AI Training Data News Q1 2025: Navigating the Dynamic Landscape of AI Training Datasets
Abstract: In Q1 2025, the global AI training data ecosystem is in a state of vibrant evolution. This post explores whether the AI boom is stalling or surging by examining key trends, innovative projects, emerging applications, regulatory influences, and future outlooks. Drawing on recent developments—from Google’s fair use initiatives and Nvidia’s robust performance to groundbreaking projects such as DeepSeek’s efficient R1 model—the post provides an in‐depth yet accessible analysis. We also delve into advances in synthetic data, blockchain tokenization, and ethical considerations that are reshaping the AI training landscape. With practical examples, structured tables, and bullet lists, this post is designed for developers, tech enthusiasts, and industry professionals looking to stay ahead in the rapidly evolving AI ecosystem. Introduction The rapid evolution of artificial intelligence continues to be fueled by innovations in AI training data. The discussion in AI circles over whether the boom is stalling or surging has created a critical dialogue. Recent news from Q1 2025—featured in the Original Article—highlights a period where challenges such as rising training costs, data shortages, and regulatory pressures intersect with groundbreaking innovations in synthetic data generation and blockchain tokenization. In this blog post, we unpack these discussions, provide relevant background information, and examine how these trends are shaping the future of AI training data. Background and Context The Rise of AI Training Data AI models rely on vast amounts of data. Early breakthroughs in neural networks were powered by large-scale datasets sourced from text, images, and other digital media. Today, however, the landscape has become more complex. Despite massive global data creation, much of this data is unstructured or proprietary, which increases costs and legal hurdles. Notable examples such as GPT-4 and Gemini Ultra have been known to have training costs reaching tens of millions of dollars, highlighting the financial and resource-intensive nature of these projects. For further context on training cost trends, check out this detailed analysis on Visual Capitalist. Regulatory and Ethical Considerations With the AI revolution comes a parallel evolution in regulatory oversight. The recently enforced EU AI Act has set stringent guidelines for data use, steering the industry towards fair and ethical practices. OpenAI’s lobbying for fewer U.S. restrictions illustrates the current regulatory tug-of-war that impacts innovation pace and data strategy in the AI domain. The Emergence of Synthetic Data and Blockchain Tokenization As real-world data becomes scarcer and more expensive, synthetic data is rising as a viable, cost-effective alternative. Institutions like MIT are leading the charge on synthetic data for robotics and other AI applications, as highlighted by MIT News. In parallel, blockchain initiatives are being harnessed to tokenized AI training datasets, ensuring data integrity and monetization. Projects exploring tokenization, such as those featured on License-token.com, underscore a move towards decentralized, secure data markets. Core Concepts and Features 1. Multimodal Data Integration Key Concept: AI systems today benefit from multimodal inputs, where text, images, audio, and video converge to enhance decision-making and pattern recognition. Features Include: Improved model robustness by combining diverse data types. Enhanced applications, particularly in robotics and conversational AI. Better contextual understanding through comprehensive data integration. 2. Synthetic Data as a Catalyst Core Concept: Synthetic data provides a workaround for issues of data scarcity and regulatory constraints by generating artificially created, yet realistic, datasets. Important Aspects: Lower training costs and reduced reliance on limited human-generated data. The use of advanced techniques such as Generative Adversarial Networks (GANs) and Variational Autoencoders (VAEs) that ensure high-quality synthetic output. Applications in industries where data privacy and alternative training strategies are essential. 3. Blockchain and Tokenization in Data Monetization Defining the Concept: Blockchain technology is increasingly used to tokenize datasets, making data transactions traceable and secure. Key Features: Security: Smart contracts ensure data usage is transparent and tamper-proof. Monetization: Tokenization transforms data assets into tradeable digital commodities. Interoperability: Emerging blockchain platforms are integrating with AI training ecosystems to facilitate seamless data access. 4. Ethical Data Practices and Open-Source Collaboration Challenge and Opportunity: Ethical data is central to sustaining AI’s societal acceptance. Notable Points: Tackling algorithmic bias and ens
