last developments for LLM x robotics
LLM-DaaS: Revolutionizing Drone Operations with Language Models In an era where drones are becoming integral to various industries, the need for streamlined operations is more critical than ever. Enter LLM-DaaS, a groundbreaking framework that harnesses the capabilities of Large Language Models (LLMs) to elevate Drone-as-a-Service (DaaS) operations. This innovative approach translates natural language user requests into structured, actionable tasks, addressing a significant challenge within the realm of drone services. Transforming Text into Actionable Tasks One of the main hurdles in drone service operations is accurately interpreting and structuring user requests made in everyday language. LLM-DaaS tackles this issue head-on. By fine-tuning models like Phi-3.5, LLaMA-3.2, and Gemma 2b on a specifically curated dataset that maps free-text user requests to structured DaaS commands, the system enables users to engage in a more human-like conversational exchange when discussing their drone service needs, particularly in scenarios like package delivery. Users can articulate their requests freely, and the finely-tuned LLM efficiently extracts essential DaaS metadata, such as delivery timelines, the source and destination locations, and the weight of the packages. This level of sophistication allows for a seamless transition from a simple user inquiry to a comprehensive operational plan. Smart Selection and Composition of Drone Services LLM-DaaS does not stop at translating requests; it also features a robust service selection model. This model identifies the best available drone for the job, ensuring the chosen vehicle is capable of transporting the package efficiently from the initial delivery point to the nearest optimal destination. When necessary, the DaaS composition model can craft a composite service utilizing multiple drones to transport packages directly from the source to the final destination. This dynamic capability is especially crucial in emergency response scenarios or in highly populated urban areas where logistical challenges are prevalent. Integrating Real-Time Data for Enhanced Operations Incorporating real-time weather data is a game-changer. By optimizing route planning and scheduling according to the latest environmental conditions, LLM-DaaS not only boosts operational efficiency but also enhances the safety of drone operations under uncertain circumstances. Simulations conducted to test LLM-DaaS indicate a marked improvement in task accuracy and overall operational efficacy. These results suggest that the framework is not only viable but is set to establish itself as a crucial component of DaaS operations—especially in environments fraught with unpredictability. Conclusion As drone services continue to expand and evolve, frameworks like LLM-DaaS will play invaluable roles in harnessing the full potential of these technologies. By transforming simple, free-text user requests into complex, structured tasks, and ensuring optimal drone selection and deployment, LLM-DaaS exemplifies the intersection of artificial intelligence and operational logistics. For those interested in delving deeper into this innovative framework, full details can be found in the original paper, available here. As we navigate through the future of drone operations, LLM-DaaS stands out as a clear beacon of technological advancement in automating services with efficiency and reliability.
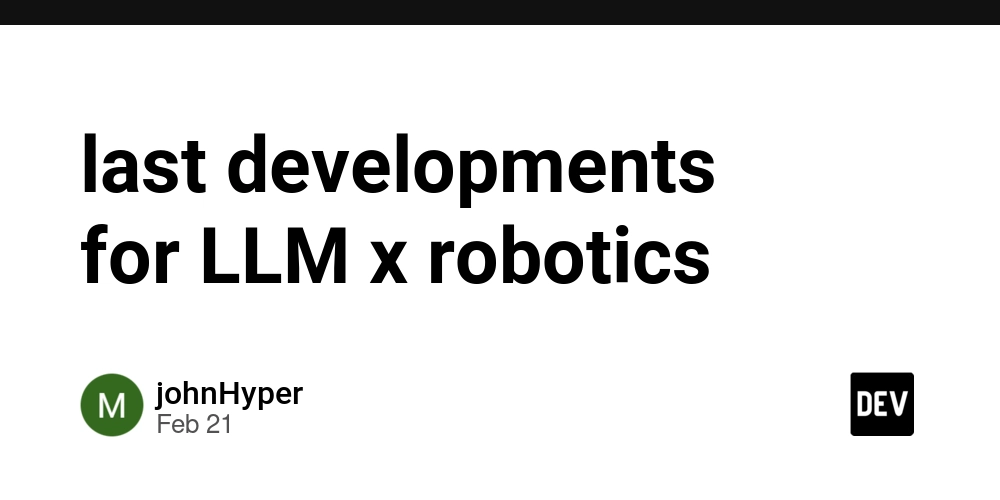
LLM-DaaS: Revolutionizing Drone Operations with Language Models
In an era where drones are becoming integral to various industries, the need for streamlined operations is more critical than ever. Enter LLM-DaaS, a groundbreaking framework that harnesses the capabilities of Large Language Models (LLMs) to elevate Drone-as-a-Service (DaaS) operations. This innovative approach translates natural language user requests into structured, actionable tasks, addressing a significant challenge within the realm of drone services.
Transforming Text into Actionable Tasks
One of the main hurdles in drone service operations is accurately interpreting and structuring user requests made in everyday language. LLM-DaaS tackles this issue head-on. By fine-tuning models like Phi-3.5, LLaMA-3.2, and Gemma 2b on a specifically curated dataset that maps free-text user requests to structured DaaS commands, the system enables users to engage in a more human-like conversational exchange when discussing their drone service needs, particularly in scenarios like package delivery.
Users can articulate their requests freely, and the finely-tuned LLM efficiently extracts essential DaaS metadata, such as delivery timelines, the source and destination locations, and the weight of the packages. This level of sophistication allows for a seamless transition from a simple user inquiry to a comprehensive operational plan.
Smart Selection and Composition of Drone Services
LLM-DaaS does not stop at translating requests; it also features a robust service selection model. This model identifies the best available drone for the job, ensuring the chosen vehicle is capable of transporting the package efficiently from the initial delivery point to the nearest optimal destination. When necessary, the DaaS composition model can craft a composite service utilizing multiple drones to transport packages directly from the source to the final destination.
This dynamic capability is especially crucial in emergency response scenarios or in highly populated urban areas where logistical challenges are prevalent.
Integrating Real-Time Data for Enhanced Operations
Incorporating real-time weather data is a game-changer. By optimizing route planning and scheduling according to the latest environmental conditions, LLM-DaaS not only boosts operational efficiency but also enhances the safety of drone operations under uncertain circumstances.
Simulations conducted to test LLM-DaaS indicate a marked improvement in task accuracy and overall operational efficacy. These results suggest that the framework is not only viable but is set to establish itself as a crucial component of DaaS operations—especially in environments fraught with unpredictability.
Conclusion
As drone services continue to expand and evolve, frameworks like LLM-DaaS will play invaluable roles in harnessing the full potential of these technologies. By transforming simple, free-text user requests into complex, structured tasks, and ensuring optimal drone selection and deployment, LLM-DaaS exemplifies the intersection of artificial intelligence and operational logistics.
For those interested in delving deeper into this innovative framework, full details can be found in the original paper, available here.
As we navigate through the future of drone operations, LLM-DaaS stands out as a clear beacon of technological advancement in automating services with efficiency and reliability.