous data input (like new brand guidelines or updated product lines) and integrate robust data validation checks. 2.2 Infrastructure Sizing During the pilot, GPU or CPU usage might have been modest. Full-scale adoption may demand advanced containers, orchestration (Kubernetes), or multi-region setups. Make cost governance part of the plan—paying for idle GPU clusters can inflate monthly bills. 2.3 DevOps and MLOps Integration To maintain frequent model updates without downtime, incorporate continuous integration/continuous deployment (CI/CD) pipelines tailored for machine learning. “MLOps” frameworks automate model retraining, versioning, and performance monitoring, supporting agile improvements. 3. Organizational Buy-In and Adoption 3.1 Leadership Support Showcasing pilot success to C-level or department heads helps secure the budget for upscaled generative AI. Summarize stats, ROI, or user testimonials to highlight broad benefits. 3.2 Cross-Functional Collaboration Enterprise expansion might require input from marketing, compliance, HR, or even external partners. Early alignment ensures each group’s concerns—like brand tone or data privacy—are addressed. 3.3 Culture of Experimentation Encourage employees to propose new generative AI use cases. This fosters a sense of shared ownership and organically identifies high-impact expansions. 4. Evolving the Model for Larger Impact 4.1 Fine-Tuning for More Domains Initial success might revolve around marketing copy creation. Future expansions can address product design, internal documentation summarization, or user support knowledge bases. Fine-tune the model with additional domain-specific data sets. 4.2 Enhancing Prompt Engineering Complex scenarios—for instance, multi-lingual content generation—demand more advanced prompts. By refining guidelines or examples, you can steer the AI toward context-rich, brand-aligned outputs. 4.3 Advanced Features Consider real-time analytics or sentiment analysis layers. This ensures generative outputs adapt to user mood, brand changes, or new product lines on the fly. 5. Ensuring Robust Performance and Security 5.1 Monitoring & Alerting In a live environment, unexpected spikes in user queries can cause latency or degrade output quality. Set real-time alerts for response times, memory usage, or content error rates. 5.2 Bias Detection Generative models can inadvertently produce biased or offensive content. Regular audits, layered editorial reviews, and robust training data curation guard your brand’s reputation. 5.3 Privacy and Compliance Ensure the final solution meets local or industry regulations—like ensuring personal user data is anonymized or encrypting sensitive textual data at rest and in transit. 6. Measuring Ongoing ROI Even post-deployment, measure improvements: Productivity Gains: Time employees save or cycles shortened for content creation or R&D tasks. User Engagement: If the AI generates customer-facing materials, track user response, brand loyalty, or conversion metrics. Quality and Consistency: Are outputs consistently in line with brand voice, accuracy, and legal compliance? These KPIs inform iterative model updates and expansions into new areas. 7. Future Directions: Beyond Standard Generation As generative AI matures, organizations can explore: Agentic Systems: AI that not only generates content but sets sub-goals, orchestrating multi-step tasks with minimal supervision. Multi-Modal Integration: Combine textual generation with image creation or voice synthesis, enabling richer user experiences. Real-Time Personalization: Solutions that adapt to each user’s context or preferences, fueling more dynamic marketing or product designs. C
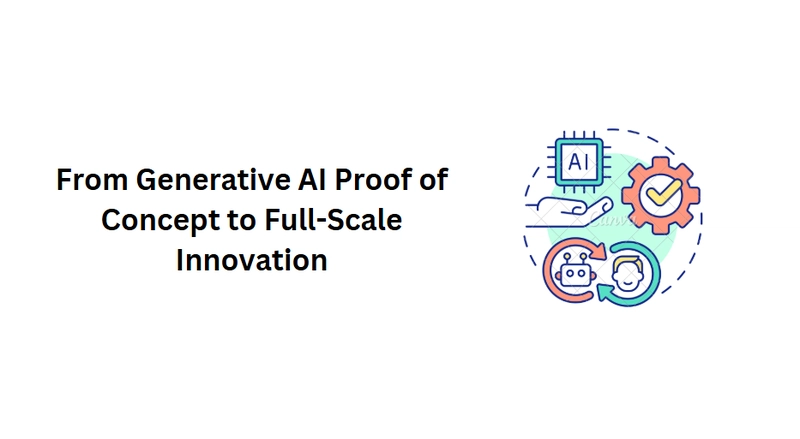
Completing a generative ai proof of concept can yield exciting insights and quick wins, but it’s only the first chapter in harnessing AI’s creative power. Turning that pilot into an enterprise-wide or customer-facing solution requires robust planning, data optimization, and scaled deployment strategies. This blog explores how to transition from a successful pilot to broad-based innovation, ensuring the technology and cultural pillars line up for longevity and transformative impact.