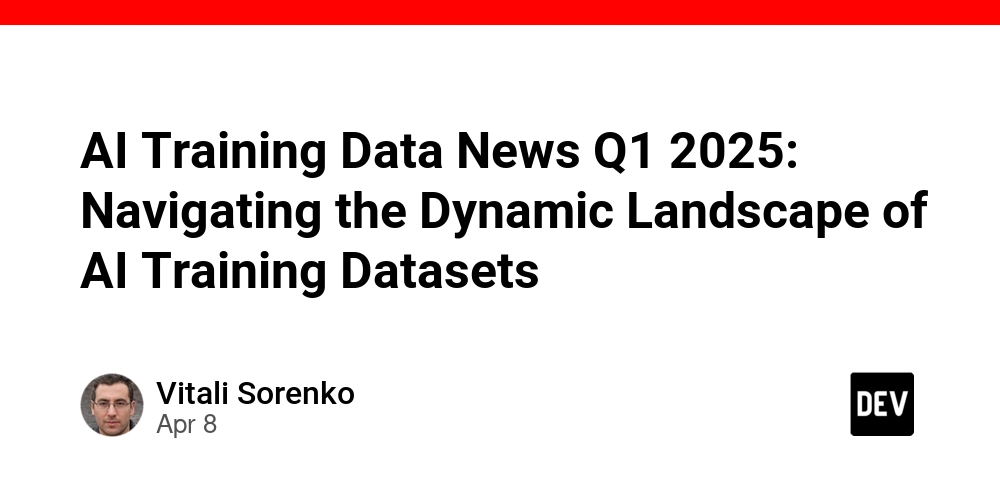
Abstract:
In Q1 2025, the global AI training data ecosystem is in a state of vibrant evolution. This post explores whether the AI boom is stalling or surging by examining key trends, innovative projects, emerging applications, regulatory influences, and future outlooks. Drawing on recent developments—from Google’s fair use initiatives and Nvidia’s robust performance to groundbreaking projects such as DeepSeek’s efficient R1 model—the post provides an in‐depth yet accessible analysis. We also delve into advances in synthetic data, blockchain tokenization, and ethical considerations that are reshaping the AI training landscape. With practical examples, structured tables, and bullet lists, this post is designed for developers, tech enthusiasts, and industry professionals looking to stay ahead in the rapidly evolving AI ecosystem.
Introduction
The rapid evolution of artificial intelligence continues to be fueled by innovations in AI training data. The discussion in AI circles over whether the boom is stalling or surging has created a critical dialogue. Recent news from Q1 2025—featured in the Original Article—highlights a period where challenges such as rising training costs, data shortages, and regulatory pressures intersect with groundbreaking innovations in synthetic data generation and blockchain tokenization. In this blog post, we unpack these discussions, provide relevant background information, and examine how these trends are shaping the future of AI training data.
Background and Context
The Rise of AI Training Data
AI models rely on vast amounts of data. Early breakthroughs in neural networks were powered by large-scale datasets sourced from text, images, and other digital media. Today, however, the landscape has become more complex. Despite massive global data creation, much of this data is unstructured or proprietary, which increases costs and legal hurdles. Notable examples such as GPT-4 and Gemini Ultra have been known to have training costs reaching tens of millions of dollars, highlighting the financial and resource-intensive nature of these projects. For further context on training cost trends, check out this detailed analysis on Visual Capitalist.
Regulatory and Ethical Considerations
With the AI revolution comes a parallel evolution in regulatory oversight. The recently enforced EU AI Act has set stringent guidelines for data use, steering the industry towards fair and ethical practices. OpenAI’s lobbying for fewer U.S. restrictions illustrates the current regulatory tug-of-war that impacts innovation pace and data strategy in the AI domain.
The Emergence of Synthetic Data and Blockchain Tokenization
As real-world data becomes scarcer and more expensive, synthetic data is rising as a viable, cost-effective alternative. Institutions like MIT are leading the charge on synthetic data for robotics and other AI applications, as highlighted by MIT News. In parallel, blockchain initiatives are being harnessed to tokenized AI training datasets, ensuring data integrity and monetization. Projects exploring tokenization, such as those featured on License-token.com, underscore a move towards decentralized, secure data markets.
Core Concepts and Features
1. Multimodal Data Integration
Key Concept: AI systems today benefit from multimodal inputs, where text, images, audio, and video converge to enhance decision-making and pattern recognition.
Features Include:
- Improved model robustness by combining diverse data types.
- Enhanced applications, particularly in robotics and conversational AI.
- Better contextual understanding through comprehensive data integration.
2. Synthetic Data as a Catalyst
Core Concept: Synthetic data provides a workaround for issues of data scarcity and regulatory constraints by generating artificially created, yet realistic, datasets.
