Google Unveils Ironwood: 7th Gen TPU for Enhanced AI Inference
Originally published at ssojet Google has unveiled Ironwood, its seventh-generation Tensor Processing Unit (TPU), engineered specifically for inference workloads. Ironwood represents a significant leap in AI computational capabilities, designed to tackle the demands of generative AI models. Image courtesy of TechRadar Performance and Efficiency Ironwood can scale up to 9,216 chips, providing an impressive 42.5 Exaflops of compute power. This performance exceeds that of the world's largest supercomputer, El Capitan, which offers 1.7 Exaflops per pod. Each chip delivers peak performance of 4,614 TFLOPs. Ironwood is built to support advanced AI models including Large Language Models (LLMs) and Mixture of Experts (MoEs). The design minimizes data movement and latency, essential for handling massive tensor manipulations effectively. Key performance features include: High Bandwidth Memory (HBM): Each chip is equipped with 192 GB of HBM, which is 6 times that of the previous Trillium generation, allowing for larger model processing and reducing data transfer needs. Improved Bandwidth: Ironwood achieves a bandwidth of 7.2 TBps per chip, 4.5 times greater than Trillium. Inter-Chip Interconnect (ICI): Bandwidth is enhanced to 1.2 Tbps bidirectional, facilitating efficient communication between chips. For more details, refer to the Google Cloud Blog and TechRadar. AI Hypercomputer Integration Ironwood is part of Google's AI Hypercomputer architecture, a system optimized for AI workloads. This integrated supercomputing system leverages over a decade of AI expertise. It supports various frameworks such as Vertex AI and Pathways, enabling developers to utilize Ironwood effectively for distributed computing. Image courtesy of Google Cloud Blog Software and Training Features Google's Pathways framework enhances Ironwood's capabilities for AI training and inference. Pathways on Cloud provides features like disaggregated serving, allowing for dynamic scaling of inference workloads. Cluster Director for GKE and Slurm enables efficient management of clusters, ensuring high availability and quick provisioning. The system supports advanced features such as: 360° Observability: Monitoring cluster performance and health. Job Continuity: Automated health checks and node replacement to maintain workload continuity. For more information on training capabilities, see Google Cloud's documentation. Hardware Specifications and Configuration Ironwood TPUs are available in two configurations: a smaller 256-chip pod and a larger 9,216-chip pod. The larger pod is particularly capable for demanding AI training workloads. Each Ironwood chip supports FP8 calculations, marking a shift from previous INT8 and BF16 formats. This enhances training throughput significantly, allowing for optimized performance across various AI applications. Key hardware specifications include: Power Efficiency: Ironwood is designed to be 2x more power efficient compared to Trillium. Chip Design: Incorporates multiple chiplets and HBM3E memory to enhance processing power and flexibility. For further details on hardware specifications, visit TechRadar and The Next Platform. Conclusion Ironwood TPU is set to redefine the capabilities of AI workloads by providing unprecedented power and efficiency. Its integration within Google's AI Hypercomputer system and advanced software frameworks position it as a leading solution for enterprise AI applications. For enterprises looking to implement secure Single Sign-On (SSO) and user management, consider SSOJet’s API-first platform. SSOJet offers directory sync, SAML, OIDC, and magic link authentication to enhance your security infrastructure. Explore our services or contact us at SSOJet.
