Image Data Labeling Services: A Complete Guide for AI Developers
The success of artificial intelligence (AI) and machine learning (ML) models heavily relies on one key element: data. Specifically, labeled data.
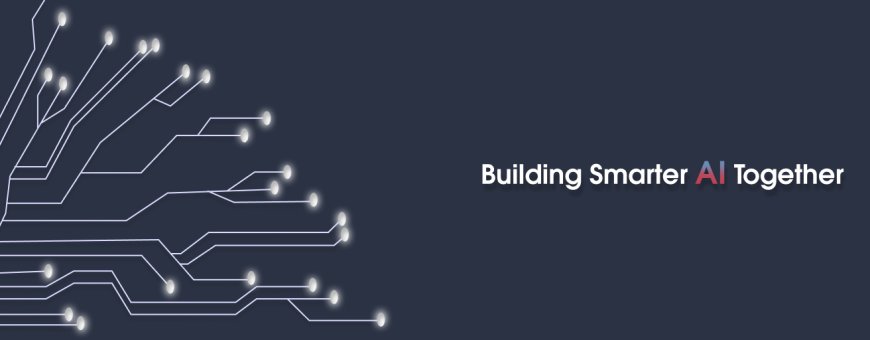
The success of artificial intelligence (AI) and machine learning (ML) models heavily relies on one key element: data. Specifically, labeled data. When it comes to computer vision tasks, image data labeling serves as the backbone of training highly accurate and efficient models. If you're an AI developer, ML engineer, or data scientist, you know just how important data quality is to your success, but finding the right services to label your images can often be daunting.
This blog will uncover everything you need to know about image data labeling services, from methods used and challenges faced to how to choose the right provider and what the future holds. Along the way, we'll highlight how companies like Macgence, a leader in providing tailor-made data solutions for AI/ML, can streamline this process for you.
What Is Image Data Labeling and Why Does It Matter?
Image data labeling is the process of annotating images with relevant metadata that computer vision models can understand and learn from. These labels are crucial in helping AI recognize objects, detect patterns, and perform tasks like image classification, object detection, and segmentation.
For example: If you’re building a self-driving car, labeled images allow the ML model to identify things like pedestrians, other vehicles, and traffic lights. The more accurate the annotations, the better your model performs.
Key reasons image data labeling matters:
- Improves AI Accuracy: Properly labeled data leads to more reliable predictions.
- Facilitates Model Training: The more detailed your annotations, the better context your model has for making decisions.
- Scalable AI Solutions: High-quality data builds a foundation for scalable machine learning applications.
Types of Image Annotation Techniques
Different AI projects demand different annotation techniques, depending on the end goal of the model. Here’s an overview of the most common types of image data labeling:
1. Bounding Boxes
Bounding boxes mark objects within an image using rectangular frames. This method is ideal for object detection tasks, like identifying vehicles or animals. However, while easy to implement, it may not always capture an object’s precise shape.
Example fields: Autonomous vehicles, retail inventory detection.
2. Semantic Segmentation
This technique involves labeling every pixel in an image with a class label. Semantic segmentation provides a high level of accuracy and is commonly used in applications where precision is crucial.
Example fields: Medical imaging, autonomous drones.
3. Polygon Annotation
Unlike bounding boxes, polygon annotation uses customized shapes to outline objects more accurately. This is especially useful for irregularly shaped items, such as logos or specific anatomical structures.
Example fields: Agriculture (crop monitoring), sports analytics.
4. Landmark Annotation
Landmark annotation identifies key points or specific locations in an image. For example, placing dots on a face to capture landmarks like eyes, nose, and mouth to train facial recognition models.
Example fields: Augmented reality, facial sentiment analysis.
5. 3D Cuboid Annotation
Used for 3D vision modeling, cuboid annotation adds depth to the traditional 2D bounding box by representing an object as a 3D structure.
Example fields: Robotics, autonomous cars.
6. Polyline Annotation
Polyline annotation is used for tasks requiring linear features, such as lane detection in self-driving cars or marking pipelines in industrial settings.
Example fields: Self-driving vehicles, infrastructure mapping.