Important Aspects:
- Lower training costs and reduced reliance on limited human-generated data.
- The use of advanced techniques such as Generative Adversarial Networks (GANs) and Variational Autoencoders (VAEs) that ensure high-quality synthetic output.
- Applications in industries where data privacy and alternative training strategies are essential.
3. Blockchain and Tokenization in Data Monetization
Defining the Concept: Blockchain technology is increasingly used to tokenize datasets, making data transactions traceable and secure.
Key Features:
- Security: Smart contracts ensure data usage is transparent and tamper-proof.
- Monetization: Tokenization transforms data assets into tradeable digital commodities.
- Interoperability: Emerging blockchain platforms are integrating with AI training ecosystems to facilitate seamless data access.
4. Ethical Data Practices and Open-Source Collaboration
Challenge and Opportunity: Ethical data is central to sustaining AI’s societal acceptance.
Notable Points:
- Tackling algorithmic bias and ensuring equitable data representation.
- Prioritizing privacy and GDPR compliance.
- Encouraging open-source data releases and community-funded initiatives, as exemplified by platforms like dev.to/vitalisorenko/blockchain-for-open-source-funding-a-new-paradigm-1moe.
Applications and Use Cases
Real-World Examples of AI Training Data in Action
Robotics and Adaptive Systems:
Google’s Gemini Robotics project leverages multimodal data to power adaptive robotic systems. By integrating sensor, visual, and audio data, these robots achieve higher levels of performance in real-world tasks, from warehouse logistics to autonomous navigation.Cost-Effective AI Models:
DeepSeek’s R1 model, launched in January 2025, demonstrates how budget-oriented approaches can produce competitive AI models. This project uses synthetic data to significantly reduce training costs, enabling smaller organizations to innovate without enormous financial barriers.Tokenized Data Marketplaces:
Platforms exploring blockchain tokenization—such as the initiatives detailed on License-token.com—are transforming the way data is bought, sold, and shared. This trend not only supports data integrity but also creates an ecosystem for sustainable data monetization, ensuring that every transaction is authenticated and secure.
Challenges and Limitations
While breakthroughs in AI training data bring exciting possibilities, several challenges still persist:
High Energy Consumption:
Training large-scale models requires extensive data center infrastructure, driving up energy usage. This is becoming a key bottleneck as models grow in size and complexity.Regulatory Ambiguity:
Despite recent strides, regulatory environments vary significantly across regions. The EU AI Act provides a stringent framework, but similar regulations in the U.S. and other regions remain fluid, leading to uncertainty.Data Quality and Bias:
Even synthetic data may introduce biases if not carefully managed. Ensuring the integrity and fairness of both real and synthetic datasets is a continuing challenge.Adoption Barriers:
While tokenization promises to democratize data access, the integration of blockchain technology into AI workflows is in its nascent stages. Issues related to scalability, interoperability, and market acceptance need to be resolved.Cost Concerns:
Although approaches like synthetic data reduce direct expenses, the initial investment in state-of-the-art infrastructure and the ongoing cost of regulatory compliance still pose significant challenges.
Future Outlook and Innovations
Looking ahead to Q2 2025 and beyond, several trends and innovations are likely to shape the AI training data landscape:
Predicted Trends
Increased Synthetic Data Adoption:
As real data becomes more regulated and expensive, synthetic data will see broader adoption. Innovations in GANs and VAEs are expected to further improve the fidelity of generated data, making it an even more viable alternative.Expansion of Blockchain-Based Data Marketplaces:
Tokenization will continue to mature, with blockchain solutions ensuring data traceability and monetization. As ethical concerns and transparency become more prominent, the merging of blockchain with AI training will likely set a new industry standard.Refined Regulatory Policies:
Global regulatory bodies are anticipated to refine data use guidelines. Continued discussions and harmonization efforts will address the current ambiguities, providing clearer pathways for data usage in AI training.Enhanced Energy Efficiency:
With the spotlight on environmental impact, tech giants like Nvidia are innovating to improve the energy efficiency of data centers. Advancements in low-power computing and sustainable infrastructure will drive the future of AI training.
Emerging Innovations
Domain-Specific Datasets:
Customized data collections tailored to niche applications will enhance model performance for specialized tasks, such as medical diagnostics or legal analytics.Real-Time Data Integration:
Projects like xAI’s Grok offer continuous data updates, making AI systems more responsive and current. This real-time integration is crucial for applications such as autonomous vehicles and dynamic decision-making systems.Community-Driven Open-Source Platforms:
Empowering communities through open-source initiatives will foster collaborative advancements. This collaborative trend is supported by platforms such as Gitcoin, which help fund and incentivize innovative open-source projects.
