What role does latent space play in Generative AI?
Latent space plays a crucial role in Generative AI by representing data in a compressed, meaningful format that captures essential features and patterns. It is an abstract, high-dimensional space where similar data points are positioned close to each other, enabling models to generate new, realistic samples based on learned representations. In models like Generative Adversarial Networks (GANs) and Variational Autoencoders (VAEs), latent space acts as an intermediary between raw input data and the generated output. For example, in image generation, instead of directly manipulating pixels, models encode input images into a latent space representation, which helps in learning underlying structures like shapes, colors, and textures. When generating new images, the model samples points from the latent space and decodes them into realistic outputs. One key advantage of latent space is its ability to control and manipulate generated data. By adjusting latent variables, users can modify specific attributes of an image, such as changing facial expressions, styles, or object features. This capability is widely used in applications like deepfake generation, AI-powered design, and personalized content creation. Latent space also enhances efficiency by reducing the dimensionality of data, making training and inference faster. Since models learn a compressed representation of data, they can generalize better and generate high-quality outputs with minimal computational resources. Understanding latent space is essential for anyone working with Generative AI, as it provides insights into how AI models learn and create new content. To gain expertise in this field, professionals should focus on deep learning techniques, neural networks, and model optimization. Obtaining a Gen AI and machine learning certification can help individuals master these concepts and advance their careers in AI-driven industries.
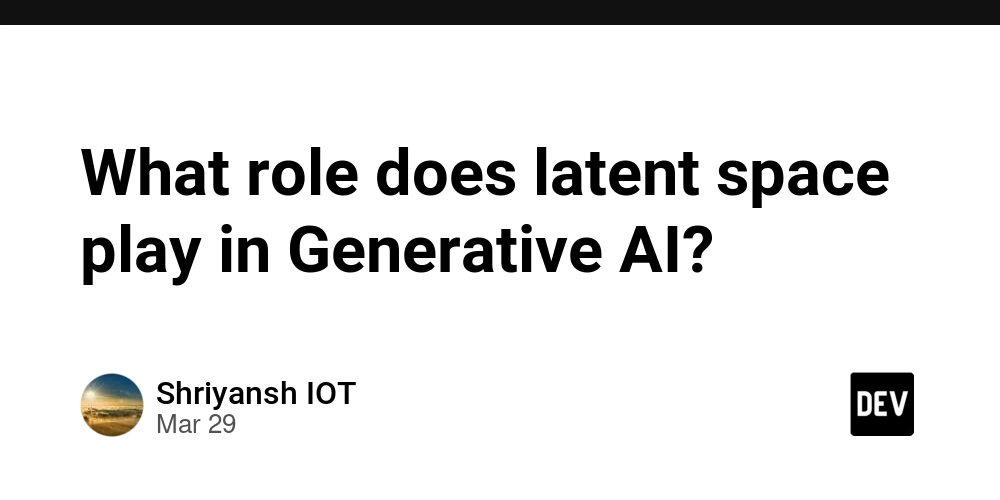
Latent space plays a crucial role in Generative AI by representing data in a compressed, meaningful format that captures essential features and patterns. It is an abstract, high-dimensional space where similar data points are positioned close to each other, enabling models to generate new, realistic samples based on learned representations.
In models like Generative Adversarial Networks (GANs) and Variational Autoencoders (VAEs), latent space acts as an intermediary between raw input data and the generated output. For example, in image generation, instead of directly manipulating pixels, models encode input images into a latent space representation, which helps in learning underlying structures like shapes, colors, and textures. When generating new images, the model samples points from the latent space and decodes them into realistic outputs.
One key advantage of latent space is its ability to control and manipulate generated data. By adjusting latent variables, users can modify specific attributes of an image, such as changing facial expressions, styles, or object features. This capability is widely used in applications like deepfake generation, AI-powered design, and personalized content creation.
Latent space also enhances efficiency by reducing the dimensionality of data, making training and inference faster. Since models learn a compressed representation of data, they can generalize better and generate high-quality outputs with minimal computational resources.
Understanding latent space is essential for anyone working with Generative AI, as it provides insights into how AI models learn and create new content. To gain expertise in this field, professionals should focus on deep learning techniques, neural networks, and model optimization. Obtaining a Gen AI and machine learning certification can help individuals master these concepts and advance their careers in AI-driven industries.