AI Innovation vs. Infrastructure Expenses: Are You Trapped in a Cost Spiral?
Your company has successfully launched an advanced AI-driven recommendation system to enhance customer interactions, increase engagement, and drive exceptional business outcomes — leading to well-deserved executive praise. However, after a few months, a stark reality emerges: cloud expenses are surging, GPU shortages are delaying model training, and data storage costs are mounting rapidly. This is the AI infrastructure cost challenge. While AI offers groundbreaking benefits, its infrastructure costs can quickly become overwhelming. Companies are investing billions into AI, yet many struggle to scale without incurring exorbitant expenses. With AI spending projected to exceed $300 billion by 2025 among top tech firms (The Times), businesses must reconsider their approach to AI infrastructure — balancing cutting-edge innovation with cost efficiency. The Growing Costs of AI Infrastructure 1. Computing Power: The High Cost of Intelligence AI models, particularly those utilizing deep learning, require substantial computational resources. The demand for GPUs and TPUs has driven up costs, and cloud providers charge premium rates for AI-specific workloads. Poor resource management, such as over-provisioning cloud services without assessing actual usage, can lead to excessive spending. 2. Data Storage and Management: The Hidden Expense AI relies on vast datasets, making storage and management critical yet costly. High-performance storage solutions, regulatory compliance, and redundancy measures contribute to mounting expenses. Many businesses underestimate the long-term costs of maintaining an AI-ready data ecosystem. 3. Infrastructure Scalability Challenges As AI adoption grows, infrastructure must scale accordingly. This includes acquiring additional hardware, expanding cloud capacity, and ensuring system reliability. Without a clear scaling strategy, organizations risk either over-investing in resources or underutilizing them, leading to inefficiencies and wasted expenditure. 4. Energy Consumption: A Sustainability Concern AI workloads are among the most energy-intensive computing tasks. Research from HBR indicates that training a single AI model can generate as much carbon emissions as five cars over their lifetime. AI-powered data centers consume up to 10 times more electricity than traditional IT operations. With sustainability becoming a key priority, businesses must explore energy-efficient AI architectures and renewable-powered data centers. 5. Talent Costs for AI Infrastructure Management Beyond hardware and cloud services, AI infrastructure requires skilled professionals for optimization and maintenance. AI engineers, data scientists, and IT specialists command high salaries, making recruitment both costly and competitive. Without a strong internal team, managing AI investments can become a significant challenge. Strategies to Manage AI Infrastructure Costs Balancing AI innovation with financial sustainability is essential for long-term success. Implementing cost-saving strategies can help businesses reduce expenditures while maintaining, or even enhancing, AI capabilities. 1. Adopt Cloud-Native and Hybrid Solutions A hybrid cloud approach that combines on-premises and cloud-based infrastructure can provide cost flexibility. Businesses can leverage cloud resources for high-demand AI tasks while using local infrastructure for routine operations, reducing overall expenses. Pay-as-you-go cloud models also help minimize upfront capital investments. 2. Optimize Compute Resource Utilization AI workloads don’t always require top-tier GPUs. Businesses can reduce costs by: Using lower-cost cloud instances for less-intensive training tasks. Implementing model pruning and quantization to lower computational demands. Running workloads during off-peak hours to take advantage of discounted cloud pricing. 3. Enhance AI Model Efficiency Optimizing AI models can significantly cut infrastructure costs. Techniques such as transfer learning, knowledge distillation, and federated learning help achieve high-performance outcomes with fewer resources. Selecting the right model architecture can also prevent unnecessary expenses. 4. Implement Smart Data Management Effective data management can reduce both storage and processing costs. Businesses should: Use tiered storage solutions, keeping frequently accessed data in high-performance storage and archiving less-used data in cost-effective solutions. Deduplicate and clean datasets to avoid redundant storage. Apply data compression techniques to minimize storage requirements. 5. Embrace Energy-Efficient AI Practices To lower energy costs and address sustainability concerns, organizations can: Optimize hardware usage with dynamic workload scheduling. Utilize renewable energy-powered data centers. Adopt energy-efficient AI chips designed for lower power consumption, such as NVIDIA Jetson and Google’s TPUs. 6. Invest in AI Cost Monitoring
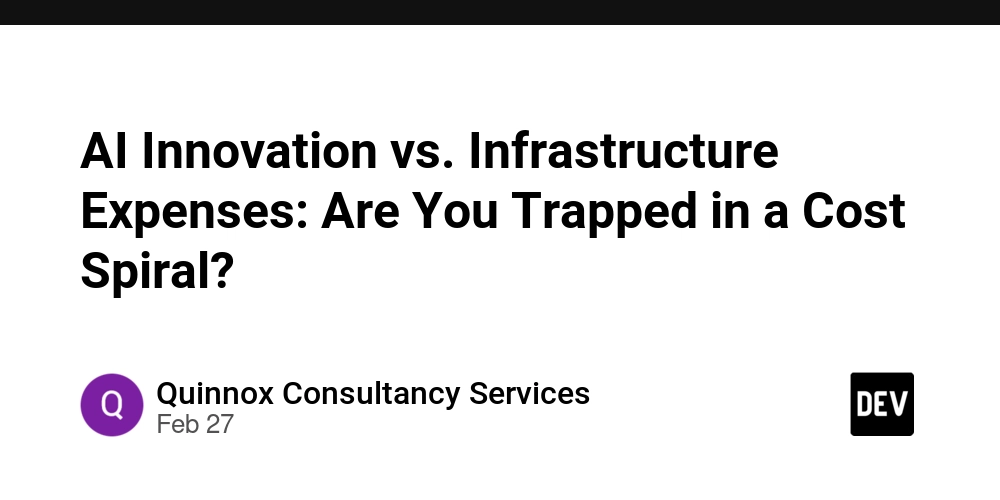
Your company has successfully launched an advanced AI-driven recommendation system to enhance customer interactions, increase engagement, and drive exceptional business outcomes — leading to well-deserved executive praise. However, after a few months, a stark reality emerges: cloud expenses are surging, GPU shortages are delaying model training, and data storage costs are mounting rapidly.