Structured Data
Table: Key AI Training Data Projects in Q1 2025
Project | Focus Area | Highlight | Impact | Source |
---|---|---|---|---|
DeepSeek R1 | Cost Efficiency | Launched in January using synthetic data | Reduced training costs, accessible AI | General Knowledge |
Gemini Robotics | Multimodal Data | Adaptive robotics using diverse data inputs | Improved real-world application | General Knowledge |
MIT Efficiency Models | Synthetic Data | Robust robotics agents with synthetic datasets | Scalable and cost-effective solutions | MIT News |
License-Token Tokenization | Data Monetization | Exploring tokenization of training data | Secure and transparent data transactions | License-token.com |
xAI Grok | Real-Time Data Updates | Continuous feeding of live data | Increased responsiveness in AI models | xAI |
Nvidia Frameworks | Infrastructure Efficiency | Energy-saving data center innovations | Reducing overall training costs | Nvidia Blog |
Bullet List: Key Trends in AI Training Data for Q1 2025
- Multimodal Data Integration: Combining text, images, and auditory data.
- Synthetic Data Surge: Adoption of computer-generated data as a substitute for scarce real data.
- Data Marketplaces Expansion: Platforms facilitating secure data access through tokenization.
- Open-Source Collaboration: Community-driven projects enhancing data access and fairness.
- Energy Efficiency Improvements: Innovations aimed at reducing the massive energy demands of data centers.
- Strict Regulatory Environment: Ongoing adaptations in response to new laws like the EU AI Act.
Future Outlook and Innovations Recap
The interplay of technological advancements and regulatory pressure is propelling the evolution of AI training data into a more resilient and versatile ecosystem. Looking forward, innovations in synthetic data and blockchain tokenization are expected to push the frontiers of what AI models can achieve, while energy-efficient solutions help address environmental and cost concerns. Startups and industry giants alike must be prepared to navigate these changes by embracing open-source principles and leveraging cutting-edge infrastructure.
In addition, platforms that foster community engagement, such as those discussed on Dev.to and Gitcoin, are playing a pivotal role in guiding the change. Their approaches not only tackle funding challenges but also nurture an innovation-driven ecosystem essential for sustainable growth.
Summary
In Q1 2025, the debate about whether the AI boom is stalling or surging is met with robust evidence of ongoing innovation. While challenges such as energy consumption, regulatory ambiguity, and data bias persist, the sector’s evolution is marked by exciting trends: multimodal integration, synthetic data adoption, blockchain tokenization of datasets, and increasingly ethical data practices.
This post has explored the landscape by first providing crucial background and context, followed by an analysis of core concepts such as synthetic data and decentralized data marketplaces. It also walked through practical applications—from robotics to adaptive models—and discussed current challenges and future outlooks. Drawing from sources like the EU AI Act, MIT News, and Nvidia Blog, the discussion confirms that AI training data is not stagnating but evolving intelligently.
For those following the latest in AI training data developments, the intersection of open-source collaboration and advanced tokenization is opening promising new paths for research, funding, and sustainable development.
Final Thoughts
The dynamic landscape of AI training data in Q1 2025 presents both a challenge and an opportunity. The integration of state-of-the-art synthetic data techniques with blockchain-based tokenization and ongoing regulatory evolution lays a foundation for a future where data is not only abundant and accessible but also ethically managed and cost-efficient. As more organizations invest in resilient data centers and open-source projects gain momentum, the AI boom is poised to surge into new realms of possibility.
Staying abreast of these trends is essential for developers, tech enthusiasts, and investors alike. Continue exploring AI Training Data News Q1 2025 for further insights and join the conversation by engaging with community platforms like those on Dev.to and Gitcoin. With a concerted effort toward sustainable development and innovation, the future of AI training data looks both promising and transformative.
Keywords: AI training data news Q1 2025, synthetic data, blockchain tokenization, multimodal training, open-source solution, regulatory framework, data marketplaces, energy efficient data centers, ethical AI data, adaptive robotics.
By understanding these trends and challenges today, industry leaders can better prepare for the next leap forward in AI training data—and ultimately, in AI innovation itself.