Random Forest Algorithm: Powering Smarter Predictions with Supervised Learning
Definition Explains Random Forest as an ensemble method that combines decision trees to improve performance and reduce overfitting. Working Steps Outlines four key steps — Bootstrapping, Tree Building, Random Feature Selection, and Voting/Averaging. Advantages Lists benefits like reducing overfitting, handling missing data, working well with large datasets, and providing feature importance. Applications Mentions real-world uses like fraud detection, medical diagnosis, stock market prediction, and more. Key Parameters Specifies important settings like n_estimators, max_depth, max_features, and bootstrap.
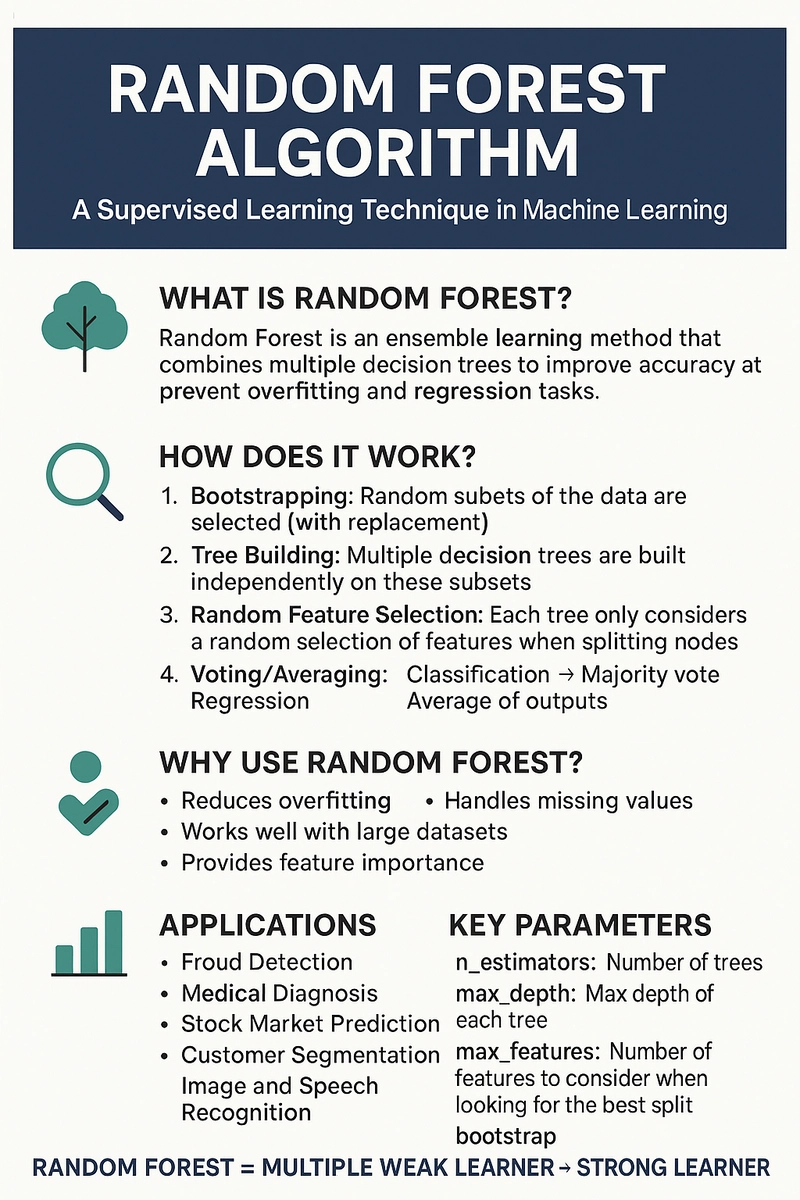
Definition
Explains Random Forest as an ensemble method that combines decision trees to improve performance and reduce overfitting.
Working Steps
Outlines four key steps — Bootstrapping, Tree Building, Random Feature Selection, and Voting/Averaging.
Advantages
Lists benefits like reducing overfitting, handling missing data, working well with large datasets, and providing feature importance.
Applications
Mentions real-world uses like fraud detection, medical diagnosis, stock market prediction, and more.
Key Parameters
Specifies important settings like n_estimators, max_depth, max_features, and bootstrap.