Challenges in Image Data Labeling
Despite being essential, image data labeling comes with its own set of challenges:
1. Scalability
Dealing with millions of images is no easy feat. Creating high-quality annotations at scale requires robust infrastructure, experienced annotators, and seamless project management.
2. Quality Control
Maintaining annotation quality across large datasets can be tricky. Model performance depends heavily on data accuracy, so even minor inconsistencies in labeling can lead to significant errors.
3. Domain Expertise
Certain projects, like medical imaging or satellite image labeling, require specific domain knowledge. Recruiting skilled annotators with this expertise is often difficult and costly.
4. Cost and Time
Manually labeling large datasets requires significant financial and time investment. Without the right tools and experienced services, this process can quickly turn into a bottleneck.
How to Choose the Right Image Data Labeling Service
If you're considering outsourcing your image data labeling, it's essential to choose a service that fits your project requirements. Here’s what to look for:
1. Expertise and Customization
The service provider should have expertise in your specific domain and offer customizable solutions to meet your unique needs. For instance, Macgence specializes in creating bespoke data solutions for AI/ML and offers services tailored to various industries.
2. Scalability
Ensure the provider can handle datasets of all sizes, from a few thousand to millions of images, without compromising on quality or speed.
3. Quality Assurance
Look for services that implement multi-layer quality checks and provide annotations with high levels of accuracy.
4. Cost-Effectiveness
Compare costs among providers but don’t compromise quality for the sake of savings. A low-cost provider might save you money upfront but lead to costly errors later.
5. Tool Support
Check whether the labeling service supports the right tools and annotations for your project. Features like AI-assisted labeling can also speed up the process.
6. Turnaround Time
Timely delivery is crucial, especially in fast-paced AI/ML projects. Choose a service that aligns with your project timelines.
Real-World Applications of Image Data Labeling
Image labeling is not just a buzzword; it powers a variety of real-world AI solutions:
- Healthcare: Training AI to detect diseases from CT scans, X-rays, and MRIs.
- Retail: Enhancing inventory tracking and customer behavior analysis using computer vision.
- Agriculture: Using labeled imagery to monitor crop health and optimize yields.
- Autonomous Vehicles: Allowing cars to "see" and react to their surroundings.
- Security and Surveillance: Strengthening facial recognition and threat detection systems.
Macgence has supported numerous clients in powering such impactful applications by providing meticulously labeled datasets.
Future Trends in Image Data Labeling
The field of image data labeling is continuously evolving. Here’s a look at upcoming trends:
1. Automated Labeling with AI
AI-assisted labeling tools are becoming increasingly popular, combining automation with human validation to reduce errors and improve efficiency.
2. Real-Time Annotation
With advancements in tools and processing speed, real-time annotation is becoming viable, particularly for fast-moving industries like robotics and gaming.
3. Focus on Ethical AI
Initiatives are gaining traction for ensuring fairness and removing bias in training data, driven by demand for ethical AI development.
4. More Specialized Services
As the diversity of AI applications grows, service providers will offer increasingly niche expertise, such as aerial imagery annotation or medical labeling.
Key Takeaways for Image Data Labeling
Image data labeling is at the heart of creating high-performing AI/ML models. While it presents challenges like scalability and quality control, outsourcing to reliable services like Macgence can simplify the process and deliver exceptional results tailored to your project.
By choosing the right service provider, you can focus on building your AI solution while ensuring that your data preparation is in expert hands.
Looking to elevate your AI/ML development? Contact Macgence today to explore our data labeling services and get started on building intelligent, data-powered solutions.
FAQs
What is an image annotation tool?
An image annotation tool is software that enables annotators to add metadata, such as bounding boxes or segmentation masks, to images for use in AI/ML training.
How much time does image labeling typically take?
The time required depends on factors like dataset size, annotation type, and project complexity. Outsourcing can significantly reduce this time.
Is outsourcing image labeling cost-effective?
Yes, especially for large-scale projects. Reliable service providers ensure accuracy, scalability, and domain expertise, saving time and costs in the long run.
Why choose Macgence for data labeling services?
Macgence offers tailored solutions, domain expertise, and a strong commitment to quality, making it a trusted partner for AI developers and businesses.