Lessons from Small Data in the Era of Big Data
Data is often called the fuel of the 21st century—and it’s not hard to see why. Over the past few decades, we’ve witnessed an incredible transformation in how we collect, analyze, and leverage data. What began with small, curated datasets has now exploded into an era of big data, reshaping industries and even influencing major global events. In this post, I’ll share some lessons learned from working with small data and explore how these insights can help us tackle the challenges posed by big data. From Small Data to Big Data: A Brief Overview The Era of Small Data Small data refers to datasets that are typically smaller in volume, more structured, and often carefully curated. Think of it like a well-organized spreadsheet where every piece of information is handpicked and cleaned before analysis. This approach worked well for a long time and provided many useful insights in fields like education, healthcare, and finance. Some key takeaways from the small data era include: Data Quality is Crucial: No matter how sophisticated your analysis or machine learning model is, “garbage in is garbage out.” Ensuring that data is clean, consistent, and complete is a non-negotiable first step. Simplicity Wins: Often, a simple statistical model can offer powerful insights. With small datasets, complex models aren’t always necessary to derive actionable insights. Ethical Considerations: Even with small datasets, issues like privacy, informed consent, and data security were always on the radar—and they’re even more important now. Enter Big Data Big data, as the name suggests, involves massive and complex datasets that can capture the full spectrum of information—from online transactions and sensor data to social media interactions and beyond. Big data is characterized by the famous three V’s: Volume: The sheer amount of data generated every second is staggering. Variety: Data now comes in many forms—structured, unstructured, and semi-structured. Velocity: Data is generated and needs to be processed at high speeds. This transition has enabled organizations to uncover hidden patterns and make real-time decisions. However, with great power comes great challenges. The Big Data Challenge: Lessons Learned and Road Ahead While big data offers immense opportunities, it also introduces new complexities that need careful consideration: Data Quality and Noise Even with huge datasets, the problem of quality persists. The larger the dataset, the more challenging it becomes to filter out noise and ensure consistency. The lessons from small data remind us that robust data cleaning and preprocessing remain vital. Scalability and Storage Traditional databases simply can’t keep up with the petabytes of data generated today. Solutions like Hadoop, Spark, and cloud storage platforms (Amazon S3, Google Cloud, etc.) have emerged to address these challenges. Yet, these technologies bring their own set of complexities and costs. Security and Privacy Securing data at scale is no small feat. As data flows faster and in greater volumes, protecting sensitive information becomes increasingly challenging. Implementing multi-layered security protocols and robust privacy measures is essential to avoid breaches and maintain trust. Real-Time Insights In today’s fast-paced environment, the value of data decreases with time. Systems must not only store and process big data but also transform it into real-time, actionable insights. This requires specialized algorithms and data pipelines that can handle high-speed data streams. Cost Implications The infrastructure needed to store, process, and analyze big data is not only complex but also expensive. Organizations need to balance the potential benefits against the significant investments in technology and skilled personnel. Reflecting on the Past, Innovating for the Future One central question emerges: "If we didn’t fully solve the challenges of small data, how can we expect to master big data?" The answer lies in building on the lessons of the past. We must continue to refine data quality practices, focus on ethical considerations, and develop scalable technologies that don’t sacrifice real-time performance for volume. While big data is still evolving, the path forward is clear. Collaboration between industry, academia, and government is crucial to develop innovative solutions that harness the full potential of big data while mitigating its challenges. Conclusion Big data is not just a technological buzzword—it’s a transformative force that is reshaping industries and our daily lives. By learning from the limitations and successes of small data, we can design better systems that not only handle large volumes of data but also provide timely, accurate, and ethical insights. As we navigate this exciting era, I invite you to share your thoughts and experiences. How have you tackled the challenges of big data in your projects? Let’s learn from eac
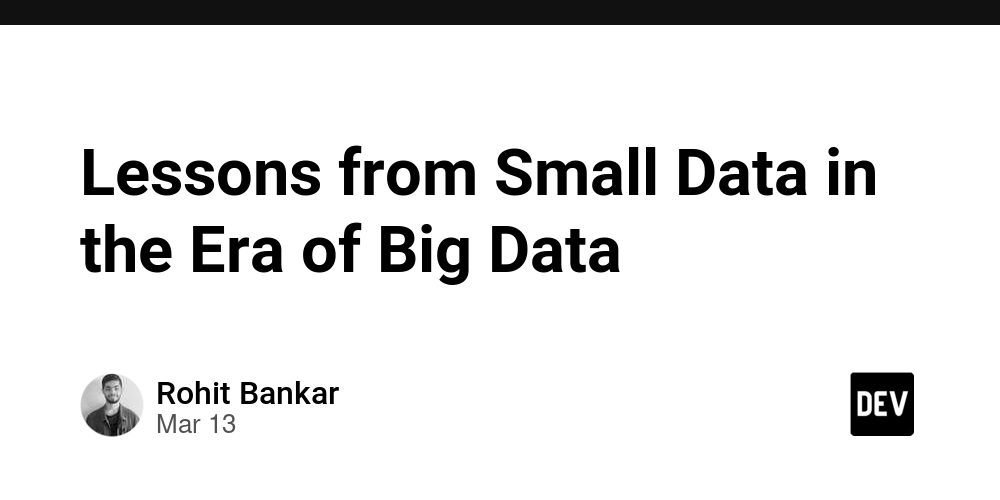
Data is often called the fuel of the 21st century—and it’s not hard to see why. Over the past few decades, we’ve witnessed an incredible transformation in how we collect, analyze, and leverage data. What began with small, curated datasets has now exploded into an era of big data, reshaping industries and even influencing major global events.
