AI In Fraud Detection: How Banks Use Machine Learning
Fraud has always been a big headache for banks. Every day, millions of people make online transactions, and fraudsters are always looking for ways to take advantage. But now, banks are fighting back using Artificial Intelligence (AI) and Machine Learning (ML). These technologies are changing the way banks detect and prevent fraud. Let’s explore how this works in simple terms, with real-life examples and useful insights. What is AI Fraud Detection? AI fraud detection means using computer systems that can “think” and “learn” like humans. These systems can identify suspicious activity by analyzing huge amounts of data. For example, if a person usually spends money in Pakistan but suddenly makes a big purchase in the US at midnight, the AI might flag that transaction as unusual. If you were facing the same issue, wouldn’t you expect your bank to take action quickly? Well, that’s exactly what AI is helping them do. These AI systems learn from past data. They spot patterns that don’t look normal. Over time, they get smarter and more accurate. In short, AI helps banks keep an eye on your money 24/7. How do AI and Machine learning detect and prevent fraud? AI and ML use data to predict whether a transaction is real or fake. To do this, they use algorithms and step-by-step rules that help the system make decisions. For instance, if someone buys fuel every morning, that looks normal. But if the same card is used to buy jewellery and then transfer money abroad in five minutes, something fishy is going on. AI systems can immediately block such activities or send alerts. Banks also use natural language processing (NLP), a branch of AI that understands human language. It helps them read customer complaints or social media posts that may hint at fraud. So yes, your tweet about a hacked card might help your bank take faster action! Moreover, these systems use facial recognition and biometric data. If someone tries to log in using your account but their face doesn’t match yours, access will be denied. Isn’t that a smart move? Traditional Fraud Detection vs. Machine Learning Fraud Detection In the past, banks depended mostly on rule-based systems. These systems had fixed conditions. For example, “If a person spends more than $10,000, then flag the transaction.” But fraudsters quickly learned how to bypass those rules. Traditional systems were slow, and many genuine transactions got blocked. On the other hand, AI and ML are faster and more flexible. They learn from every fraud case and update themselves. That’s why they’re more efficient. To paint a clearer picture: according to a report by Statista, global losses due to payment card fraud reached around $28.65 billion in 2020. But with the use of AI, banks have been able to reduce false positives by up to 50%, saving time and customer trust. Challenges and Solutions Of course, AI and ML are not perfect. They do face challenges. One of the biggest problems is data quality. If the data is wrong or outdated, the AI might make a wrong decision. Another issue is bias. If an AI system is trained on biased data, it may treat certain customers unfairly. For example, it may think a new customer is a fraudster just because their transaction pattern is different. But banks are working hard to fix these problems. They are training AI models on better and more diverse data. They also regularly test and improve the systems to ensure fairness. If they keep doing this, they will surely overcome these hurdles. Furthermore, privacy is a big concern. People may worry that banks are watching their every move. However, with proper policies and data protection laws, customer information can be kept safe. As the saying goes, “Where there’s a will, there’s a way.” How Can We Help? AI is powerful, but it still needs human help. If people report suspicious activity quickly, banks can take action sooner. Also, using strong passwords and enabling two-factor authentication makes it harder for fraudsters to succeed. If banks could educate customers regularly, there would be fewer fraud cases. For example, they might say: “If you ever receive a call asking for your PIN, hang up immediately and report it.” This kind of awareness is just as important as technology. Let’s not forget technology must go hand-in-hand with responsibility. If banks use AI responsibly, and if customers stay alert, fraud can be greatly reduced. Glaxit: Your Trusted Tech Partner If you’re a bank or financial institution looking to adopt AI-powered fraud detection systems, Glaxit can help. With years of experience in AI solutions and financial technology, Glaxit provides custom-made tools that help detect fraud before it happens. Whether you’re a small bank or a large enterprise, our team understands the unique challenges you face. We believe that if businesses use the right tools at the right time, they can avoid major losses. So, don’t wait for
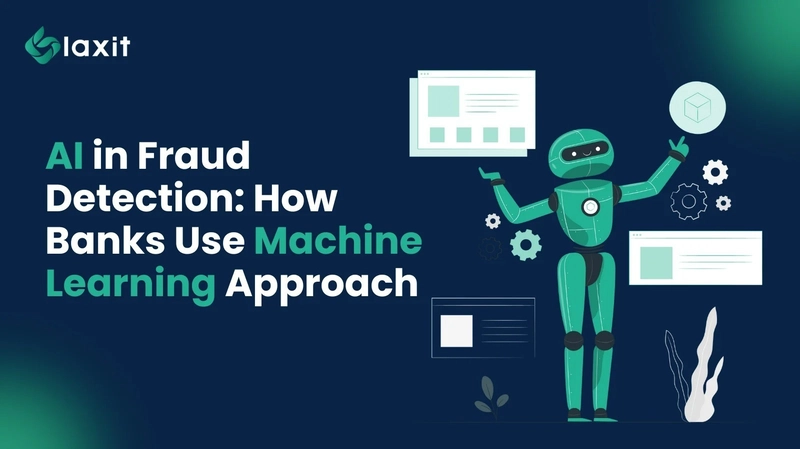
Fraud has always been a big headache for banks. Every day, millions of people make online transactions, and fraudsters are always looking for ways to take advantage. But now, banks are fighting back using Artificial Intelligence (AI) and Machine Learning (ML). These technologies are changing the way banks detect and prevent fraud. Let’s explore how this works in simple terms, with real-life examples and useful insights.
