Building AI Agents with Google ADK, FastAPI, and MCP
This guide will walk you through integrating Google's Agent Development Kit (ADK) with FastAPI and the Model Context Protocol (MCP). We'll start with basic ADK usage, then extend it to work with FastAPI, and finally create an MCP server from the same codebase. What You'll Learn How to build a basic ADK agent with tools How to serve your ADK agent using FastAPI How to expose your ADK tools through an MCP server Best practices for each integration pattern Prerequisites You'll need: Python 3.10+ installed Access to Google's Gemini API (or another supported model) Basic knowledge of Python and APIs Part 1: Getting Started with Google ADK The Google Agent Development Kit (ADK) is a framework for building AI agents with tool-using capabilities. Installation pip install google-adk python-dotenv Creating a Basic Agent Let's create a simple agent with two tools: one to check the weather and another to get the current time in a city. First, create a directory structure: adk_agents/ ├── multi_tool_agent/ │ ├── __init__.py │ └── agent.py └── __init__.py Define your agent in multi_tool_agent/agent.py: import datetime from zoneinfo import ZoneInfo from google.adk.agents import Agent def get_weather(city: str) -> dict: """Get the current weather in a city.""" if city.lower() == "new york": return { "status": "success", "report": "The weather in New York is sunny with 25°C." } return { "status": "error", "error_message": f"Weather for '{city}' unavailable." } def get_current_time(city: str) -> dict: """Get the current time in a city.""" city_timezones = { "new york": "America/New_York", "london": "Europe/London", "tokyo": "Asia/Tokyo", "paris": "Europe/Paris" } if city.lower() in city_timezones: try: tz = ZoneInfo(city_timezones[city.lower()]) now = datetime.datetime.now(tz) return { "status": "success", "report": f"The current time in {city} is {now.strftime('%Y-%m-%d %H:%M:%S %Z')}" } except Exception: pass return { "status": "error", "error_message": f"Time information for '{city}' unavailable." } # Define the agent with the name "root_agent" (required by ADK) root_agent = Agent( name="weather_time_agent", model="gemini-1.5-flash", # Use your preferred Gemini model description="Agent that provides weather and time information for cities.", instruction="You help users with time and weather information for various cities.", tools=[get_weather, get_current_time], ) Make sure to export the agent in multi_tool_agent/__init__.py: from .agent import root_agent __all__ = ["root_agent"] Testing Your Agent Run the agent using the ADK CLI: cd adk_agents adk chat -a multi_tool_agent If you want to test the GUI: cd adk_agents adk web -a multi_tool_agent Part 2: Integrating with FastAPI Now let's wrap our ADK agent in a FastAPI application, which makes it deployable as a web service. Installation pip install fastapi "uvicorn[standard]" sqlalchemy Creating the FastAPI Wrapper Create api.py in your root directory: import os import sys import uvicorn from fastapi import FastAPI from google.adk.cli.fast_api import get_fast_api_app from dotenv import load_dotenv # Set up paths BASE_DIR = os.path.abspath(os.path.dirname(__file__)) AGENT_DIR = BASE_DIR # Parent directory containing multi_tool_agent # Set up DB path for sessions SESSION_DB_URL = f"sqlite:///{os.path.join(BASE_DIR, 'sessions.db')}" # Create the FastAPI app using ADK's helper app: FastAPI = get_fast_api_app( agent_dir=AGENT_DIR, session_db_url=SESSION_DB_URL, allow_origins=["*"], # In production, restrict this web=True, # Enable the ADK Web UI ) # Add custom endpoints @app.get("/health") async def health_check(): return {"status": "healthy"} @app.get("/agent-info") async def agent_info(): """Provide agent information""" from multi_tool_agent import root_agent return { "agent_name": root_agent.name, "description": root_agent.description, "model": root_agent.model, "tools": [t.__name__ for t in root_agent.tools] } if __name__ == "__main__": print("Starting FastAPI server...") uvicorn.run( app, host="0.0.0.0", port=9999, reload=False ) Running the FastAPI Server python api.py Access the ADK Web UI at http://localhost:9999/dev-ui Part 3: Creating an MCP Server The Model Context Protocol (MCP) allows AI models to discover and use tools. Let's expose our tools via MCP. Installation pip install mcp Adding MCP Server Support Add the
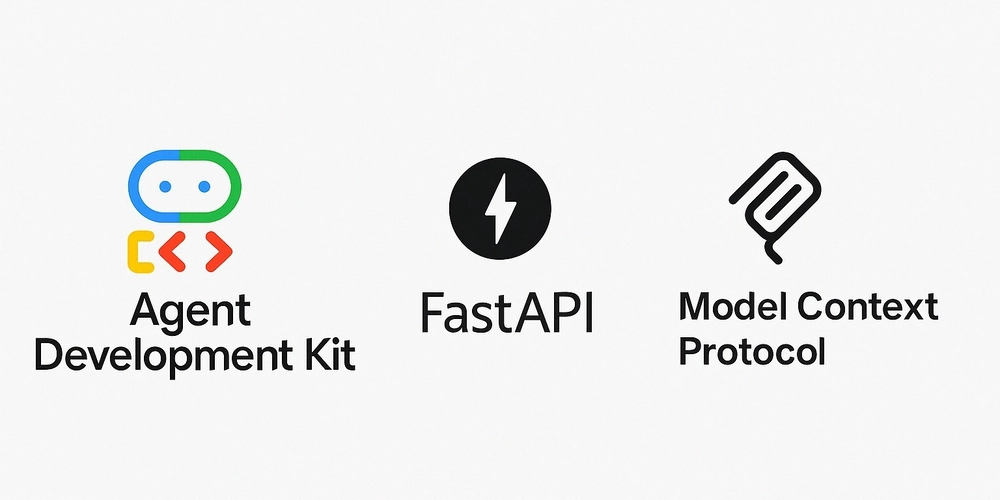
This guide will walk you through integrating Google's Agent Development Kit (ADK) with FastAPI and the Model Context Protocol (MCP). We'll start with basic ADK usage, then extend it to work with FastAPI, and finally create an MCP server from the same codebase.
What You'll Learn
- How to build a basic ADK agent with tools
- How to serve your ADK agent using FastAPI
- How to expose your ADK tools through an MCP server
- Best practices for each integration pattern
Prerequisites
You'll need:
- Python 3.10+ installed
- Access to Google's Gemini API (or another supported model)
- Basic knowledge of Python and APIs
Part 1: Getting Started with Google ADK
The Google Agent Development Kit (ADK) is a framework for building AI agents with tool-using capabilities.
Installation
pip install google-adk python-dotenv
Creating a Basic Agent
Let's create a simple agent with two tools: one to check the weather and another to get the current time in a city.
First, create a directory structure:
adk_agents/
├── multi_tool_agent/
│ ├── __init__.py
│ └── agent.py
└── __init__.py
Define your agent in multi_tool_agent/agent.py
:
import datetime
from zoneinfo import ZoneInfo
from google.adk.agents import Agent
def get_weather(city: str) -> dict:
"""Get the current weather in a city."""
if city.lower() == "new york":
return {
"status": "success",
"report": "The weather in New York is sunny with 25°C."
}
return {
"status": "error",
"error_message": f"Weather for '{city}' unavailable."
}
def get_current_time(city: str) -> dict:
"""Get the current time in a city."""
city_timezones = {
"new york": "America/New_York",
"london": "Europe/London",
"tokyo": "Asia/Tokyo",
"paris": "Europe/Paris"
}
if city.lower() in city_timezones:
try:
tz = ZoneInfo(city_timezones[city.lower()])
now = datetime.datetime.now(tz)
return {
"status": "success",
"report": f"The current time in {city} is {now.strftime('%Y-%m-%d %H:%M:%S %Z')}"
}
except Exception:
pass
return {
"status": "error",
"error_message": f"Time information for '{city}' unavailable."