In this post, I’ll share some lessons learned from working with small data and explore how these insights can help us tackle the challenges posed by big data.
From Small Data to Big Data: A Brief Overview
The Era of Small Data
Small data refers to datasets that are typically smaller in volume, more structured, and often carefully curated. Think of it like a well-organized spreadsheet where every piece of information is handpicked and cleaned before analysis. This approach worked well for a long time and provided many useful insights in fields like education, healthcare, and finance.
Some key takeaways from the small data era include:
Data Quality is Crucial: No matter how sophisticated your analysis or machine learning model is, “garbage in is garbage out.” Ensuring that data is clean, consistent, and complete is a non-negotiable first step.
Simplicity Wins: Often, a simple statistical model can offer powerful insights. With small datasets, complex models aren’t always necessary to derive actionable insights.
Ethical Considerations: Even with small datasets, issues like privacy, informed consent, and data security were always on the radar—and they’re even more important now.
Enter Big Data
Big data, as the name suggests, involves massive and complex datasets that can capture the full spectrum of information—from online transactions and sensor data to social media interactions and beyond. Big data is characterized by the famous three V’s:
Volume: The sheer amount of data generated every second is staggering.
Variety: Data now comes in many forms—structured, unstructured, and semi-structured.
Velocity: Data is generated and needs to be processed at high speeds.
This transition has enabled organizations to uncover hidden patterns and make real-time decisions. However, with great power comes great challenges.
The Big Data Challenge: Lessons Learned and Road Ahead
While big data offers immense opportunities, it also introduces new complexities that need careful consideration:
Data Quality and Noise
Even with huge datasets, the problem of quality persists. The larger the dataset, the more challenging it becomes to filter out noise and ensure consistency. The lessons from small data remind us that robust data cleaning and preprocessing remain vital.Scalability and Storage
Traditional databases simply can’t keep up with the petabytes of data generated today. Solutions like Hadoop, Spark, and cloud storage platforms (Amazon S3, Google Cloud, etc.) have emerged to address these challenges. Yet, these technologies bring their own set of complexities and costs.Security and Privacy
Securing data at scale is no small feat. As data flows faster and in greater volumes, protecting sensitive information becomes increasingly challenging. Implementing multi-layered security protocols and robust privacy measures is essential to avoid breaches and maintain trust.Real-Time Insights
In today’s fast-paced environment, the value of data decreases with time. Systems must not only store and process big data but also transform it into real-time, actionable insights. This requires specialized algorithms and data pipelines that can handle high-speed data streams.Cost Implications
The infrastructure needed to store, process, and analyze big data is not only complex but also expensive. Organizations need to balance the potential benefits against the significant investments in technology and skilled personnel.
Reflecting on the Past, Innovating for the Future
One central question emerges: "If we didn’t fully solve the challenges of small data, how can we expect to master big data?"
The answer lies in building on the lessons of the past. We must continue to refine data quality practices, focus on ethical considerations, and develop scalable technologies that don’t sacrifice real-time performance for volume.
While big data is still evolving, the path forward is clear. Collaboration between industry, academia, and government is crucial to develop innovative solutions that harness the full potential of big data while mitigating its challenges.
Conclusion
Big data is not just a technological buzzword—it’s a transformative force that is reshaping industries and our daily lives. By learning from the limitations and successes of small data, we can design better systems that not only handle large volumes of data but also provide timely, accurate, and ethical insights.
As we navigate this exciting era, I invite you to share your thoughts and experiences. How have you tackled the challenges of big data in your projects? Let’s learn from each other and continue to push the boundaries of what data can achieve.