What is AI Fraud Detection?
AI fraud detection means using computer systems that can “think” and “learn” like humans. These systems can identify suspicious activity by analyzing huge amounts of data. For example, if a person usually spends money in Pakistan but suddenly makes a big purchase in the US at midnight, the AI might flag that transaction as unusual. If you were facing the same issue, wouldn’t you expect your bank to take action quickly? Well, that’s exactly what AI is helping them do.
These AI systems learn from past data. They spot patterns that don’t look normal. Over time, they get smarter and more accurate. In short, AI helps banks keep an eye on your money 24/7.
How do AI and Machine learning detect and prevent fraud?
AI and ML use data to predict whether a transaction is real or fake. To do this, they use algorithms and step-by-step rules that help the system make decisions. For instance, if someone buys fuel every morning, that looks normal. But if the same card is used to buy jewellery and then transfer money abroad in five minutes, something fishy is going on. AI systems can immediately block such activities or send alerts.
Banks also use natural language processing (NLP), a branch of AI that understands human language. It helps them read customer complaints or social media posts that may hint at fraud. So yes, your tweet about a hacked card might help your bank take faster action!
Moreover, these systems use facial recognition and biometric data. If someone tries to log in using your account but their face doesn’t match yours, access will be denied. Isn’t that a smart move?
Traditional Fraud Detection vs. Machine Learning Fraud Detection
In the past, banks depended mostly on rule-based systems. These systems had fixed conditions. For example, “If a person spends more than $10,000, then flag the transaction.” But fraudsters quickly learned how to bypass those rules.
Traditional systems were slow, and many genuine transactions got blocked. On the other hand, AI and ML are faster and more flexible. They learn from every fraud case and update themselves. That’s why they’re more efficient.
To paint a clearer picture: according to a report by Statista, global losses due to payment card fraud reached around $28.65 billion in 2020. But with the use of AI, banks have been able to reduce false positives by up to 50%, saving time and customer trust.
Challenges and Solutions
Of course, AI and ML are not perfect. They do face challenges. One of the biggest problems is data quality. If the data is wrong or outdated, the AI might make a wrong decision.
Another issue is bias. If an AI system is trained on biased data, it may treat certain customers unfairly. For example, it may think a new customer is a fraudster just because their transaction pattern is different.
But banks are working hard to fix these problems. They are training AI models on better and more diverse data. They also regularly test and improve the systems to ensure fairness. If they keep doing this, they will surely overcome these hurdles. Furthermore, privacy is a big concern. People may worry that banks are watching their every move. However, with proper policies and data protection laws, customer information can be kept safe. As the saying goes, “Where there’s a will, there’s a way.”
How Can We Help?
AI is powerful, but it still needs human help. If people report suspicious activity quickly, banks can take action sooner. Also, using strong passwords and enabling two-factor authentication makes it harder for fraudsters to succeed.
If banks could educate customers regularly, there would be fewer fraud cases. For example, they might say: “If you ever receive a call asking for your PIN, hang up immediately and report it.” This kind of awareness is just as important as technology. Let’s not forget technology must go hand-in-hand with responsibility. If banks use AI responsibly, and if customers stay alert, fraud can be greatly reduced.
Glaxit: Your Trusted Tech Partner
If you’re a bank or financial institution looking to adopt AI-powered fraud detection systems, Glaxit can help. With years of experience in AI solutions and financial technology, Glaxit provides custom-made tools that help detect fraud before it happens. Whether you’re a small bank or a large enterprise, our team understands the unique challenges you face.
We believe that if businesses use the right tools at the right time, they can avoid major losses. So, don’t wait for fraud to happen, act now! With Glaxit expertise, your systems will not only get smarter but also more secure. Let’s work together to create a safer digital world because prevention is always better than cure.