1. Reflecting on Pilot Outcomes
1.1 Achieved Metrics
Did the pilot surpass your set success metrics—like generating content in half the usual time or achieving consistent brand voice alignment? Celebrate these achievements and gather them as data points for a broader rollout.
1.2 Identified Gaps
Analyze any shortfalls—like suboptimal model accuracy or user confusion. Address these proactively via better data, more training, or refined prompts to ensure the final release meets higher standards.
2. Strengthening Data and Infrastructure
2.1 Data Pipeline Enhancements
Scaling to an enterprise solution typically involves bigger datasets and possibly real-time feeds. Expand your pipeline to handle continuous data input (like new brand guidelines or updated product lines) and integrate robust data validation checks.
2.2 Infrastructure Sizing
During the pilot, GPU or CPU usage might have been modest. Full-scale adoption may demand advanced containers, orchestration (Kubernetes), or multi-region setups. Make cost governance part of the plan—paying for idle GPU clusters can inflate monthly bills.
2.3 DevOps and MLOps Integration
To maintain frequent model updates without downtime, incorporate continuous integration/continuous deployment (CI/CD) pipelines tailored for machine learning. “MLOps” frameworks automate model retraining, versioning, and performance monitoring, supporting agile improvements.
3. Organizational Buy-In and Adoption
3.1 Leadership Support
Showcasing pilot success to C-level or department heads helps secure the budget for upscaled generative AI. Summarize stats, ROI, or user testimonials to highlight broad benefits.
3.2 Cross-Functional Collaboration
Enterprise expansion might require input from marketing, compliance, HR, or even external partners. Early alignment ensures each group’s concerns—like brand tone or data privacy—are addressed.
3.3 Culture of Experimentation
Encourage employees to propose new generative AI use cases. This fosters a sense of shared ownership and organically identifies high-impact expansions.
4. Evolving the Model for Larger Impact
4.1 Fine-Tuning for More Domains
Initial success might revolve around marketing copy creation. Future expansions can address product design, internal documentation summarization, or user support knowledge bases. Fine-tune the model with additional domain-specific data sets.
4.2 Enhancing Prompt Engineering
Complex scenarios—for instance, multi-lingual content generation—demand more advanced prompts. By refining guidelines or examples, you can steer the AI toward context-rich, brand-aligned outputs.
4.3 Advanced Features
Consider real-time analytics or sentiment analysis layers. This ensures generative outputs adapt to user mood, brand changes, or new product lines on the fly.
5. Ensuring Robust Performance and Security
5.1 Monitoring & Alerting
In a live environment, unexpected spikes in user queries can cause latency or degrade output quality. Set real-time alerts for response times, memory usage, or content error rates.
5.2 Bias Detection
Generative models can inadvertently produce biased or offensive content. Regular audits, layered editorial reviews, and robust training data curation guard your brand’s reputation.
5.3 Privacy and Compliance
Ensure the final solution meets local or industry regulations—like ensuring personal user data is anonymized or encrypting sensitive textual data at rest and in transit.
6. Measuring Ongoing ROI
Even post-deployment, measure improvements:
Productivity Gains: Time employees save or cycles shortened for content creation or R&D tasks.
User Engagement: If the AI generates customer-facing materials, track user response, brand loyalty, or conversion metrics.
Quality and Consistency: Are outputs consistently in line with brand voice, accuracy, and legal compliance?
These KPIs inform iterative model updates and expansions into new areas.
7. Future Directions: Beyond Standard Generation
As generative AI matures, organizations can explore:
Agentic Systems: AI that not only generates content but sets sub-goals, orchestrating multi-step tasks with minimal supervision.
Multi-Modal Integration: Combine textual generation with image creation or voice synthesis, enabling richer user experiences.
Real-Time Personalization: Solutions that adapt to each user’s context or preferences, fueling more dynamic marketing or product designs.
Conclusion
Completing a generative ai proof of concept is the first leap in a broader journey. Going from pilot to full-scale adoption involves scaling data flows, upgrading infrastructure, and building organizational readiness. With thorough planning, cultural alignment, and a focus on robust performance and compliance, your generative AI solution can become a linchpin for enterprise-wide creativity, efficiency, and strategic advantage. By treating the pilot’s insights as a launching pad rather than an endpoint, businesses can unlock even greater transformative potential in this rapidly evolving AI landscape.