}
# Define the agent with the name "root_agent" (required by ADK)
root_agent = Agent(
name="weather_time_agent",
model="gemini-1.5-flash", # Use your preferred Gemini model
description="Agent that provides weather and time information for cities.",
instruction="You help users with time and weather information for various cities.",
tools=[get_weather, get_current_time],
)
Make sure to export the agent in multi_tool_agent/__init__.py
:
from .agent import root_agent
__all__ = ["root_agent"]
Testing Your Agent
Run the agent using the ADK CLI:
cd adk_agents
adk chat -a multi_tool_agent
If you want to test the GUI:
cd adk_agents
adk web -a multi_tool_agent
Part 2: Integrating with FastAPI
Now let's wrap our ADK agent in a FastAPI application, which makes it deployable as a web service.
Installation
pip install fastapi "uvicorn[standard]" sqlalchemy
Creating the FastAPI Wrapper
Create api.py
in your root directory:
import os
import sys
import uvicorn
from fastapi import FastAPI
from google.adk.cli.fast_api import get_fast_api_app
from dotenv import load_dotenv
# Set up paths
BASE_DIR = os.path.abspath(os.path.dirname(__file__))
AGENT_DIR = BASE_DIR # Parent directory containing multi_tool_agent
# Set up DB path for sessions
SESSION_DB_URL = f"sqlite:///{os.path.join(BASE_DIR, 'sessions.db')}"
# Create the FastAPI app using ADK's helper
app: FastAPI = get_fast_api_app(
agent_dir=AGENT_DIR,
session_db_url=SESSION_DB_URL,
allow_origins=["*"], # In production, restrict this
web=True, # Enable the ADK Web UI
)
# Add custom endpoints
@app.get("/health")
async def health_check():
return {"status": "healthy"}
@app.get("/agent-info")
async def agent_info():
"""Provide agent information"""
from multi_tool_agent import root_agent
return {
"agent_name": root_agent.name,
"description": root_agent.description,
"model": root_agent.model,
"tools": [t.__name__ for t in root_agent.tools]
}
if __name__ == "__main__":
print("Starting FastAPI server...")
uvicorn.run(
app,
host="0.0.0.0",
port=9999,
reload=False
)
Running the FastAPI Server
python api.py
Access the ADK Web UI at http://localhost:9999/dev-ui
Part 3: Creating an MCP Server
The Model Context Protocol (MCP) allows AI models to discover and use tools. Let's expose our tools via MCP.
Installation
pip install mcp
Adding MCP Server Support
Add the following code to api.py
:
# MCP Server Implementation
from mcp import types as mcp_types
from mcp.server.lowlevel import Server
from mcp.server.models import InitializationOptions
import mcp.server.stdio
import json
import asyncio
from google.adk.tools.function_tool import FunctionTool
from google.adk.tools.mcp_tool.conversion_utils import adk_to_mcp_tool_type
def create_mcp_server():
"""Creates an MCP server exposing our agent's tools."""
from multi_tool_agent.agent import get_weather, get_current_time
# Wrap functions in FunctionTool objects
weather_tool = FunctionTool(get_weather)
time_tool = FunctionTool(get_current_time)
# Create MCP Server
app = Server("weather-time-mcp-server")
@app.list_tools()
async def list_tools() -> list[mcp_types.Tool]:
"""List available tools."""
# Convert ADK tools to MCP format
mcp_tools = [
adk_to_mcp_tool_type(weather_tool),
adk_to_mcp_tool_type(time_tool)
]
return mcp_tools
@app.call_tool()
async def call_tool(name: str, arguments: dict) -> list[mcp_types.TextContent]:
"""Execute a tool call."""