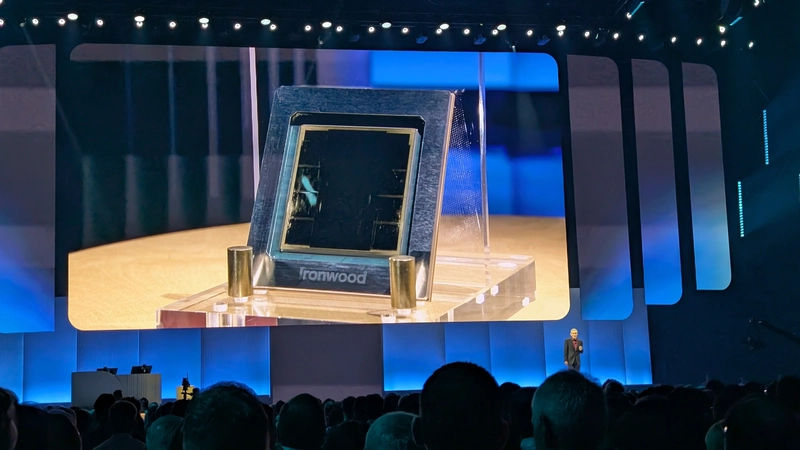
Originally published at ssojet
Google has unveiled Ironwood, its seventh-generation Tensor Processing Unit (TPU), engineered specifically for inference workloads. Ironwood represents a significant leap in AI computational capabilities, designed to tackle the demands of generative AI models.
Performance and Efficiency
Ironwood can scale up to 9,216 chips, providing an impressive 42.5 Exaflops of compute power. This performance exceeds that of the world's largest supercomputer, El Capitan, which offers 1.7 Exaflops per pod. Each chip delivers peak performance of 4,614 TFLOPs.
Ironwood is built to support advanced AI models including Large Language Models (LLMs) and Mixture of Experts (MoEs). The design minimizes data movement and latency, essential for handling massive tensor manipulations effectively.
Key performance features include:
- High Bandwidth Memory (HBM): Each chip is equipped with 192 GB of HBM, which is 6 times that of the previous Trillium generation, allowing for larger model processing and reducing data transfer needs.
- Improved Bandwidth: Ironwood achieves a bandwidth of 7.2 TBps per chip, 4.5 times greater than Trillium.
- Inter-Chip Interconnect (ICI): Bandwidth is enhanced to 1.2 Tbps bidirectional, facilitating efficient communication between chips.
For more details, refer to the Google Cloud Blog and TechRadar.
AI Hypercomputer Integration
Ironwood is part of Google's AI Hypercomputer architecture, a system optimized for AI workloads. This integrated supercomputing system leverages over a decade of AI expertise. It supports various frameworks such as Vertex AI and Pathways, enabling developers to utilize Ironwood effectively for distributed computing.
Image courtesy of Google Cloud Blog
Software and Training Features
Google's Pathways framework enhances Ironwood's capabilities for AI training and inference. Pathways on Cloud provides features like disaggregated serving, allowing for dynamic scaling of inference workloads.
Cluster Director for GKE and Slurm enables efficient management of clusters, ensuring high availability and quick provisioning. The system supports advanced features such as:
- 360° Observability: Monitoring cluster performance and health.
- Job Continuity: Automated health checks and node replacement to maintain workload continuity.
For more information on training capabilities, see Google Cloud's documentation.
Hardware Specifications and Configuration
Ironwood TPUs are available in two configurations: a smaller 256-chip pod and a larger 9,216-chip pod. The larger pod is particularly capable for demanding AI training workloads.
Each Ironwood chip supports FP8 calculations, marking a shift from previous INT8 and BF16 formats. This enhances training throughput significantly, allowing for optimized performance across various AI applications.
Key hardware specifications include:
- Power Efficiency: Ironwood is designed to be 2x more power efficient compared to Trillium.
- Chip Design: Incorporates multiple chiplets and HBM3E memory to enhance processing power and flexibility.
For further details on hardware specifications, visit TechRadar and The Next Platform.
Conclusion
Ironwood TPU is set to redefine the capabilities of AI workloads by providing unprecedented power and efficiency. Its integration within Google's AI Hypercomputer system and advanced software frameworks position it as a leading solution for enterprise AI applications.
For enterprises looking to implement secure Single Sign-On (SSO) and user management, consider SSOJet’s API-first platform. SSOJet offers directory sync, SAML, OIDC, and magic link authentication to enhance your security infrastructure. Explore our services or contact us at SSOJet.