This is the AI infrastructure cost challenge. While AI offers groundbreaking benefits, its infrastructure costs can quickly become overwhelming. Companies are investing billions into AI, yet many struggle to scale without incurring exorbitant expenses. With AI spending projected to exceed $300 billion by 2025 among top tech firms (The Times), businesses must reconsider their approach to AI infrastructure — balancing cutting-edge innovation with cost efficiency.
The Growing Costs of AI Infrastructure
1. Computing Power: The High Cost of Intelligence
AI models, particularly those utilizing deep learning, require substantial computational resources. The demand for GPUs and TPUs has driven up costs, and cloud providers charge premium rates for AI-specific workloads. Poor resource management, such as over-provisioning cloud services without assessing actual usage, can lead to excessive spending.
2. Data Storage and Management: The Hidden Expense
AI relies on vast datasets, making storage and management critical yet costly. High-performance storage solutions, regulatory compliance, and redundancy measures contribute to mounting expenses. Many businesses underestimate the long-term costs of maintaining an AI-ready data ecosystem.
3. Infrastructure Scalability Challenges
As AI adoption grows, infrastructure must scale accordingly. This includes acquiring additional hardware, expanding cloud capacity, and ensuring system reliability. Without a clear scaling strategy, organizations risk either over-investing in resources or underutilizing them, leading to inefficiencies and wasted expenditure.
4. Energy Consumption: A Sustainability Concern
AI workloads are among the most energy-intensive computing tasks. Research from HBR indicates that training a single AI model can generate as much carbon emissions as five cars over their lifetime. AI-powered data centers consume up to 10 times more electricity than traditional IT operations. With sustainability becoming a key priority, businesses must explore energy-efficient AI architectures and renewable-powered data centers.
5. Talent Costs for AI Infrastructure Management
Beyond hardware and cloud services, AI infrastructure requires skilled professionals for optimization and maintenance. AI engineers, data scientists, and IT specialists command high salaries, making recruitment both costly and competitive. Without a strong internal team, managing AI investments can become a significant challenge.
Strategies to Manage AI Infrastructure Costs
Balancing AI innovation with financial sustainability is essential for long-term success. Implementing cost-saving strategies can help businesses reduce expenditures while maintaining, or even enhancing, AI capabilities.
1. Adopt Cloud-Native and Hybrid Solutions
A hybrid cloud approach that combines on-premises and cloud-based infrastructure can provide cost flexibility. Businesses can leverage cloud resources for high-demand AI tasks while using local infrastructure for routine operations, reducing overall expenses. Pay-as-you-go cloud models also help minimize upfront capital investments.
2. Optimize Compute Resource Utilization
AI workloads don’t always require top-tier GPUs. Businesses can reduce costs by:
Using lower-cost cloud instances for less-intensive training tasks.
Implementing model pruning and quantization to lower computational demands.
Running workloads during off-peak hours to take advantage of discounted cloud pricing.
3. Enhance AI Model Efficiency
Optimizing AI models can significantly cut infrastructure costs. Techniques such as transfer learning, knowledge distillation, and federated learning help achieve high-performance outcomes with fewer resources. Selecting the right model architecture can also prevent unnecessary expenses.
4. Implement Smart Data Management
Effective data management can reduce both storage and processing costs. Businesses should:
Use tiered storage solutions, keeping frequently accessed data in high-performance storage and archiving less-used data in cost-effective solutions.
Deduplicate and clean datasets to avoid redundant storage.
Apply data compression techniques to minimize storage requirements.
5. Embrace Energy-Efficient AI Practices
To lower energy costs and address sustainability concerns, organizations can:
Optimize hardware usage with dynamic workload scheduling.
Utilize renewable energy-powered data centers.
Adopt energy-efficient AI chips designed for lower power consumption, such as NVIDIA Jetson and Google’s TPUs.
6. Invest in AI Cost Monitoring and Governance
Lack of visibility into AI expenditures can result in inefficiencies. Companies should implement robust cost-monitoring tools to track infrastructure spending in real time. AI governance frameworks help enforce budget limits, improve accountability, and prevent unnecessary resource allocation.
_Stop wasting months on AI setup — start building today!
Join QAI Studio now and turn your AI vision into reality in days, not months!