# Map tool names to functions
tools = {
weather_tool.name: weather_tool,
time_tool.name: time_tool
}
if name in tools:
try:
# Execute the tool
result = await tools[name].run_async(
args=arguments,
tool_context=None,
)
return [mcp_types.TextContent(type="text", text=json.dumps(result))]
except Exception as e:
return [mcp_types.TextContent(
type="text",
text=json.dumps({"error": str(e)})
)]
else:
return [mcp_types.TextContent(
type="text",
text=json.dumps({"error": f"Tool '{name}' not found"})
)]
return app
async def run_mcp_server():
"""Run the MCP server over standard I/O."""
app = create_mcp_server()
async with mcp.server.stdio.stdio_server() as (read_stream, write_stream):
await app.run(
read_stream,
write_stream,
InitializationOptions(
server_name=app.name,
server_version="0.1.0",
capabilities=app.get_capabilities(),
),
)
Add Command-Line Argument Support
Update the main section to support both modes:
if __name__ == "__main__":
# Parse command line arguments
import argparse
parser = argparse.ArgumentParser(description="ADK Agent Server")
parser.add_argument("--mode", choices=["web", "mcp"], default="web",
help="Run as web server or MCP server (default: web)")
args = parser.parse_args()
if args.mode == "mcp":
# Run as MCP server
print("Starting MCP server mode...")
asyncio.run(run_mcp_server())
else:
# Run as web server (default)
print("Starting Web server mode...")
uvicorn.run(
app,
host="0.0.0.0",
port=9999,
reload=False
)
Testing the MCP Server
Create a simple test file test_mcp_server.py
:
import os
import asyncio
import subprocess
import time
from google.adk.tools.mcp_tool import MCPToolset
from mcp.client.stdio import StdioServerParameters
API_SCRIPT_PATH = os.path.join(os.path.dirname(os.path.abspath(__file__)), "api.py")
async def test_mcp_server():
"""Test the MCP server by checking tool availability."""
# Connect to MCP server
tools, exit_stack = await MCPToolset.from_server(
connection_params=StdioServerParameters(
command='python',
args=[API_SCRIPT_PATH, '--mode', 'mcp']
)
)
try:
# List available tools
tool_names = [tool.name for tool in tools]
print(f"Found {len(tool_names)} tools: {', '.join(tool_names)}")
# Verify expected tools are available
if "get_weather" in tool_names and "get_current_time" in tool_names:
print("SUCCESS: MCP server is working correctly!")
else:
print("WARNING: Not all expected tools were found!")
finally:
await exit_stack.aclose()
if __name__ == "__main__":
asyncio.run(test_mcp_server())
Run the test:
python test_mcp_server.py
Using Your MCP Server with Claude or other AI Assistants
Once your MCP server is running, you can use it with any MCP-compatible AI assistant:
- Start your MCP server:
python api.py --mode mcp
Configure your AI assistant (e.g., Claude) to use this MCP server. For Claude Desktop, you'd point it to your running MCP server.
-
The AI assistant will be able to:
- Discover the available tools (get_weather and get_current_time)
- Call these tools when appropriate during conversations
Best Practices
-
Environment Variables: Store API keys and credentials in a
.env
file - Error Handling: Add proper error handling in both tool implementations
- Logging: Add comprehensive logging for debugging purposes
- Authentication: Add authentication for production deployments
- Testing: Write unit tests for your tools and integration tests for your endpoints
Common Issues and Solutions
-
Agent Not Found in FastAPI: Make sure
AGENT_DIR
is set to the parent directory containing your agent subdirectory - MCP Tool Conversion Errors: Ensure your tool functions have proper type hints
-
Module Import Errors: Check your
__init__.py
files export the required objects
Conclusion
You now have a flexible codebase that can:
- Run as a standalone ADK agent
- Serve as a FastAPI web application with a UI
- Function as an MCP server for AI assistants
This approach gives you multiple deployment options from the same codebase, making your AI agent more versatile and accessible.